filmov
tv
Advanced RAG with Self-Correction | LangGraph | No Hallucination | Agents | LangChain | GROQ | AI
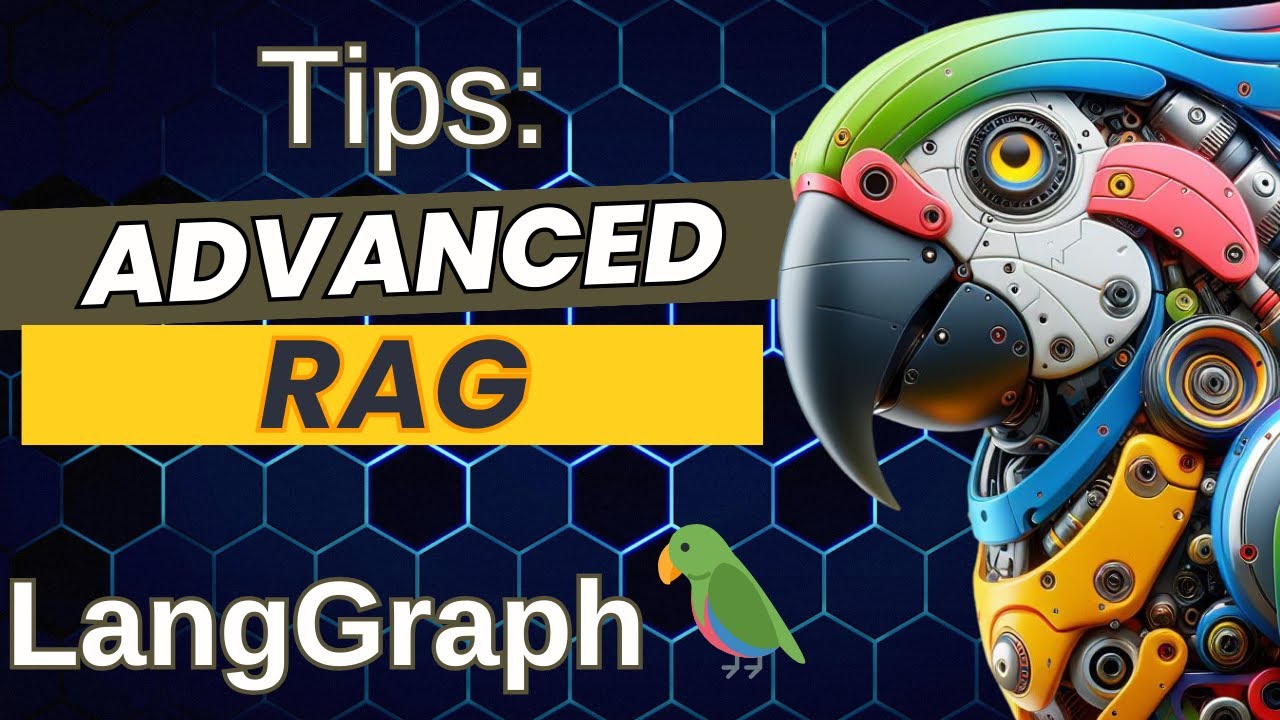
Показать описание
In this video, I'll guide you through building an advanced Retrieval-Augmented Generation (RAG) application using LangGraph. You'll learn step by step how to create an adaptive and self-reflective RAG system, and how to effectively prevent hallucination in your language models.
🔍 Key Topics Covered:
- Adaptive and Self-Reflective RAG: Learn how to design a RAG system that self-corrects to improve its responses.
- Preventing Hallucinations: Discover techniques to ensure your language model provides accurate and reliable information.
- Agents: See how to integrate an agent that uses a search browser to find information when the LLM doesn't have the answer.
- GROQ for LLM inference: Explore how to utilize GROQ to speed-up your LLM responses.
💡 Tools and Technologies:
- LangGraph: The framework I used for building the advanced RAG application.
- Tavily: For agent-based browsing and information retrieval.
- GROQ: To reduce latency on LLM inference.
- Google: To generate text embeddings.
- Chroma: Vector store database.
