filmov
tv
Concept Activation Vectors for Generating User-Defined 3D Shapes | Monolith
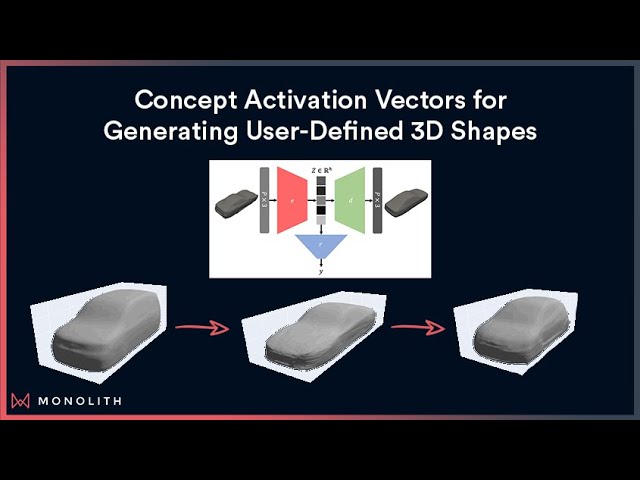
Показать описание
Abstract
=======
We explore the interpretability of 3D geometric deep learning models in the context of Computer-Aided Design (CAD). The field of parametric CAD can be limited by the difficulty of expressing high-level design concepts in terms of a few numeric parameters. In this paper, we use a deep learning architectures to encode high dimensional 3D shapes into a vectorized latent representation that can be used to describe arbitrary concepts. Specifically, we train a simple auto-encoder to parameterize a dataset of complex shapes.
To understand the latent encoded space, we use the idea of Concept Activation Vectors (CAV) to reinterpret the latent space in terms of user-defined concepts. This allows modification of a reference design to exhibit more or fewer characteristics of a chosen concept or group of concepts. We also test the statistical significance of the identified concepts and determine the sensitivity of a physical quantity of interest across the dataset.
⚠️ This work was done in the scope of the Innovate UK grant on "Explainable AI system to rationalise accelerated decision making on automotive component performance and manufacturability" [Project 10009522].
#machinelearning #deeplearning #xai