filmov
tv
Exploring Multi-Agent AI and AutoGen with Chi Wang
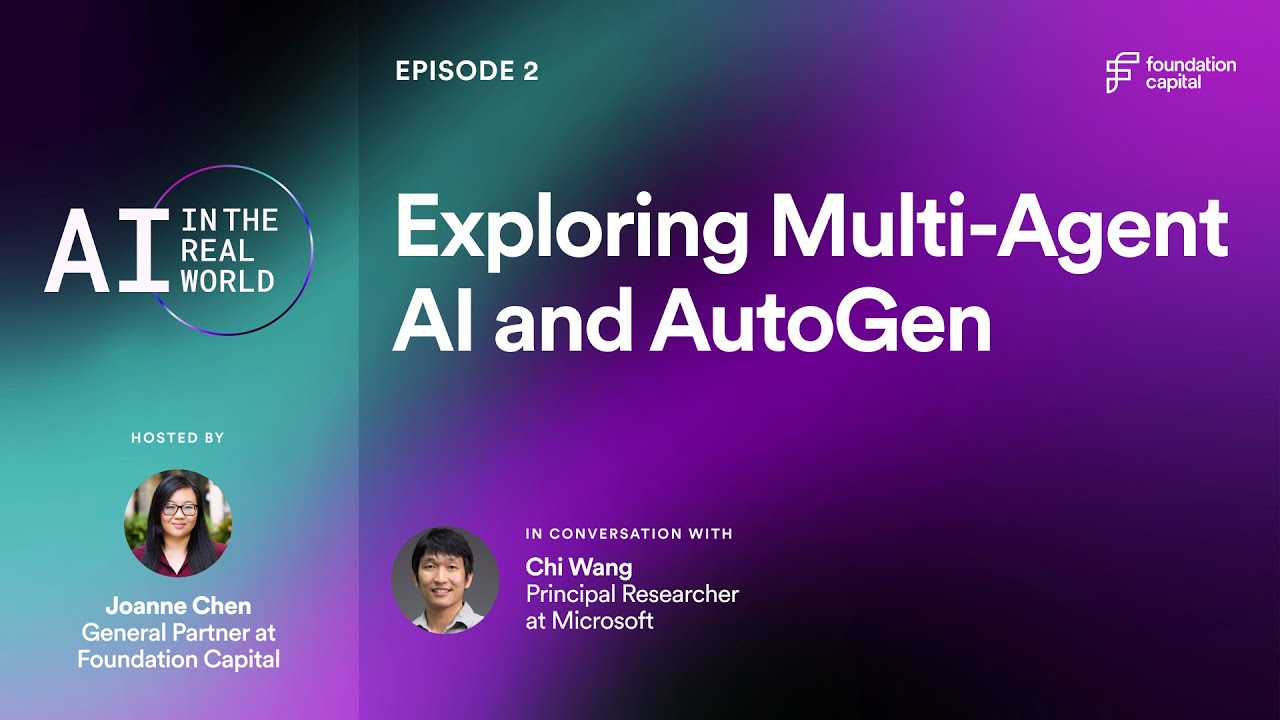
Показать описание
In this episode, I’m joined by Chi Wang, a principal researcher at Microsoft and the creator of AutoGen, a open-source framework that allows developers to combine LLMs, tools, and human input to build multi-agent AI systems.
By enabling AI agents to collaborate, learn from each other, and contribute their unique skills, AutoGen is unlocking a new frontier of AI capabilities. It's quickly gained traction among both academics and enterprises and is currently powering a wide range of use cases, including synthetic data generation, code generation, and pharamaceutical data science.
In our conversation, Chi breaks down the core concepts behind multi-agent AI, the pros and cons of multi-agent architectures, and the real-world use cases enabled by AutoGen. He also shares some of the open research challenges he's tackling, his perspective on the future of AI, and what excites him most about where the field is headed.
(00:00) Intro
(01:27) Chi's background and early interest in AI
(05:42) Defining agents and their core capabilities
(08:13) Pros and cons of multi-agent systems
(11:23) Multi-agent architectures and the "Society of Mind" theory
(14:36) Real-world use cases enabled by multi-agent systems
(16:45) The backstory and genesis of AutoGen
(19:43) How AutoGen's architecture leverages language models, tools, and human input
(23:13) More examples of AutoGen's diverse applications
(29:23) How AutoGen is being used in enterprises and production
(32:42) Advice for AI builders focusing on the enterprise
(40:24) What's next for AutoGen and open research challenges
(47:17) Resource recommendations for AI builders
(48:09) What excites Chi most about the future of AI
By enabling AI agents to collaborate, learn from each other, and contribute their unique skills, AutoGen is unlocking a new frontier of AI capabilities. It's quickly gained traction among both academics and enterprises and is currently powering a wide range of use cases, including synthetic data generation, code generation, and pharamaceutical data science.
In our conversation, Chi breaks down the core concepts behind multi-agent AI, the pros and cons of multi-agent architectures, and the real-world use cases enabled by AutoGen. He also shares some of the open research challenges he's tackling, his perspective on the future of AI, and what excites him most about where the field is headed.
(00:00) Intro
(01:27) Chi's background and early interest in AI
(05:42) Defining agents and their core capabilities
(08:13) Pros and cons of multi-agent systems
(11:23) Multi-agent architectures and the "Society of Mind" theory
(14:36) Real-world use cases enabled by multi-agent systems
(16:45) The backstory and genesis of AutoGen
(19:43) How AutoGen's architecture leverages language models, tools, and human input
(23:13) More examples of AutoGen's diverse applications
(29:23) How AutoGen is being used in enterprises and production
(32:42) Advice for AI builders focusing on the enterprise
(40:24) What's next for AutoGen and open research challenges
(47:17) Resource recommendations for AI builders
(48:09) What excites Chi most about the future of AI
Комментарии