filmov
tv
Inverse Transform Sampling - VISUALLY EXPLAINED with EXAMPLES!
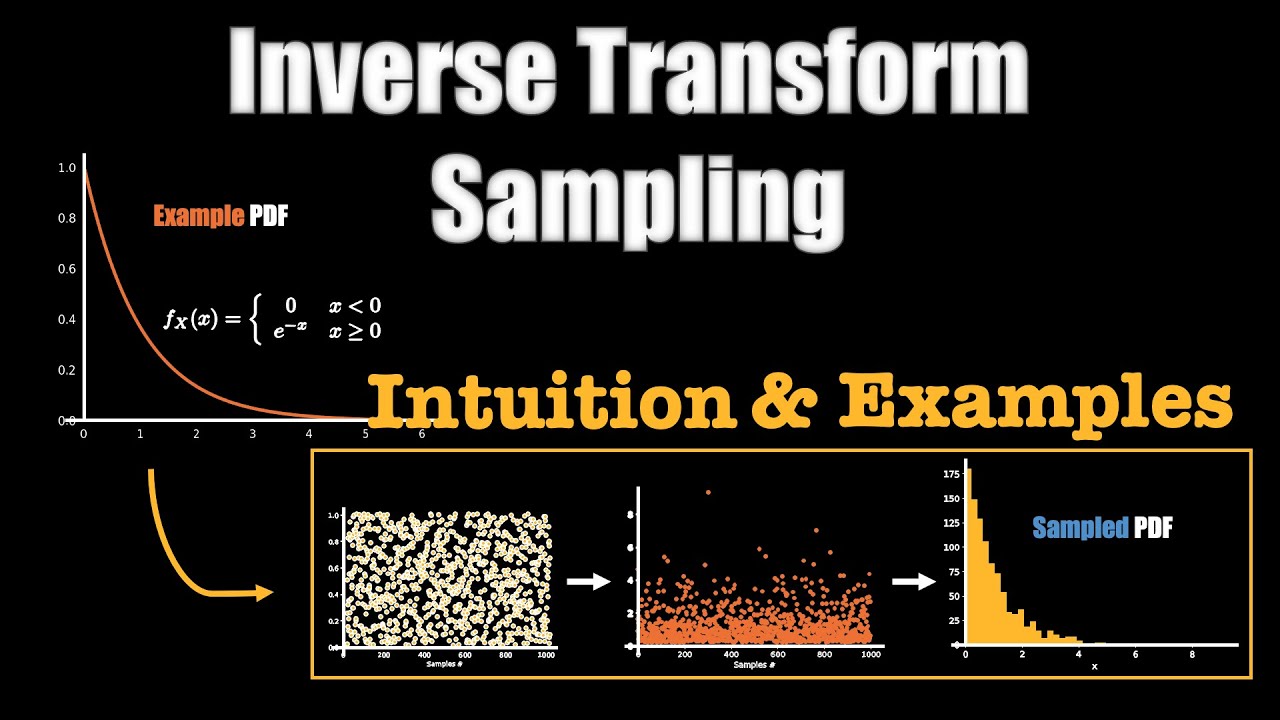
Показать описание
This tutorial explains the Inverse Transform Sampling using a simple example. The proof of why the algorithm/transform works is also explained.
#sampling
#statistics
#distributions
#sampling
#statistics
#distributions
Inverse Transform Sampling - VISUALLY EXPLAINED with EXAMPLES!
Inverse Transform Sampling : Data Science Concepts
Inverse Transform Sampling | How a computer can choose random numbers from a given distribution.
Every Random Variable is a Transformation of U[0,1] (Inverse Transform Sampling)
Inverse Transform Sampling | Triangular Distribution
PDF Sampling: Inverse transform sampling (case: Plummer model)
Inverse Probability Transform & Proof (Sampling technique)
Making an animated gif for Wikipedia using Python (tutorial) - Inverse Transform Sampling
Simulating Continuous Distributions via Inverse Transform Sampling
Google Data Science interview Question | Inverse Transform Sampling | Truncated Normal | Python
Sine Curve and the Unit Circle
Lect 1 : Inverse transformation method using Rstudio
Simulating the Pareto Distribution with Inverse Transform Sampling
Random numbers: Rejection Method 01: The Idea
What is Monte Carlo simulation in statistics? Inverse transform m |Class 14,CS1-Actuarial Statistics
Accept-Reject Sampling : Data Science Concepts
Generating the Random Numbers Using the Inverse Transform Method
Monte Carlo Methods - VISUALLY EXPLAINED!
Using The Inverse CDF Method to Simulate Random Variables
AST9240 - Inversion Sampling
Rejection Sampling - VISUALLY EXPLAINED with EXAMPLES!
inverse transform technique
Code Review: Inverse transform sampling (2 Solutions!!)
Inverse CDF method
Комментарии