filmov
tv
Model calibration
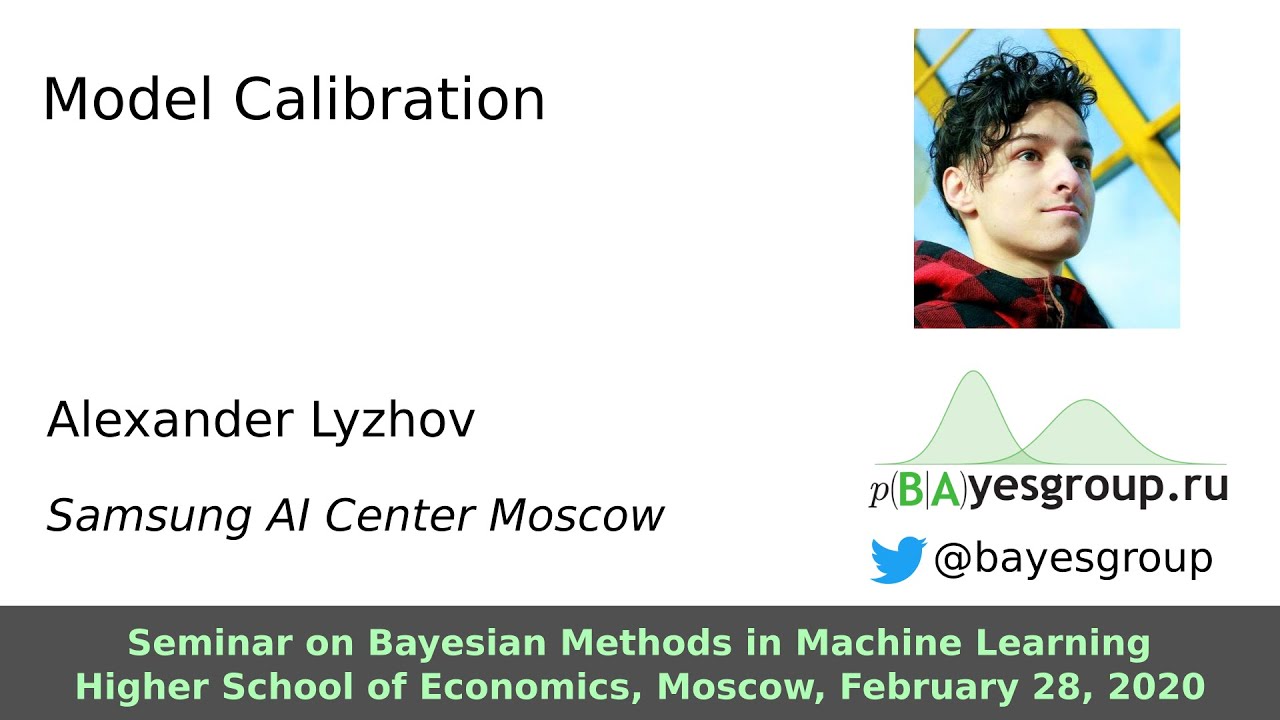
Показать описание
Александр Лыжов, Samsung AI Center Moscow, Research Scientist
In many real-world applications we would like the probabilities that the model outputs (e.g. class probabilities in classification) to be correct in some sense (e.g. to match the actual probabilities of class occurrence). This property of models is called calibration. In this talk I will first do a introduction to various aspects of calibration: definitions of calibration errors, estimators of these errors, calibration of neural networks. Then I will talk about developments that occured in understanding of calibration in 2019 in depth. I want to focus on unbiased calibration estimators and hypothesis testing for calibration in particular. If we have time after that, we may talk about calibration of regression and differentiable calibration losses in neural network training.
In many real-world applications we would like the probabilities that the model outputs (e.g. class probabilities in classification) to be correct in some sense (e.g. to match the actual probabilities of class occurrence). This property of models is called calibration. In this talk I will first do a introduction to various aspects of calibration: definitions of calibration errors, estimators of these errors, calibration of neural networks. Then I will talk about developments that occured in understanding of calibration in 2019 in depth. I want to focus on unbiased calibration estimators and hypothesis testing for calibration in particular. If we have time after that, we may talk about calibration of regression and differentiable calibration losses in neural network training.