filmov
tv
Risks and Opportunities of AI in Clinical Drug Development | David Madigan and Demissie Alemayehu
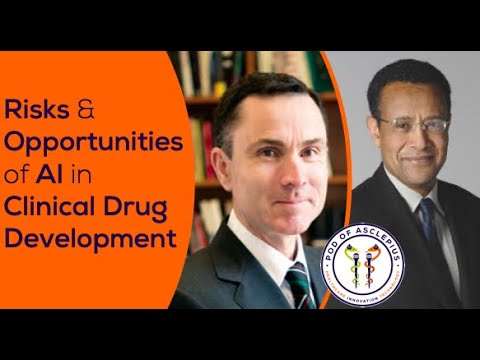
Показать описание
There are many places in which ML/AI methods can be of benefit to pharmaceutical research (several have already been covered on the show). David and Demissie explain where AI can fit in to in vivo studies, which carries it’s own benefits, but also with heightened risk to to human test subjects. They go on to cover several other areas of interest including AI for observation studies and real world evidence. It’s a “big tent” conversation as we lead up to the Pfizer/ASA/Columbia University Symposium on Risks and Opportunities of AI in Clinical Drug Development. Mihaela van der Schaar will be following up in a subsequent episode on this subject.
Let us know in the comment section if you have any questions. We hope you enjoy the episode and if you do, please leave a like and subscribe to the channel. Every bit helps us grow and continue to produce this podcast for you, so please consider sharing this episode with your friends, your lab, or your department.
__Table of Contents__
0:00 – Introduction
0:55 – David Madigan, Prof. Columbia – background & interests
1:55 – Demissie Alemayehu, VP Pfizer – role of AI in novel trial designs
3:52 – Leveraging data using historical controls and causal inference
5:52 – Defining & redefining AI in Pharma: Big tent of extracting value
9:25 – Conference theme: a mix of bright & engaging presenters
11:05 – Mitigating risks: from first-time-in-humans to personalized medicine
14:28 – Bias from treatment contamination by Amol Navathe, UPenn
16:34 – Treatment polluted outcomes – a prediction paradox
22:05 – AI Opportunities in automating trial efficiency and prediction
26:10 – Opportunities in personalized medicine
27:04 – In search of the Holy Grail of large-scale electronic health records
28:55 – Privacy and commercial limits to accessing data
30:12 – Need for generative models to share high-fidelity EHR data
32:50 – OHDSI’s 500 million patient database
35:42 – Estimating is not the same as knowing the causal effect
37:37 – Negative controls and synthetic positive controls
41:00 – Does integrating PK/PD and agent-based models add value?
44:18 – Making treatment groups comparable by Adler Perotte
48:38 – Conference take-aways
49:39 – Announcing new ACM-IMS Foundations of Data Science Conference, Oct. 18-20 in Seattle.
#artificialintelligence
#healthcaretechnology
#datascience
#machinelearning
#healthcareinnovation
#statistics
Let us know in the comment section if you have any questions. We hope you enjoy the episode and if you do, please leave a like and subscribe to the channel. Every bit helps us grow and continue to produce this podcast for you, so please consider sharing this episode with your friends, your lab, or your department.
__Table of Contents__
0:00 – Introduction
0:55 – David Madigan, Prof. Columbia – background & interests
1:55 – Demissie Alemayehu, VP Pfizer – role of AI in novel trial designs
3:52 – Leveraging data using historical controls and causal inference
5:52 – Defining & redefining AI in Pharma: Big tent of extracting value
9:25 – Conference theme: a mix of bright & engaging presenters
11:05 – Mitigating risks: from first-time-in-humans to personalized medicine
14:28 – Bias from treatment contamination by Amol Navathe, UPenn
16:34 – Treatment polluted outcomes – a prediction paradox
22:05 – AI Opportunities in automating trial efficiency and prediction
26:10 – Opportunities in personalized medicine
27:04 – In search of the Holy Grail of large-scale electronic health records
28:55 – Privacy and commercial limits to accessing data
30:12 – Need for generative models to share high-fidelity EHR data
32:50 – OHDSI’s 500 million patient database
35:42 – Estimating is not the same as knowing the causal effect
37:37 – Negative controls and synthetic positive controls
41:00 – Does integrating PK/PD and agent-based models add value?
44:18 – Making treatment groups comparable by Adler Perotte
48:38 – Conference take-aways
49:39 – Announcing new ACM-IMS Foundations of Data Science Conference, Oct. 18-20 in Seattle.
#artificialintelligence
#healthcaretechnology
#datascience
#machinelearning
#healthcareinnovation
#statistics
Комментарии