filmov
tv
Nanotechnology’s Hidden Errors: Beyond Noise and Variability
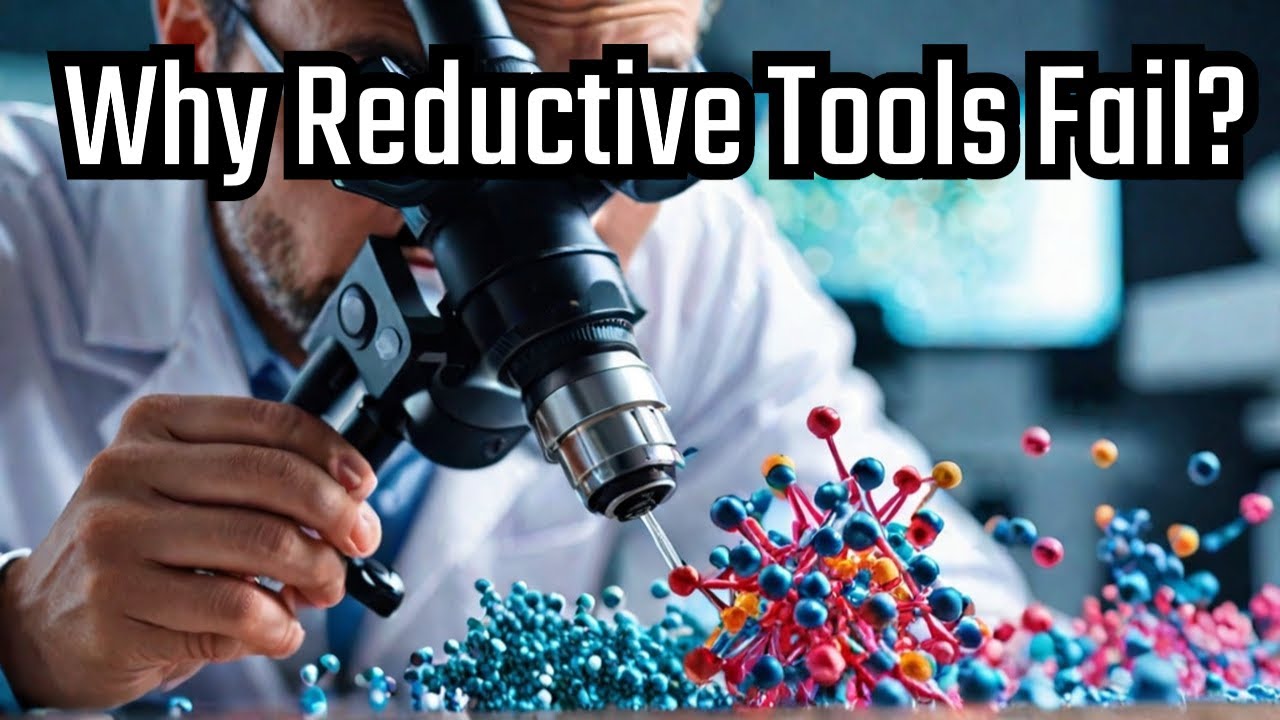
Показать описание
Discover how reductive tools fall short in nanotechnology by misinterpreting variability and randomness. Learn why molecular systems at the nanoscale rely on unpredictability to function, unlike traditional machines. In this video, we explore the concept of nanotechnology variability: reductive errors beyond the noise and its limitations when applied to biological systems. The discussion focuses on how randomness and nonlinear dynamics are crucial at the nano scale, contrasting with traditional, predictable models used in larger systems.
#Reductionism #Nanotechnology #ComplexSystems #EmergentProperties #BiologicalSystems
Key Points: 🔍 Introduction to Reductionism:
Learn about the scientific process of breaking down complex phenomena into smaller, understandable components, a method that has led to breakthroughs in physics and chemistry but struggles with more complex systems.
💡 Nanotechnology and Randomness:
At the nano scale, randomness in movement, energy, and behavior is not just noise but a fundamental feature. Molecular motors like ATP synthase work not because they overcome randomness but because they exploit it.
