filmov
tv
Model-based Reinforcement Learning for Optimal Feedback Control of Switched Systems
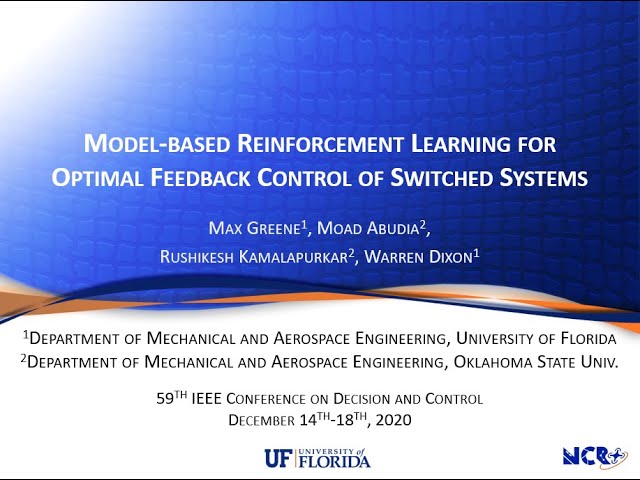
Показать описание
Presented at the 59th Conference on Decision and Control –Jeju Island, Republic of Korea (Dec. 14th-18th, 2020)
This paper examines the use of reinforcement learning-based controllers to approximate multiple value functions of specific classes of subsystems while following a switching sequence. Each subsystem may have varying characteristics, such as different cost or different system dynamics. Stability of the overall switching sequence is proven using Lyapunov-based analysis techniques. Specifically, Lyapunov-based methods are developed to prove boundedness of individual subsystems and to determine a minimum dwell-time condition to ensure stability of the overall switching sequence. Uniformly ultimately bounded regulation of the states, approximation of the value function, and approximation of the optimal control policy is achieved for arbitrary switching sequences provided the minimum dwell-time condition is satisfied.
0:00 Introduction
1:35 Approximate Dynamic Programming (ADP)
3:44 Switched System ADP Problem Formulation
7:37 Assumptions
8:23 Theorem Statements
10:24 Simulations
12:22 Conclusion
This paper examines the use of reinforcement learning-based controllers to approximate multiple value functions of specific classes of subsystems while following a switching sequence. Each subsystem may have varying characteristics, such as different cost or different system dynamics. Stability of the overall switching sequence is proven using Lyapunov-based analysis techniques. Specifically, Lyapunov-based methods are developed to prove boundedness of individual subsystems and to determine a minimum dwell-time condition to ensure stability of the overall switching sequence. Uniformly ultimately bounded regulation of the states, approximation of the value function, and approximation of the optimal control policy is achieved for arbitrary switching sequences provided the minimum dwell-time condition is satisfied.
0:00 Introduction
1:35 Approximate Dynamic Programming (ADP)
3:44 Switched System ADP Problem Formulation
7:37 Assumptions
8:23 Theorem Statements
10:24 Simulations
12:22 Conclusion