filmov
tv
ETH Zürich DLSC: Introduction to Operator Learning Part 1
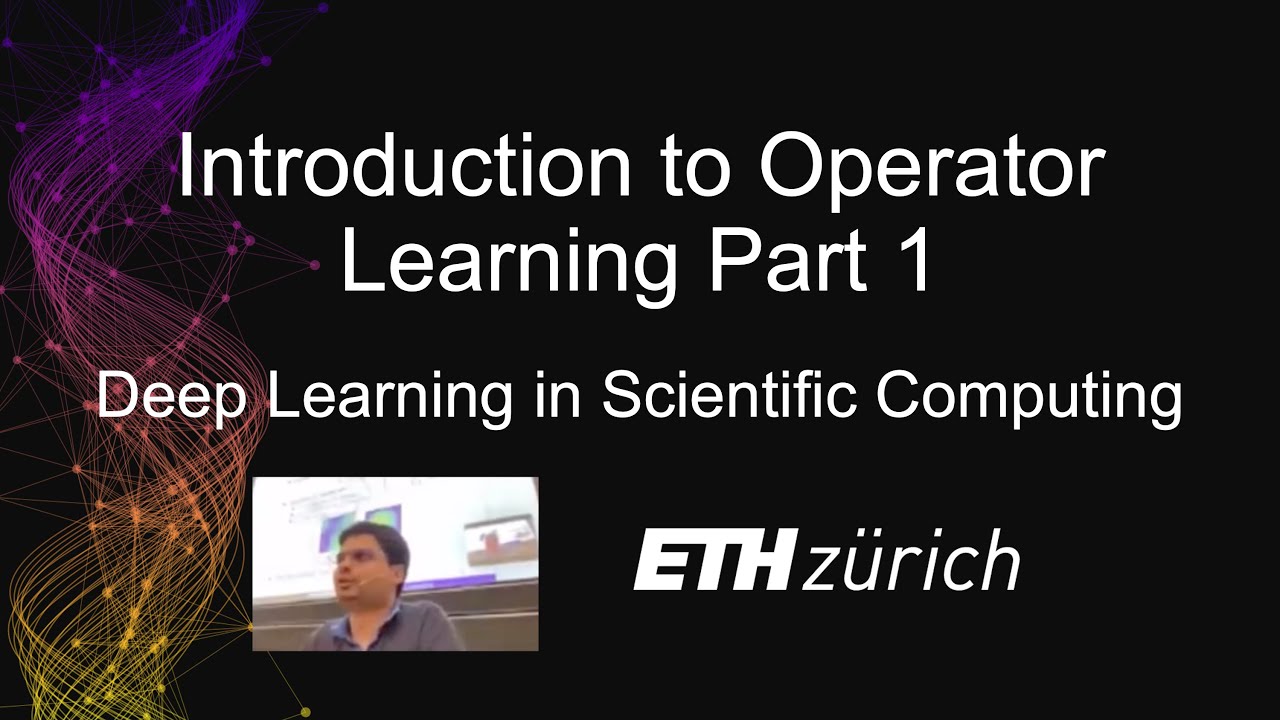
Показать описание
↓↓↓ LECTURE OVERVIEW BELOW ↓↓↓
ETH Zürich Deep Learning in Scientific Computing 2023
Lecture 7: Introduction to Operator Learning Part 1
Lecturers: Ben Moseley and Siddhartha Mishra
▬ Lecture Content ▬▬▬▬▬▬▬▬▬
0:00 - Using supervised learning to solve partial differential equations (PDEs)
2:24 - What does it mean to solve a PDE?
12:45 - The task of learning operators
18:18 - Solving parametric PDEs with deep learning
33:37 - Caveats of using deep learning
50:38 - Using low discrepancy sequences to improve performance
1:04:43 - Using deep learning for uncertainty quantification
▬ Course Overview ▬▬▬▬▬▬▬▬▬
▬ Course Learning Objectives ▬▬▬▬▬
The objective of this course is to introduce students to advanced applications of deep learning in scientific computing. The focus will be on the design and implementation of algorithms as well as on the underlying theory that guarantees reliability of the algorithms. We provide several examples of applications in science and engineering where deep learning based algorithms outperform state of the art methods.
By the end of the course you should be:
- Aware of advanced applications of deep learning in scientific computing
- Familiar with the design, implementation and theory of these algorithms
- Understand the pros/cons of using deep learning
- Understand key scientific machine learning concepts and themes
ETH Zürich Deep Learning in Scientific Computing 2023
Lecture 7: Introduction to Operator Learning Part 1
Lecturers: Ben Moseley and Siddhartha Mishra
▬ Lecture Content ▬▬▬▬▬▬▬▬▬
0:00 - Using supervised learning to solve partial differential equations (PDEs)
2:24 - What does it mean to solve a PDE?
12:45 - The task of learning operators
18:18 - Solving parametric PDEs with deep learning
33:37 - Caveats of using deep learning
50:38 - Using low discrepancy sequences to improve performance
1:04:43 - Using deep learning for uncertainty quantification
▬ Course Overview ▬▬▬▬▬▬▬▬▬
▬ Course Learning Objectives ▬▬▬▬▬
The objective of this course is to introduce students to advanced applications of deep learning in scientific computing. The focus will be on the design and implementation of algorithms as well as on the underlying theory that guarantees reliability of the algorithms. We provide several examples of applications in science and engineering where deep learning based algorithms outperform state of the art methods.
By the end of the course you should be:
- Aware of advanced applications of deep learning in scientific computing
- Familiar with the design, implementation and theory of these algorithms
- Understand the pros/cons of using deep learning
- Understand key scientific machine learning concepts and themes