filmov
tv
Ray Serve for IOT at Samsara
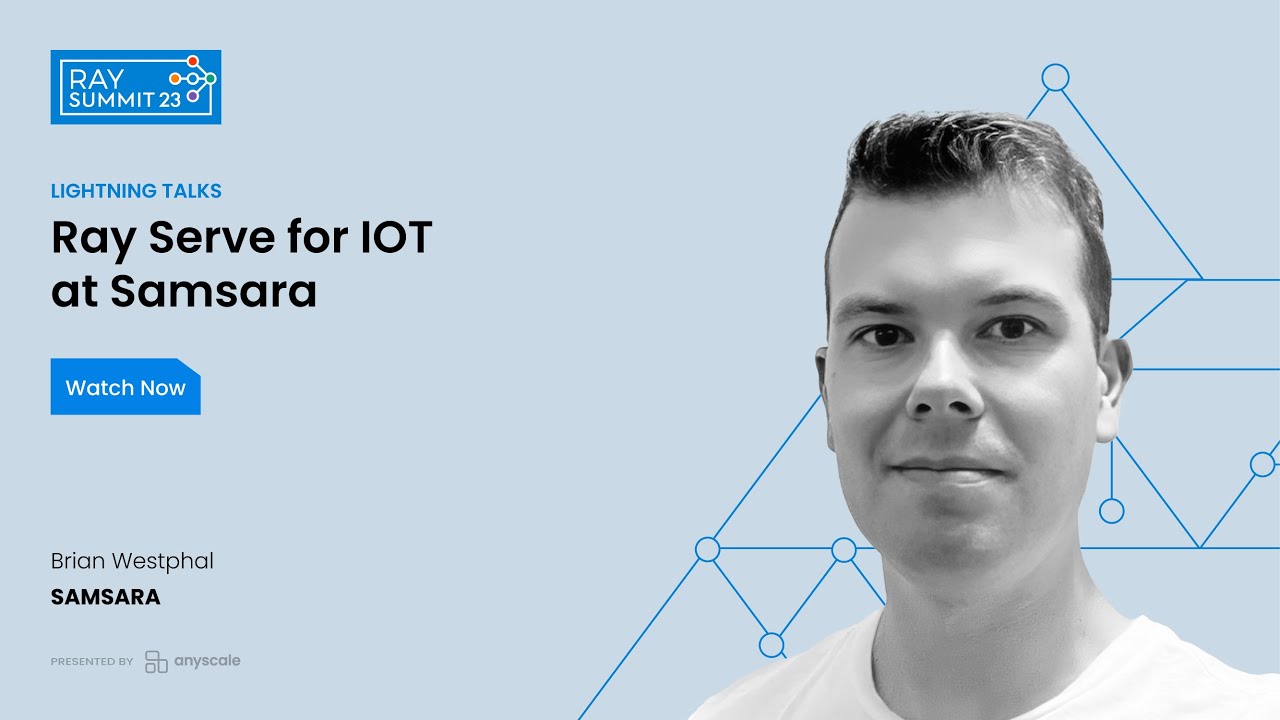
Показать описание
Samsara's machine learning infrastructure team shares how they used Ray serve to scale their cloud IOT inference platform developing computer vision and sensor fusion detection models.
The team will share pragmatic best practices learned creating production serve clusters. Providing demos such as: templates to provision a production cluster setup turn-key, common utilities we've developed, and dashboards for observability and maintenance.
In the IOT space, configuration and customization is key, we'll touch on how Ray serve helped Samsara ML engineering dev-velocity by simplifying the composition of complex pipelines to target our specific customer's use-cases.
(( Key Talking Points ))
(Section) How is Samsara's Machine learning platform special and why did we choose ray serve?
1. For teams already familiar with ray, what's the transition process like?
2. Applications are generally event detection pipelines with configuration and conditionals.
3. Data comes in many forms - computer vision and sensor fusion.
4. Enabling real-time inferences for our application: ex. relevancy.
(Section) Learnings from Deployment
1. Guidelines for cluster configuration - ex. autoscaling, logging.
2. Metrics to monitor - dashboards, what to alert on.
3. Composing pipelines as code.
4. How we maintain our clusters.
(Section) Utility Sharing - Give back to the community
1. Terraform for EKS cluster setup
2. Template/example ray cluster cluster configuration
3. Example complex serve inference pipeline
4. Utils like model LRU cache to share endpoints
About Anyscale
---
Anyscale is the AI Application Platform for developing, running, and scaling AI.
If you're interested in a managed Ray service, check out:
About Ray
---
Ray is the most popular open source framework for scaling and productionizing AI workloads. From Generative AI and LLMs to computer vision, Ray powers the world’s most ambitious AI workloads.
#llm #machinelearning #ray #deeplearning #distributedsystems #python #genai
The team will share pragmatic best practices learned creating production serve clusters. Providing demos such as: templates to provision a production cluster setup turn-key, common utilities we've developed, and dashboards for observability and maintenance.
In the IOT space, configuration and customization is key, we'll touch on how Ray serve helped Samsara ML engineering dev-velocity by simplifying the composition of complex pipelines to target our specific customer's use-cases.
(( Key Talking Points ))
(Section) How is Samsara's Machine learning platform special and why did we choose ray serve?
1. For teams already familiar with ray, what's the transition process like?
2. Applications are generally event detection pipelines with configuration and conditionals.
3. Data comes in many forms - computer vision and sensor fusion.
4. Enabling real-time inferences for our application: ex. relevancy.
(Section) Learnings from Deployment
1. Guidelines for cluster configuration - ex. autoscaling, logging.
2. Metrics to monitor - dashboards, what to alert on.
3. Composing pipelines as code.
4. How we maintain our clusters.
(Section) Utility Sharing - Give back to the community
1. Terraform for EKS cluster setup
2. Template/example ray cluster cluster configuration
3. Example complex serve inference pipeline
4. Utils like model LRU cache to share endpoints
About Anyscale
---
Anyscale is the AI Application Platform for developing, running, and scaling AI.
If you're interested in a managed Ray service, check out:
About Ray
---
Ray is the most popular open source framework for scaling and productionizing AI workloads. From Generative AI and LLMs to computer vision, Ray powers the world’s most ambitious AI workloads.
#llm #machinelearning #ray #deeplearning #distributedsystems #python #genai