filmov
tv
Naïve Bayes Classifier - Fun and Easy Machine Learning
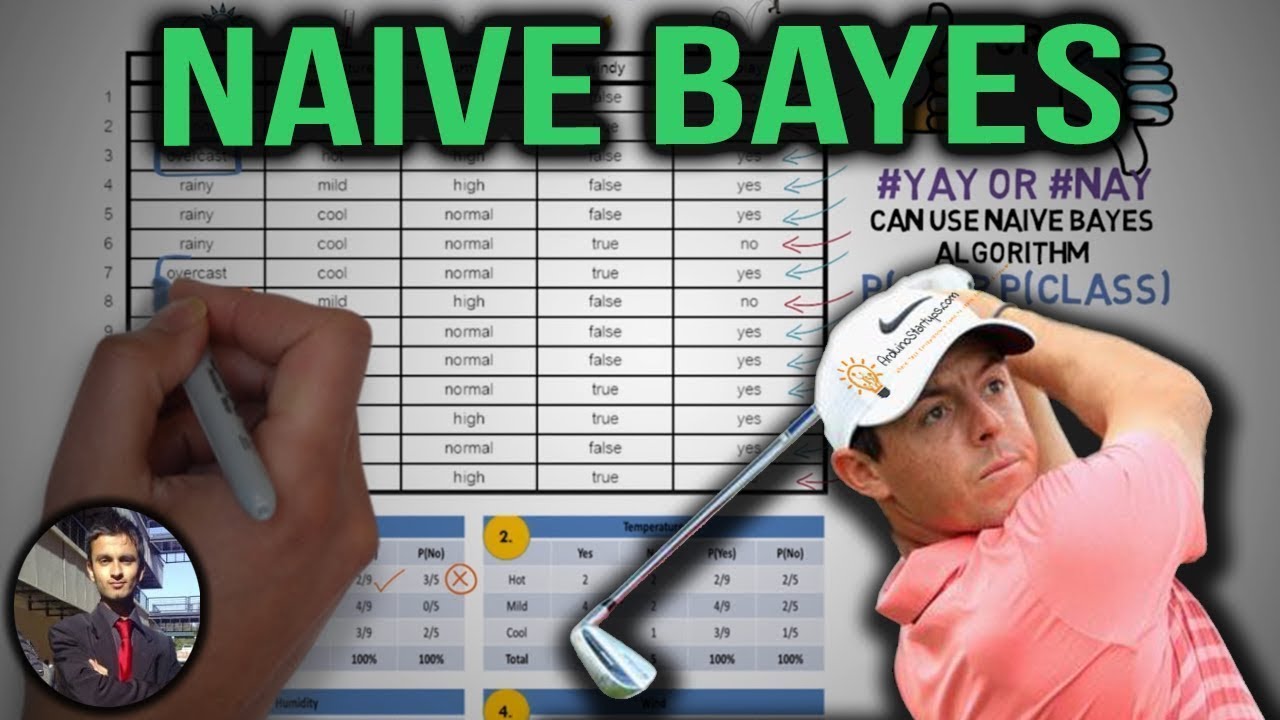
Показать описание
The theory behind the Naïve Bayes Classifier with fun examples and practical uses of it. Watch this video to learn more about it and how to apply it
Want to learn more?
======================================
--------------------------------------------------------------------------------
Now Naïve Bayes is based on Bayes Theorem also known as conditional Theorem, which you can think of it as an evidence theorem or trust theorem. So basically how much can you trust the evidence that is coming in, and it’s a formula that describes how much you should believe the evidence that you are being presented with. An example would be a dog barking in the middle of the night. If the dog always barks for no good reason, you would become desensitized to it and not go check if anything is wrong, this is known as false positives. However if the dog barks only whenever someone enters your premises, you’d be more likely to act on the alert and trust or rely on the evidence from the dog. So Bayes theorem is a mathematic formula for how much you should trust evidence.
So lets take a look deeper at the formula,
• We can start of with the Prior Probability which describes the degree to which we believe the model accurately describes reality based on all of our prior information, So how probable was our hypothesis before observing the evidence.
• Here we have the likelihood which describes how well the model predicts the data. This is term over here is the normalizing constant, the constant that makes the posterior density integrate to one. Like we seen over here.
• And finally the output that we want is the posterior probability which represents the degree to which we believe a given model accurately describes the situation given the available data and all of our prior information. So how probable is our hypothesis given the observed evidence.
So with our example above. We can view the probability that we play golf given it is sunny = the probability that we play golf given a yes times the probability it being sunny divided by probability of a yes. This uses the golf example to explain Naive Bayes.
------------------------------------------------------------
Support us on Patreon
Chat to us on Discord
Interact with us on Facebook
Check my latest work on Instagram
Learn Advanced Tutorials on Udemy
------------------------------------------------------------
To learn more on Artificial Intelligence, Augmented Reality IoT, Deep Learning FPGAs, Arduinos, PCB Design and Image Processing then check out
Please Like and Subscribe for more videos :)
Want to learn more?
======================================
--------------------------------------------------------------------------------
Now Naïve Bayes is based on Bayes Theorem also known as conditional Theorem, which you can think of it as an evidence theorem or trust theorem. So basically how much can you trust the evidence that is coming in, and it’s a formula that describes how much you should believe the evidence that you are being presented with. An example would be a dog barking in the middle of the night. If the dog always barks for no good reason, you would become desensitized to it and not go check if anything is wrong, this is known as false positives. However if the dog barks only whenever someone enters your premises, you’d be more likely to act on the alert and trust or rely on the evidence from the dog. So Bayes theorem is a mathematic formula for how much you should trust evidence.
So lets take a look deeper at the formula,
• We can start of with the Prior Probability which describes the degree to which we believe the model accurately describes reality based on all of our prior information, So how probable was our hypothesis before observing the evidence.
• Here we have the likelihood which describes how well the model predicts the data. This is term over here is the normalizing constant, the constant that makes the posterior density integrate to one. Like we seen over here.
• And finally the output that we want is the posterior probability which represents the degree to which we believe a given model accurately describes the situation given the available data and all of our prior information. So how probable is our hypothesis given the observed evidence.
So with our example above. We can view the probability that we play golf given it is sunny = the probability that we play golf given a yes times the probability it being sunny divided by probability of a yes. This uses the golf example to explain Naive Bayes.
------------------------------------------------------------
Support us on Patreon
Chat to us on Discord
Interact with us on Facebook
Check my latest work on Instagram
Learn Advanced Tutorials on Udemy
------------------------------------------------------------
To learn more on Artificial Intelligence, Augmented Reality IoT, Deep Learning FPGAs, Arduinos, PCB Design and Image Processing then check out
Please Like and Subscribe for more videos :)
Комментарии