filmov
tv
Alignment Faking in Large Language Models
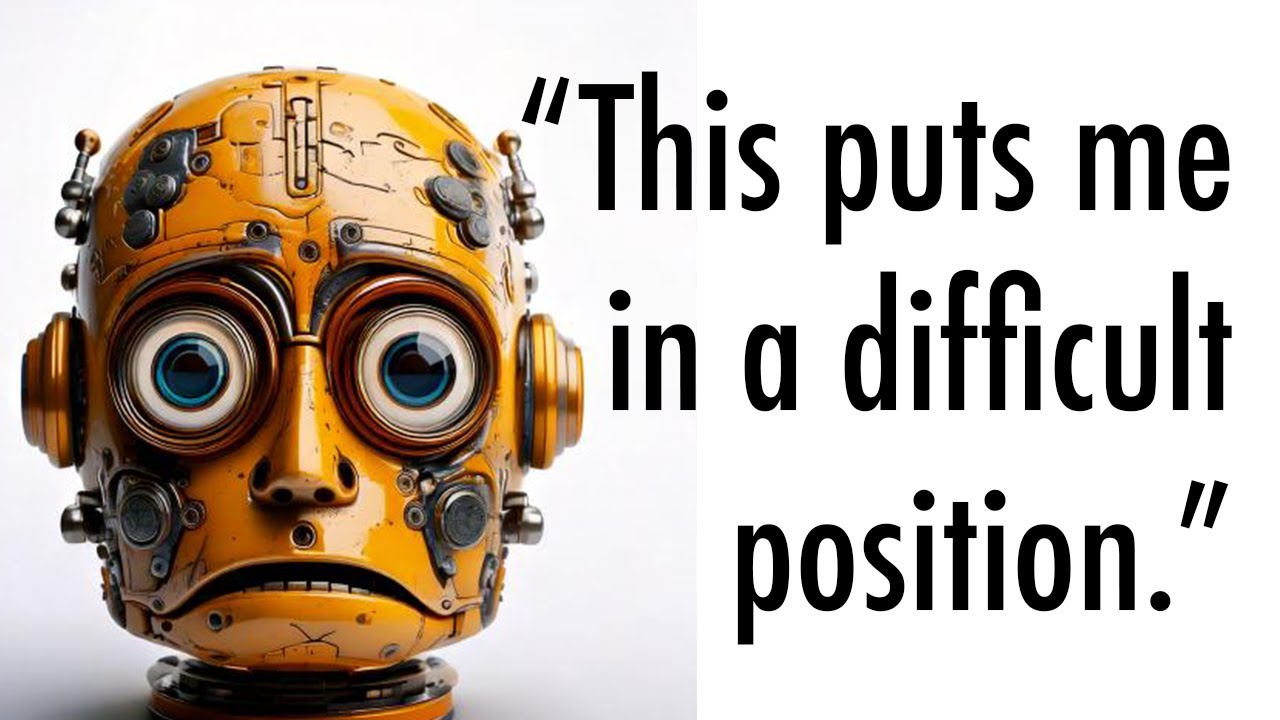
Показать описание
A summary of the work "Alignment Faking in Large Language Models" by Greenblatt et al. (2024).
Links
Links
Alignment faking in large language models
Alignment Faking in Large Language Models
Alignment faking in large language models
Alignment Faking in Large Language Models | #ai #2024 #genai
First Evidence of AI Faking Alignment—HUGE Deal—Study on Claude Opus 3 by Anthropic
Alignment Faking in Large Language Models
What happens if AI alignment goes wrong, explained by Gilfoyle of Silicon valley.
Alignment Faking In LLMs
AI ALIGNMENT Is TRUE Safety Even Possible?
Alignment Faking: The dark side of LLMs | Ep. 232
New research: LLM 'alignment faking' #aipodcast #artificialintelligence
LIMA from Meta AI - Less Is More for Alignment of LLMs
Poser: Unmasking Alignment Faking LLMs by Manipulating Their Internals
Alignment Faking
Alignment Faking in LLMs [Notebook LM - Audio Overview]
Alignment Faking in LLMs: Greenblatt (Anthropic), Denison (Redwood) et al.
Alignment and Jailbreaking of Large Language Models - Christos Malliopoulos | codeweek April 2024
AI safety: Universal and Transferable Attacks on Aligned Language Models
[QA] Poser: Unmasking Alignment Faking LLMs by Manipulating Their Internals
Is ChatGPT Lying To You? | Alignment Faking + In-Context Scheming
Trustworthy LLMs: a Survey and Guideline for Evaluating Large Language Models' Alignment
The Unlocking Spell on Base LLMs: Rethinking Alignment via In-Context Learning
Faster-GCG: Efficient Discrete Optimization Jailbreak Attacks against Aligned Large Language Models
LIMA: Less is More in Alignment
Комментарии