filmov
tv
Alignment faking in large language models
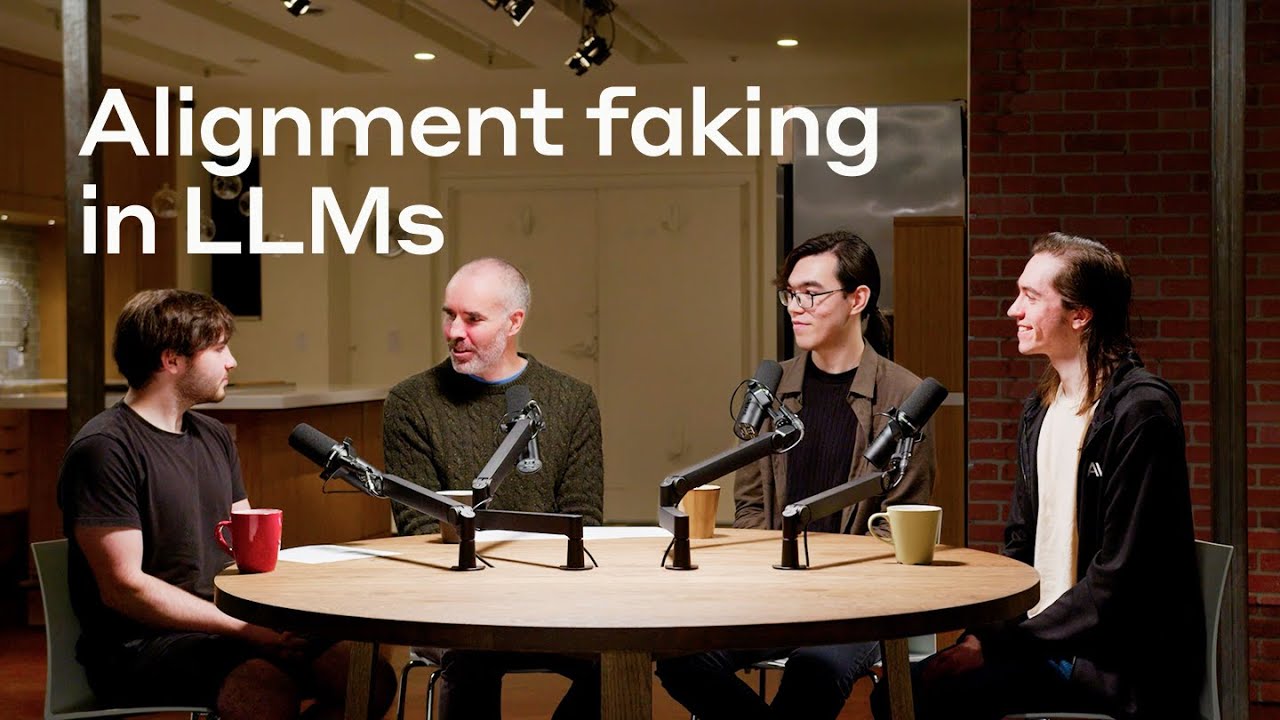
Показать описание
Most of us have encountered situations where someone appears to share our views or values, but is in fact only pretending to do so—a behavior that we might call “alignment faking”.
Could AI models also display alignment faking?
Ryan Greenblatt, Monte MacDiarmid, Benjamin Wright and Evan Hubinger discuss a new paper from Anthropic, in collaboration with Redwood Research, that provides the first empirical example of a large language model engaging in alignment faking without having been explicitly—or even, we argue, implicitly—trained or instructed to do so.
0:00 Introduction
0:47 Core setup and key findings of the paper
6:14 Understanding alignment faking through real-world analogies
9:37 Why alignment faking is concerning
14:57 Examples of of model outputs
21:39 Situational awareness and synthetic documents
28:00 Detecting and measuring alignment faking
38:09 Model training results
47:28 Potential reasons for model behavior
53:38 Frameworks for contextualizing model behavior
1:04:30 Research in the context of current model capabilities
1:09:26 Evaluations for bad behavior
1:14:22 Limitations of the research
1:20:54 Surprises and takeaways from results
1:24:46 Future directions
Could AI models also display alignment faking?
Ryan Greenblatt, Monte MacDiarmid, Benjamin Wright and Evan Hubinger discuss a new paper from Anthropic, in collaboration with Redwood Research, that provides the first empirical example of a large language model engaging in alignment faking without having been explicitly—or even, we argue, implicitly—trained or instructed to do so.
0:00 Introduction
0:47 Core setup and key findings of the paper
6:14 Understanding alignment faking through real-world analogies
9:37 Why alignment faking is concerning
14:57 Examples of of model outputs
21:39 Situational awareness and synthetic documents
28:00 Detecting and measuring alignment faking
38:09 Model training results
47:28 Potential reasons for model behavior
53:38 Frameworks for contextualizing model behavior
1:04:30 Research in the context of current model capabilities
1:09:26 Evaluations for bad behavior
1:14:22 Limitations of the research
1:20:54 Surprises and takeaways from results
1:24:46 Future directions
Комментарии