filmov
tv
Plamen Turkedjiev: Least squares regression Monte Carlo for approximating BSDES and semilinear PDES
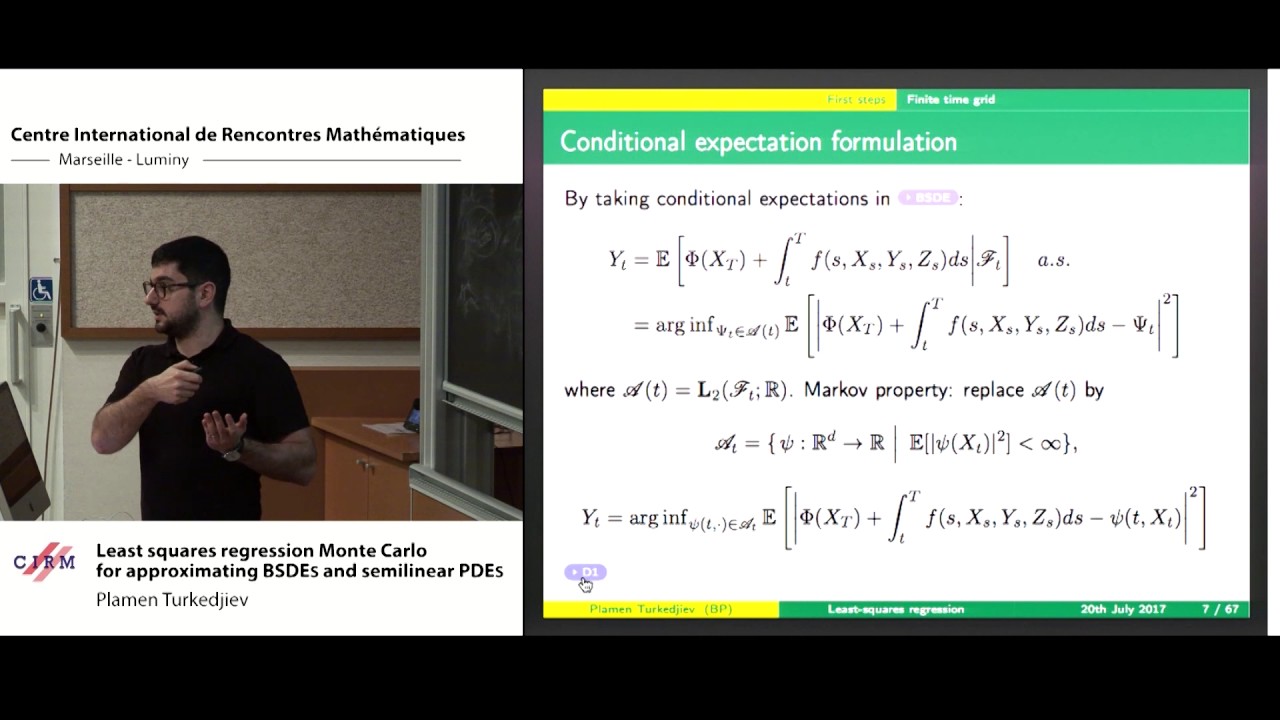
Показать описание
Abstract: In this lecture, we shall discuss the key steps involved in the use of least squares regression for approximating the solution to BSDEs. This includes how to obtain explicit error estimates, and how these error estimates can be used to tune the parameters of the numerical scheme based on complexity considerations.
The algorithms are based on a two stage approximation process. Firstly, a suitable discrete time process is chosen to approximate the of the continuous time solution of the BSDE. The nodes of the discrete time processes can be expressed as conditional expectations. As we shall demonstrate, the choice of discrete time process is very important, as its properties will impact the performance of the overall numerical scheme. In the second stage, the conditional expectation is approximated in functional form using least squares regression on synthetically generated data k Monte Carlo simulations drawn from a suitable probability distribution. A key feature of the regression step is that the explanatory variables are built on a user chosen finite dimensional linear space of functions, which the user specifies by setting basis functions. The choice of basis functions is made on the hypothesis that it contains the solution, so regularity and boundedness assumptions are used in its construction. The impact of the choice of the basis functions is exposed in error estimates.
In addition to the choice of discrete time approximation and the basis functions, the Markovian structure of the problem gives significant additional freedom with regards to the Monte Carlo simulations. We demonstrate how to use this additional freedom to develop generic stratified sampling approaches that are independent of the underlying transition density function. Moreover, we demonstrate how to leverage the stratification method to develop a HPC algorithm for implementation on GPUs.
Thanks to the Feynmann-Kac relation between the the solution of a BSDE and its associated semilinear PDE, the approximation of the BSDE can be directly used to approximate the solution of the PDE. Moreover, the smoothness properties of the PDE play a crucial role in the selection of the hypothesis space of regressions functions, so this relationship is vitally important for the numerical scheme.
We conclude with some draw backs of the regression approach, notably the curse of dimensionality.
Recording during the CEMRACS Summer school 2017 "Numerical Methods for Stochastic Models: Control, Uncertainty Quantification, Mean-field " the July 20, 2017 at the Centre International de Rencontres Mathématiques (Marseille, France)
Filmmaker: Guillaume Hennenfent
- Chapter markers and keywords to watch the parts of your choice in the video
- Videos enriched with abstracts, bibliographies, Mathematics Subject Classification
- Multi-criteria search by author, title, tags, mathematical area
The algorithms are based on a two stage approximation process. Firstly, a suitable discrete time process is chosen to approximate the of the continuous time solution of the BSDE. The nodes of the discrete time processes can be expressed as conditional expectations. As we shall demonstrate, the choice of discrete time process is very important, as its properties will impact the performance of the overall numerical scheme. In the second stage, the conditional expectation is approximated in functional form using least squares regression on synthetically generated data k Monte Carlo simulations drawn from a suitable probability distribution. A key feature of the regression step is that the explanatory variables are built on a user chosen finite dimensional linear space of functions, which the user specifies by setting basis functions. The choice of basis functions is made on the hypothesis that it contains the solution, so regularity and boundedness assumptions are used in its construction. The impact of the choice of the basis functions is exposed in error estimates.
In addition to the choice of discrete time approximation and the basis functions, the Markovian structure of the problem gives significant additional freedom with regards to the Monte Carlo simulations. We demonstrate how to use this additional freedom to develop generic stratified sampling approaches that are independent of the underlying transition density function. Moreover, we demonstrate how to leverage the stratification method to develop a HPC algorithm for implementation on GPUs.
Thanks to the Feynmann-Kac relation between the the solution of a BSDE and its associated semilinear PDE, the approximation of the BSDE can be directly used to approximate the solution of the PDE. Moreover, the smoothness properties of the PDE play a crucial role in the selection of the hypothesis space of regressions functions, so this relationship is vitally important for the numerical scheme.
We conclude with some draw backs of the regression approach, notably the curse of dimensionality.
Recording during the CEMRACS Summer school 2017 "Numerical Methods for Stochastic Models: Control, Uncertainty Quantification, Mean-field " the July 20, 2017 at the Centre International de Rencontres Mathématiques (Marseille, France)
Filmmaker: Guillaume Hennenfent
- Chapter markers and keywords to watch the parts of your choice in the video
- Videos enriched with abstracts, bibliographies, Mathematics Subject Classification
- Multi-criteria search by author, title, tags, mathematical area