filmov
tv
SVM Kernal- Polynomial And RBF Implementation Using Sklearn- Machine Learning
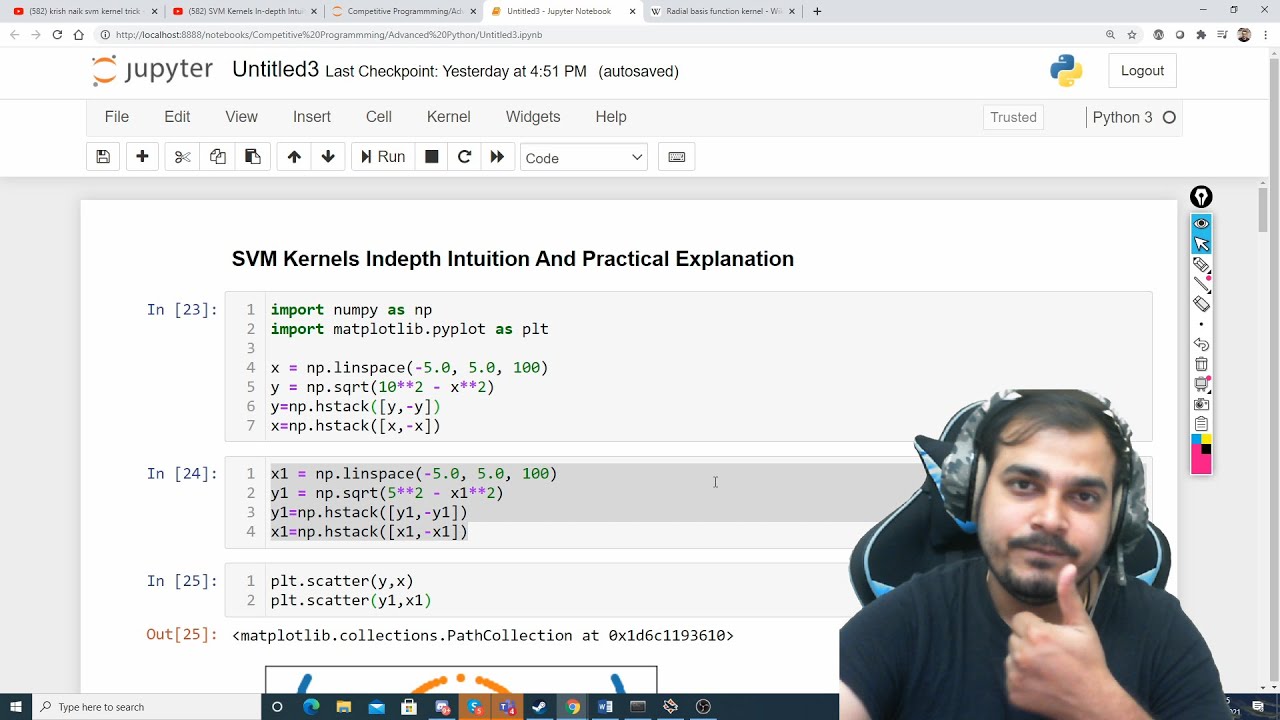
Показать описание
Join Affordable ML and DL Course starting on April 10th
SVM Tutorials
All Playlist In My channel
Please donate if you want to support the channel through GPay UPID,
Please join as a member in my channel to get additional benefits like materials in Data Science, live streaming for Members and many more
Please do subscribe my other channel too
Connect with me here:
SVM Tutorials
All Playlist In My channel
Please donate if you want to support the channel through GPay UPID,
Please join as a member in my channel to get additional benefits like materials in Data Science, live streaming for Members and many more
Please do subscribe my other channel too
Connect with me here:
The Kernel Trick in Support Vector Machine (SVM)
SVM Kernal- Polynomial And RBF Implementation Using Sklearn- Machine Learning
Support Vector Machines Part 3: The Radial (RBF) Kernel (Part 3 of 3)
SVM with polynomial kernel visualization (HD)
Support Vector Machines Part 1 (of 3): Main Ideas!!!
Support Vector Machine (SVM) in 2 minutes
Support Vector Machines Part 2: The Polynomial Kernel (Part 2 of 3)
106 RBF Kernel
107 RBF Kernel
Types of Kernel in SVM | Kernels in Support vector machine in Machne Learning by Mahesh Huddar
What is Kernel Trick in Support Vector Machine | Kernel Trick in SVM Machine Learning Mahesh Huddar
Radial Basis Function Kernel : Data Science Concepts
SVM Kernels : Data Science Concepts
A simple Numerical Example of RBF Kernel with SVM in Machine Learning
104 Polynomial Kernel
The Kernel Trick - THE MATH YOU SHOULD KNOW!
SVM Kernels In-depth Intuition- Polynomial Kernels Part 3 | Machine Learning Data Science
Support Vector Machines Implementation Python | Hyperplane | SVM Kernels | Linear | Polynomial | RBF
Support Vector Machines: All you need to know!
SVM (polynomial kernel) - Influence of the degree
Kernel Trick
26-a LFD: SVM: Choosing your polynomial or Gaussian RBF kernel.
Part 25-Support Vector Machines, the Kernel trick
103 Polynomial Kernel
Комментарии