🔥 Don't forget to 𝘀𝘂𝗯𝘀𝗰𝗿𝗶𝗯𝗲, 𝐬𝐦𝐚𝐬𝐡 the 𝗹𝗶𝗸𝗲 𝐛𝐮𝐭𝐭𝐨𝐧, and 𝐭𝐮𝐫𝐧 𝐨𝐧 the 𝐧𝐨𝐭𝐢𝐟𝐢𝐜𝐚𝐭𝐢𝐨𝐧 𝐛𝐞𝐥𝐥 for more 𝗲𝘅𝗰𝗶𝘁𝗶𝗻𝗴 𝗽𝗿𝗼𝗷𝗲𝗰𝘁𝘀 and 𝘁𝘂𝘁𝗼𝗿𝗶𝗮𝗹𝘀.
🚀 Timestamps:
0:00 Introduction
0:21 Workflow - Flow Diagram of Self-Corrective RAG
03:55 Setup Environment
04:09 Load Credentials
04:22 Routing Node
06:06 Retriever from Chroma Vector Store Database
06:47 Node to Grade Retrieved Documents
07:58 Node to Generate LLM response
08:28 Node to Prevent Hallucination
09:20 Node to Grade Final Answer
09:58 Integrate Agent
10:10 LangGraph for the Flow Diagram
11:28 Test RAG
13:08 Conclusion
Links:
#RAG #LangGraph #AI #LLM #Agents #ArtificialIntelligence #Data #Hallucination #AdaptiveRAG #SelfReflectiveAI #InformationRetrieval #Tavily #GROQ #LLM #Google #Programming #Tutorial #DataScience #Embeddings #AIResearch #Tutorial #MachineLearning #Database #Python
🔍 Key Topics Covered:
- Adaptive and Self-Reflective RAG: Learn how to design a RAG system that self-corrects to improve its responses.
- Preventing Hallucinations: Discover techniques to ensure your language model provides accurate and reliable information.
- Agents: See how to integrate an agent that uses a search browser to find information when the LLM doesn't have the answer.
- GROQ for LLM inference: Explore how to utilize GROQ to speed-up your LLM responses.
💡 Tools and Technologies:
- LangGraph: The framework I used for building the advanced RAG application.
- Tavily: For agent-based browsing and information retrieval.
- GROQ: To reduce latency on LLM inference.
- Google: To generate text embeddings.
- Chroma: Vector store database.
🔥 Don't forget to 𝘀𝘂𝗯𝘀𝗰𝗿𝗶𝗯𝗲, 𝐬𝐦𝐚𝐬𝐡 the 𝗹𝗶𝗸𝗲 𝐛𝐮𝐭𝐭𝐨𝐧, and 𝐭𝐮𝐫𝐧 𝐨𝐧 the 𝐧𝐨𝐭𝐢𝐟𝐢𝐜𝐚𝐭𝐢𝐨𝐧 𝐛𝐞𝐥𝐥 for more 𝗲𝘅𝗰𝗶𝘁𝗶𝗻𝗴 𝗽𝗿𝗼𝗷𝗲𝗰𝘁𝘀 and 𝘁𝘂𝘁𝗼𝗿𝗶𝗮𝗹𝘀.
🚀 Timestamps:
0:00 Introduction
0:21 Workflow - Flow Diagram of Self-Corrective RAG
03:55 Setup Environment
04:09 Load Credentials
04:22 Routing Node
06:06 Retriever from Chroma Vector Store Database
06:47 Node to Grade Retrieved Documents
07:58 Node to Generate LLM response
08:28 Node to Prevent Hallucination
09:20 Node to Grade Final Answer
09:58 Integrate Agent
10:10 LangGraph for the Flow Diagram
11:28 Test RAG
13:08 Conclusion
Links:
#RAG #LangGraph #AI #LLM #Agents #ArtificialIntelligence #Data #Hallucination #AdaptiveRAG #SelfReflectiveAI #InformationRetrieval #Tavily #GROQ #LLM #Google #Programming #Tutorial #DataScience #Embeddings #AIResearch #Tutorial #MachineLearning #Database #Python
Комментарии