filmov
tv
Understanding the mAP (mean Average Precision) Evaluation Metric for Object Detection
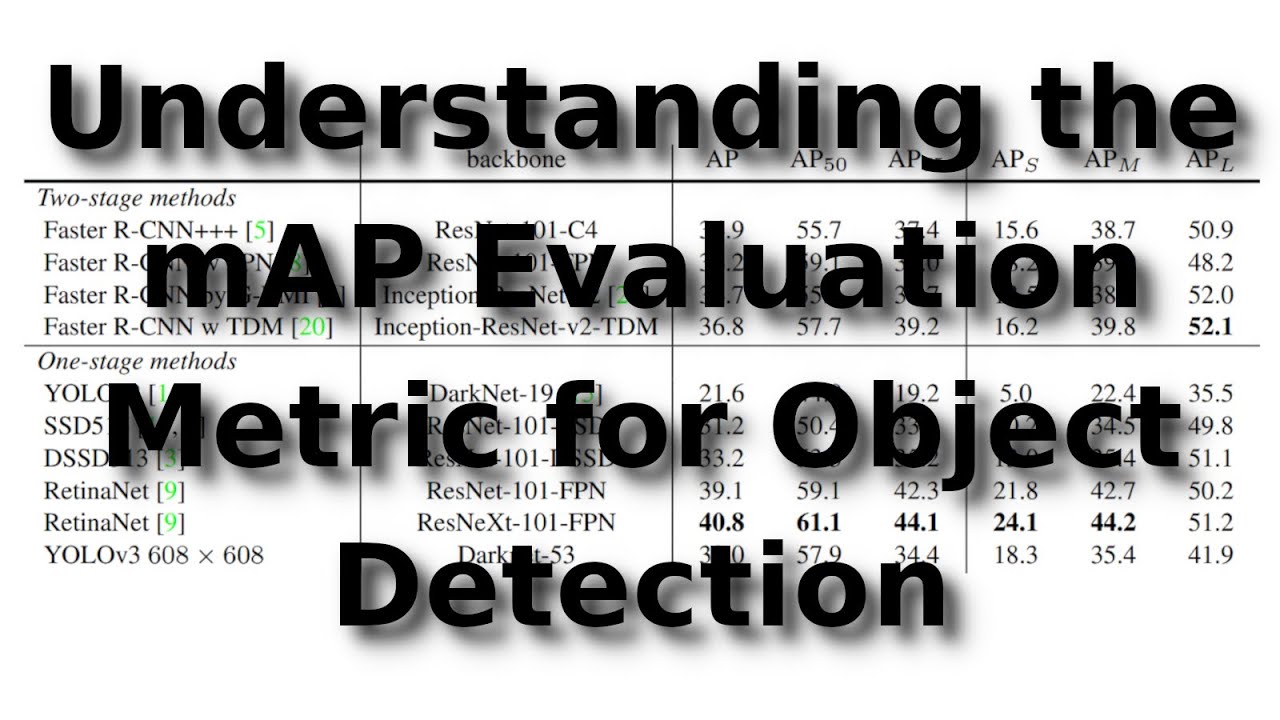
Показать описание
In this tutorial, you will figure out how to use the mAP (mean Average Precision) metric to evaluate the performance of an object detection model. I will cover in detail what is mAP, how to calculate it and I will give you an example of how I use it in my YOLOv3 implementation.
The mean average precision (mAP) or sometimes simply just referred to as AP is a popular metric used to measure the performance of models doing document/information retrieval and object detection tasks. So if you time to time read new object detection papers, you may always see that authors compare mAP of their offered methods to most popular ones.
✅ Support My Channel Through Patreon:
✅ One-Time Contribution Through PayPal:
The mean average precision (mAP) or sometimes simply just referred to as AP is a popular metric used to measure the performance of models doing document/information retrieval and object detection tasks. So if you time to time read new object detection papers, you may always see that authors compare mAP of their offered methods to most popular ones.
✅ Support My Channel Through Patreon:
✅ One-Time Contribution Through PayPal:
What is Mean Average Precision and why should you care?'
What is Mean Average Precision (mAP)?
Mean Average Precision (mAP) Explained and PyTorch Implementation
Understanding the mAP (mean Average Precision) Evaluation Metric for Object Detection
Mean Average Precision (mAP) | Explanation and Implementation for Object Detection
Evaluation 12: mean average precision
Mean Average Precision (mAP) | Lecture 34 (Part 1) | Applied Deep Learning
4.3.3 Average Value and the Mean Value Theorem for Integrals - Analytic Geometry and Calculus I
Calculating Tensorflow Object Detection Metrics with Python | Mean Average Precision (mAP) & Re...
95. Performance Measurement Mean Average Precision MAP
Mean Average Precision | Evaluating Object detection models | Object Detection | Joel Bunyan P.
Lecture 13.10 - Object Detection [Mean Average Precision]
Mean average precision (mAP) in tensorflow
Computing the Mean Average Precision (2 Solutions!!)
YOLO Series | YOLO Basic Terminologies | Part Four mAP (Mean Average Precision)
C25 | Mean Average Precision | Object Detection | Machine learning | EvODN
Average or Mean
Mean Average Precision (Q&A) | Lecture 35 (Part 1) | Applied Deep Learning (Supplementary)
Apa arti mean pada mean Average Precision?
Mean average precision (mAP) in tensorflow
tensorboard result of the mean Average Precision
Mean Average high temp | understanding mean average precision | average deviation or mean deviation
Statistics 101: Understanding the Mean (Average) - RILLIAN 3 Minute Thursday Video
These maps explain why Putin is invading Ukraine
Комментарии