filmov
tv
Deploying Many Models Efficiently with Ray Serve
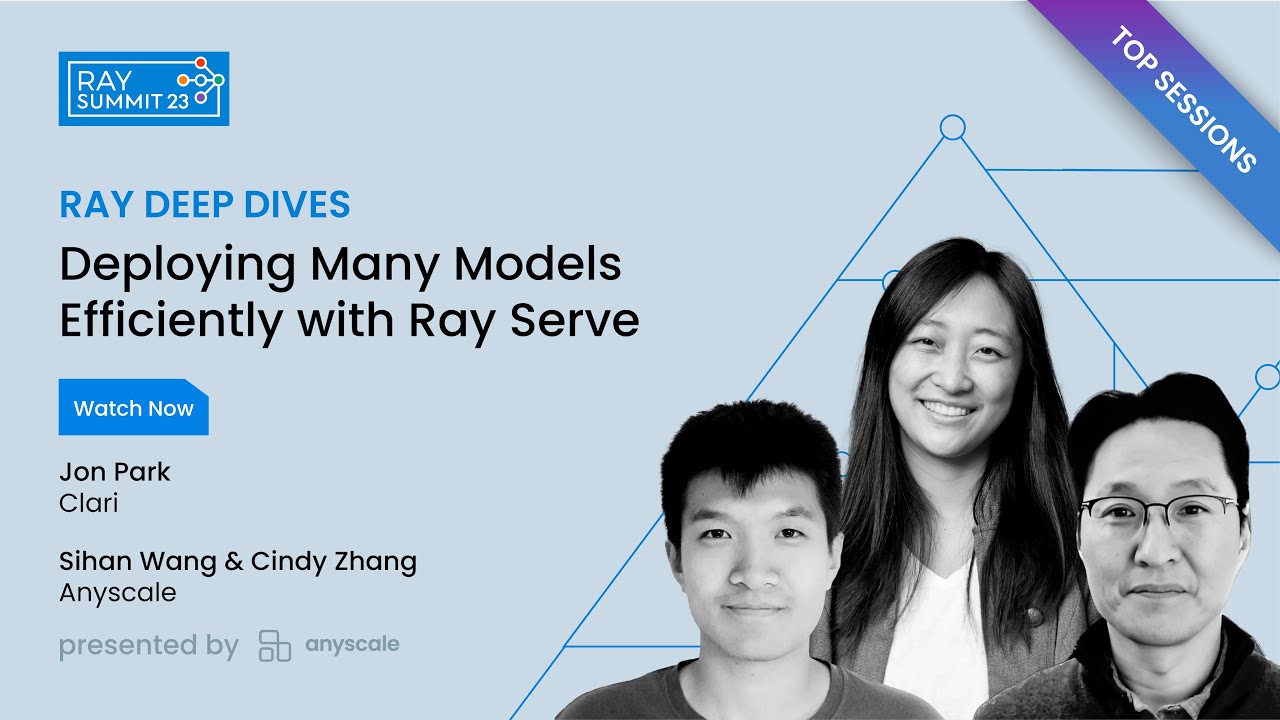
Показать описание
Serving numerous models is essential today due to diverse business needs and various customized use-cases. However, this raises the challenge of how to efficiently deploy and manage these models while considering both ease of use and cost-effectiveness. This talk aims to provide a comprehensive insight into various patterns of serving many models using Ray Serve. We will delve into how 3 features in Ray Serve - model composition, multi-application, model multiplexing - enable seamless deployment of numerous models while optimizing resource utilization.
Takeaways:
• Discuss common industry patterns for serving many models.
• Learn how to simplify management and enhance performance of many-model serving through Ray Serve's model composition, multi-application, and model multiplexing features.
• Deep dive into case studies of Ray Serve users running many-model applications in production.
About Anyscale
---
Anyscale is the AI Application Platform for developing, running, and scaling AI.
If you're interested in a managed Ray service, check out:
About Ray
---
Ray is the most popular open source framework for scaling and productionizing AI workloads. From Generative AI and LLMs to computer vision, Ray powers the world’s most ambitious AI workloads.
#llm #machinelearning #ray #deeplearning #distributedsystems #python #genai
Takeaways:
• Discuss common industry patterns for serving many models.
• Learn how to simplify management and enhance performance of many-model serving through Ray Serve's model composition, multi-application, and model multiplexing features.
• Deep dive into case studies of Ray Serve users running many-model applications in production.
About Anyscale
---
Anyscale is the AI Application Platform for developing, running, and scaling AI.
If you're interested in a managed Ray service, check out:
About Ray
---
Ray is the most popular open source framework for scaling and productionizing AI workloads. From Generative AI and LLMs to computer vision, Ray powers the world’s most ambitious AI workloads.
#llm #machinelearning #ray #deeplearning #distributedsystems #python #genai
Комментарии