filmov
tv
Correlation Doesn't Mean Causation
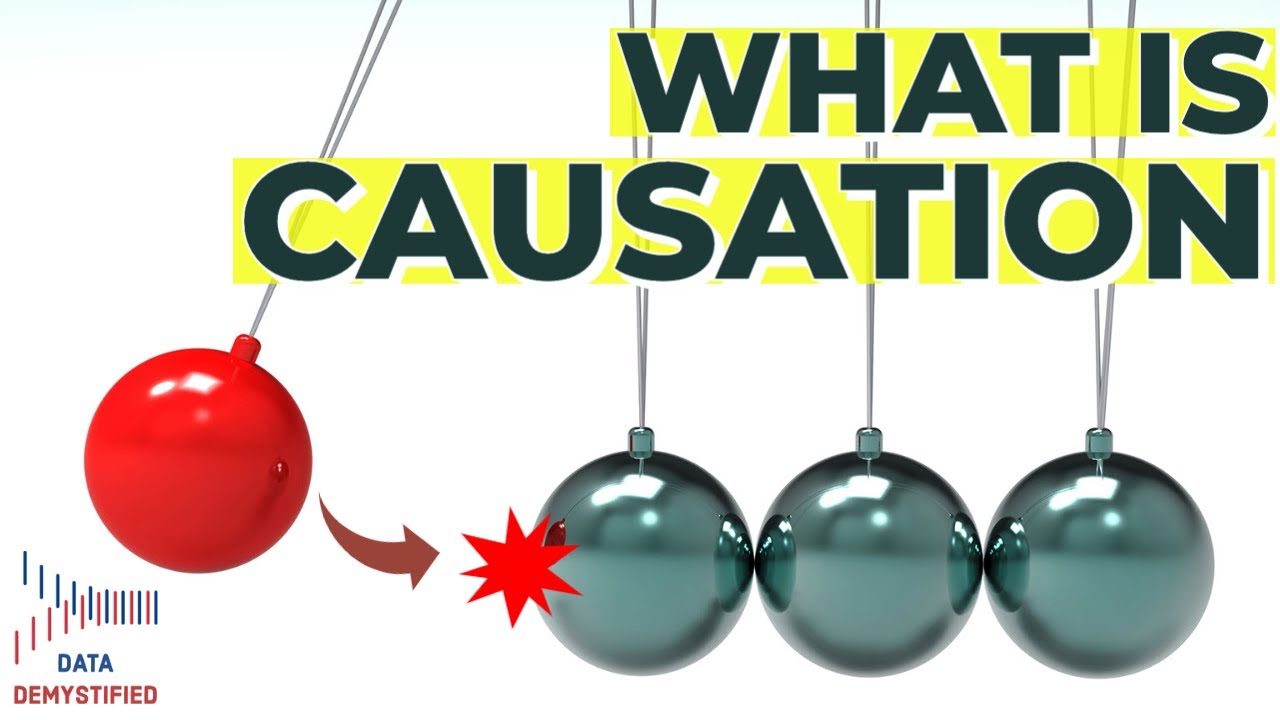
Показать описание
You’ve probably heard something like "correlation doesn’t imply causation.” That’s true, but then what does imply causation? In short, the only way to really make a causal claim is to run a randomized experiment. If you do that, you can claim causation all day long. If you stick around, I’ll teach you what a randomized experiment is, how it can be used to make critically important causal claims like whether a vaccine is effective at preventing a disease or whether a flashier YouTube thumbnail gets content creators more views. And, beyond that, yes, I’ll even explain why correlation doesn't imply causation.
So here’s the game plan. I’m going to first very quickly explain what a correlation is, then explain why we REALLY care about making causal statements, show you what does actually allow you to make all important causal claims, and, finally show you why correlations don’t necessarily imply causation. I’ll do all this with as little jargon as possible and an emphasis on intuition.
I do this all without ever using any fancy jargon or any complex math. I focus on intuition and and insights to help you get an intuitive understanding of complex, important, and timely topics in statistics, data science, and data analytics.
Follow me at:
Equipment Used for Filming:
So here’s the game plan. I’m going to first very quickly explain what a correlation is, then explain why we REALLY care about making causal statements, show you what does actually allow you to make all important causal claims, and, finally show you why correlations don’t necessarily imply causation. I’ll do all this with as little jargon as possible and an emphasis on intuition.
I do this all without ever using any fancy jargon or any complex math. I focus on intuition and and insights to help you get an intuitive understanding of complex, important, and timely topics in statistics, data science, and data analytics.
Follow me at:
Equipment Used for Filming:
Комментарии