filmov
tv
ETH Zürich DLSC: Physics-Informed Neural Networks - Applications
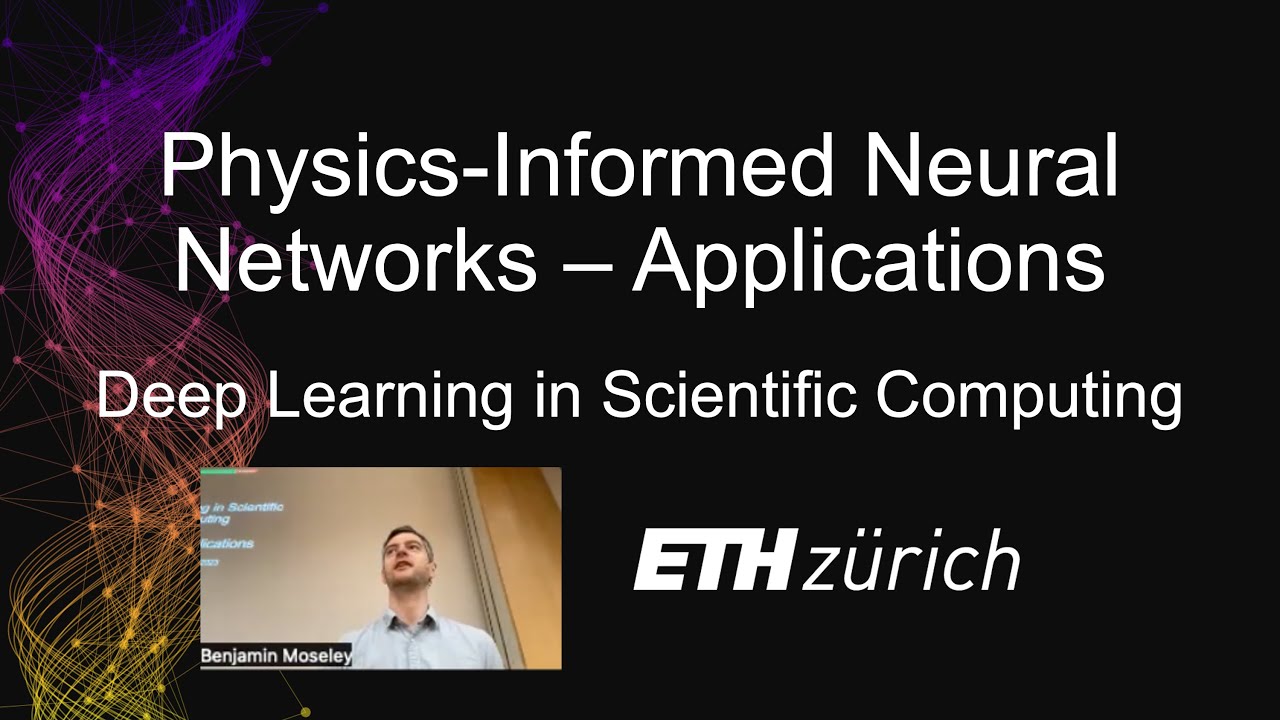
Показать описание
↓↓↓ LECTURE OVERVIEW BELOW ↓↓↓
ETH Zürich Deep Learning in Scientific Computing 2023
Lecture 5: Physics-Informed Neural Networks - Applications
Lecturers: Ben Moseley and Siddhartha Mishra
▬ Lecture Content ▬▬▬▬▬▬▬▬▬
0:00 - Lecture overview
1:45 - What is a physics-informed neural network (PINN)?
11:59 - PINNs as a general framework
17:17 - PINNs for solving the Burgers' equation
20:20 - How to train PINNs
39:42 - Training considerations
44:27 - [break - please skip]
53:07 - Simulation with PINNs
1:00:14 - Solving inverse problems with PINNs
1:24:10 - Equation discovery with PINNs
▬ Course Overview ▬▬▬▬▬▬▬▬▬
▬ Course Learning Objectives ▬▬▬▬▬
The objective of this course is to introduce students to advanced applications of deep learning in scientific computing. The focus will be on the design and implementation of algorithms as well as on the underlying theory that guarantees reliability of the algorithms. We provide several examples of applications in science and engineering where deep learning based algorithms outperform state of the art methods.
By the end of the course you should be:
- Aware of advanced applications of deep learning in scientific computing
- Familiar with the design, implementation and theory of these algorithms
- Understand the pros/cons of using deep learning
- Understand key scientific machine learning concepts and themes
ETH Zürich Deep Learning in Scientific Computing 2023
Lecture 5: Physics-Informed Neural Networks - Applications
Lecturers: Ben Moseley and Siddhartha Mishra
▬ Lecture Content ▬▬▬▬▬▬▬▬▬
0:00 - Lecture overview
1:45 - What is a physics-informed neural network (PINN)?
11:59 - PINNs as a general framework
17:17 - PINNs for solving the Burgers' equation
20:20 - How to train PINNs
39:42 - Training considerations
44:27 - [break - please skip]
53:07 - Simulation with PINNs
1:00:14 - Solving inverse problems with PINNs
1:24:10 - Equation discovery with PINNs
▬ Course Overview ▬▬▬▬▬▬▬▬▬
▬ Course Learning Objectives ▬▬▬▬▬
The objective of this course is to introduce students to advanced applications of deep learning in scientific computing. The focus will be on the design and implementation of algorithms as well as on the underlying theory that guarantees reliability of the algorithms. We provide several examples of applications in science and engineering where deep learning based algorithms outperform state of the art methods.
By the end of the course you should be:
- Aware of advanced applications of deep learning in scientific computing
- Familiar with the design, implementation and theory of these algorithms
- Understand the pros/cons of using deep learning
- Understand key scientific machine learning concepts and themes
Комментарии