filmov
tv
Geekbench AI Shows Why AI Benchmarking is HARD!
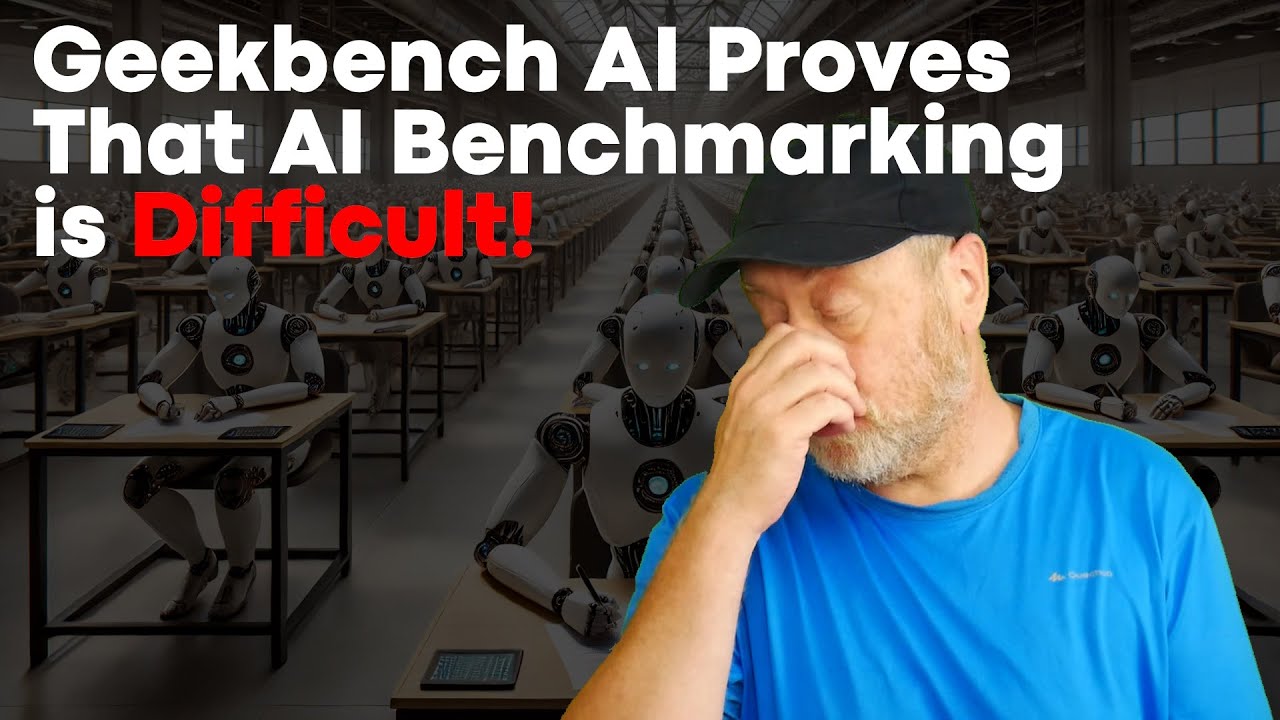
Показать описание
AI and Machine Learning are everywhere, from Large Language Models to neural networks that blur out the background on our phones. It is more important that ever to be able to test the speed and performance of our AI enabled devices. To that end Primate Labs has released Geekbench AI v1.0. However using it we quickly discover that testing AI performance is HARD!
---
#garyexplains
---
#garyexplains
Geekbench AI Shows Why AI Benchmarking is HARD!
Breaking Down Geekbench AI 1.0 with John Poole
'AI Performance EXPERT Reveals Geekbench AI Secrets! #shorts #GeekbenchAI #AISecrets'
Geekbench AI is now available for smartphones 📱✨ #shorts
Primate Labs Geekbench AI: New Benchmarking Tool Testing Machine Learning and AI Workload Power
AMD Ryzen AI Max 390: Geekbench Leak!
AI Benchmark for Measuring Machine Learning Performance
Zen 5 Laptops are HERE - Ryzen Ai 300 Series Explained
RISC-V 2024 Update: RISE, AI Accelerators & More
LEAKED BENCHMARKS!! M4 MacBook Vs Snapdragon X Elite Co-Pilot Ai Laptops
Running AI on NVIDIA A10 GPUs in Oracle Cloud, with Nicolas Savides / Nvidia
Google Pixel 9 Pro Preview: AI that ACTUALLY WORKS?
The ACTUAL Difference Between Intel and AMD
6,373 AI Benchmark From BIOS to Benchmark
Galaxy AI S24 Gratis, Tapi... 🤔 #shorts
Breaking Down Intel's Strategy For Core Ultra 200S
The New Google Gemini AI Features!
Vivo X200 Pro Vs Galaxy S24 Ultra Vs iPhone 16 Pro Max Camera Comparison
Windows 11 on ARM is rough - Acer Swift 14 AI
Best Laptop CPU? AMD Ryzen AI 9 HX 370 vs Intel Core Ultra 9 185H
M4 Pro M4 Max Benchmarks and predictions
Samsung Galaxy S24 FE Review: A Cheaper Way to Get Galaxy AI
This Mini PC Has The Most Powerful 890M iGPU Ryzen Ai 9 HX 370 First Look
AMD Ryzen AI 9 HX 375 Strix APU Benchmarked in Geekbench, Scoring Over 15,000 Points in Multi Score
Комментарии