filmov
tv
'Causal Inference and Causal Discovery in Climate Science'
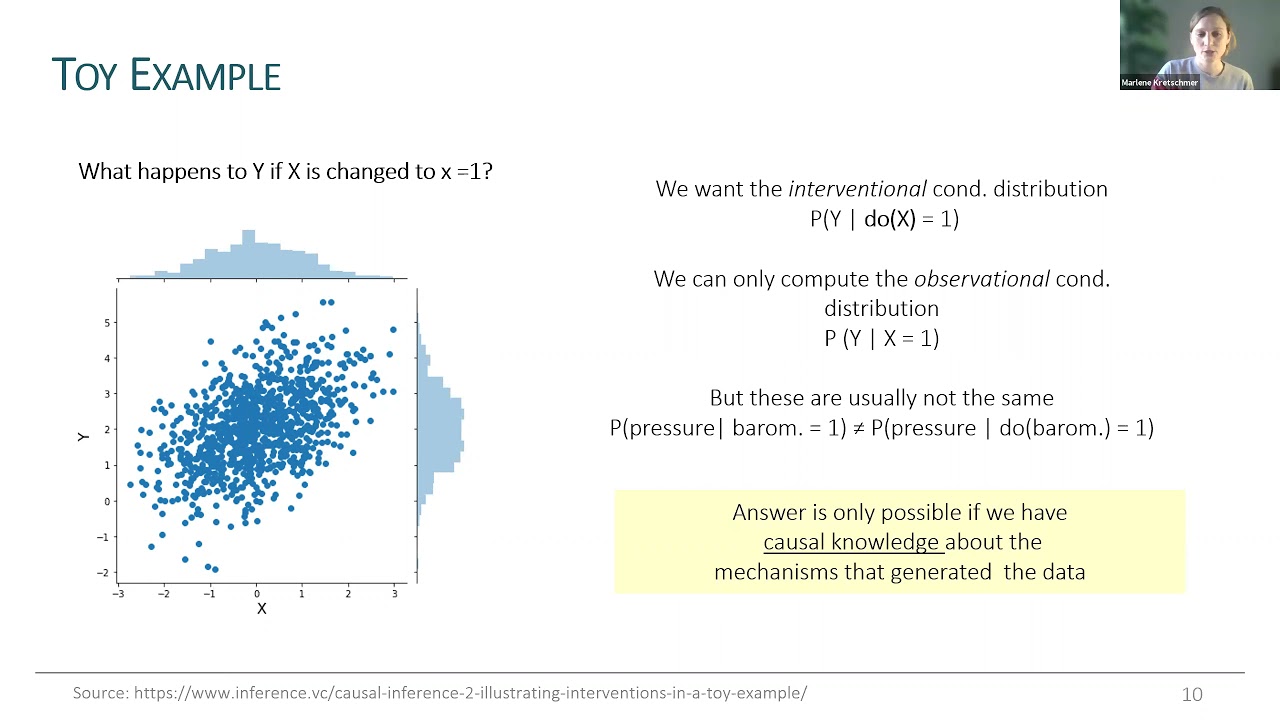
Показать описание
Speaker: Marlene Kretschmer is Postdoc in the Department of Meteorology at the University of Reading.
Abstract:Teleconnections are sources of predictability for regional weather and climate but the relative contributions of different teleconnections to regional anomalies are usually not understood and often highly contested. To close this important knowledge gap, progress is needed in analysing and quantifying teleconnection pathways. Here we argue for the use of causal inference theory and causal networks to overcome these challenges. We describe some of the key concepts of this theory and illustrate them with concrete examples of atmospheric teleconnections. We further discuss the particular challenges and advantages these imply for climate science.
Abstract:Teleconnections are sources of predictability for regional weather and climate but the relative contributions of different teleconnections to regional anomalies are usually not understood and often highly contested. To close this important knowledge gap, progress is needed in analysing and quantifying teleconnection pathways. Here we argue for the use of causal inference theory and causal networks to overcome these challenges. We describe some of the key concepts of this theory and illustrate them with concrete examples of atmospheric teleconnections. We further discuss the particular challenges and advantages these imply for climate science.
Causal Inference - EXPLAINED!
'Causal Inference and Causal Discovery in Climate Science'
Book Review - Causal Inference and Discovery in Python
Joris Mooij: Joint Causal Inference: A Unifying Perspective on Causal Discovery
Causal discovery tutorial
Causal Discovery | Inferring causality from observational data
Causality and (Graph) Neural Networks
Causal discovery in Python - Aleksander Molak, Lingaro | GHOST Day: AMLC 2022
10.4 - The PC Algorithm for Causal Discovery
An Overview of Causal Discovery
Causal Discovery
Causal inference and discovery
14. Causal Inference, Part 1
10 - Causal Discovery from Observational Data
10.2 - Assumptions for Independence-Based Causal Discovery
Causal inferences course
11.8 - Causal Discovery from Interventions in Other Settings
An introduction to Causal Inference with Python – making accurate estimates of cause and effect from...
How to learn Causal Inference with #python #dataanalysis #datascience
Causal Inference - Frederick Eberhardt - 6/7/2019
Yujia Zheng on causal-learn library: Causal discovery in Python | PyWhy Causality in Practice Talk
Causal Discovery vs Causal Inference Using Python
10.7 - Nonlinear Additive Noise Setting for Causal Discovery
Causal discovery from data: the problem with “unfaithful” structural models
Комментарии