filmov
tv
Simple Linear Regression MLE are the same as LSE
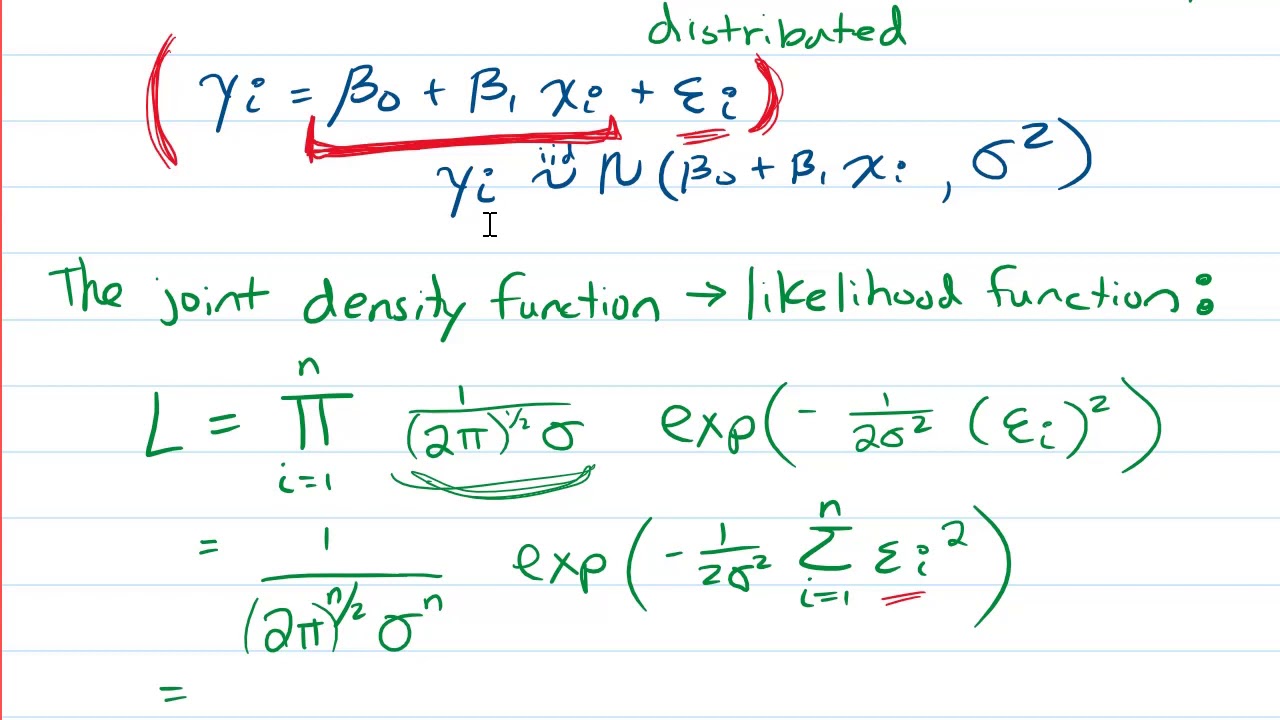
Показать описание
In this video I show that under the normality assumption for the model error, Simple Linear Regression Maximum Likelihood Estimators are the same as Least Squared Estimators
Simple Linear Regression MLE are the same as LSE
Maximum Likelihood Estimation of Parameters in Simple Linear Regression Model
Maximum Likelihood Estimation - Linear regression
Maximum Likelihood Estimation of Regression Parameters | Part 6 of Simple Linear Regression
Maximum Likelihood, clearly explained!!!
MLE vs OLS | Maximum likelihood vs least squares in linear regression
Estimation of coefficient linear regression using maximum likelihood function, manual excel
2. Maximum Likelihood for Regression Coefficients (part 1 of 3)
Linear Regression: MLE for Methods of Estimation
Linear Regression - Normal Distribution, Maximum Likelihood Estimation - I
EE375 Lecture 12e: Regression MLE derivation
Lec 3.4 GSD and MLE in Simple Linear Regression #machinelearning
Simple Linear Regression Derivation of OLS Estimators
Maximum Likelihood Estimation of Regression Parameters | Part 7 of Simple Linear Regression
Estimation Of Parameters In Simple Linear Regression Model
6840-09-21-5: Linear models - Least square estimator as MLE
Deriving the least squares estimators of the slope and intercept (simple linear regression)
Linear Regression as Maximum Likelihood Estimation (Week 07-02)
Simple Linear Regression Using Least Squares Estimators
Simple Linear Regression - Discussion of the Normality Assumption
Simple Linear Regression Concept | Statistics Tutorial #32 | MarinStatsLectures
#2 LINEAR REGRESSION | Incorporating Maximum Likelihood Estimation into Linear Regression Model
Regression 05 - Introduction to Maximum Likelihood Estimation
Linear Regression, A Maximum Likelihood Approach Part 3
Комментарии