🎬 Challenges in Synthetic Biology:
Efforts to create synthetic cells face issues because synthetic materials are often too rigid compared to the flexible, adaptive nature of biological systems. This highlights the difficulty of replicating natural processes with traditional, linear approaches.
🌟 Emergent Properties:
The video delves into the concept of emergent behaviors, where complex actions arise from simple interactions, such as the behavior of an ant colony or ecosystem, which cannot be fully explained by just studying individual components.
🔬 Fractals and Chaos Theory:
Fractal patterns are common in biological systems (e.g., blood vessels and neurons), and chaos theory explains how minor variations in initial conditions, like the butterfly effect, can lead to vastly different outcomes, as seen in weather systems and climate change.
🚀 Biological Systems and Feedback Loops:
Unlike mechanical systems, biological systems are dynamic and rely on feedback loops and variability to adapt to their environment. This adaptability is key to survival in unpredictable environments, as seen in the immune system.
🌐 Ethical Considerations in Complex Systems:
The video concludes by highlighting the need for interdisciplinary collaboration and addressing the ethical implications of manipulating complex systems, especially given the unpredictability of these systems.
Context Timestamps:
00:00 - Introduction to Reductionism
01:15 - Nanotechnology and Randomness at the Nano Scale
03:40 - Challenges in Synthetic Biology and Nanotechnology
06:15 - Emergent Properties in Complex Systems
08:45 - Fractals and Chaos Theory in Biological Systems
11:30 - Feedback Loops and Adaptability in Biology
14:50 - Ethical Considerations in Complex Systems
17:30 - The Future of Complex Systems and Interdisciplinary Approaches
19:30 - Conclusion: Embracing Complexity in Science
This video offers a comprehensive exploration of reductionism’s limitations, emphasizing the need to embrace randomness and complexity at the nano scale. It highlights how new scientific approaches must account for emergent behaviors and dynamic systems to achieve breakthroughs in nanotechnology and synthetic biology.
#Reductionism #Nanotechnology #ComplexSystems #EmergentProperties #BiologicalSystems
Key Points: 🔍 Introduction to Reductionism:
Learn about the scientific process of breaking down complex phenomena into smaller, understandable components, a method that has led to breakthroughs in physics and chemistry but struggles with more complex systems.
💡 Nanotechnology and Randomness:
At the nano scale, randomness in movement, energy, and behavior is not just noise but a fundamental feature. Molecular motors like ATP synthase work not because they overcome randomness but because they exploit it.
🎬 Challenges in Synthetic Biology:
Efforts to create synthetic cells face issues because synthetic materials are often too rigid compared to the flexible, adaptive nature of biological systems. This highlights the difficulty of replicating natural processes with traditional, linear approaches.
🌟 Emergent Properties:
The video delves into the concept of emergent behaviors, where complex actions arise from simple interactions, such as the behavior of an ant colony or ecosystem, which cannot be fully explained by just studying individual components.
🔬 Fractals and Chaos Theory:
Fractal patterns are common in biological systems (e.g., blood vessels and neurons), and chaos theory explains how minor variations in initial conditions, like the butterfly effect, can lead to vastly different outcomes, as seen in weather systems and climate change.
🚀 Biological Systems and Feedback Loops:
Unlike mechanical systems, biological systems are dynamic and rely on feedback loops and variability to adapt to their environment. This adaptability is key to survival in unpredictable environments, as seen in the immune system.
🌐 Ethical Considerations in Complex Systems:
The video concludes by highlighting the need for interdisciplinary collaboration and addressing the ethical implications of manipulating complex systems, especially given the unpredictability of these systems.
Context Timestamps:
00:00 - Introduction to Reductionism
01:15 - Nanotechnology and Randomness at the Nano Scale
03:40 - Challenges in Synthetic Biology and Nanotechnology
06:15 - Emergent Properties in Complex Systems
08:45 - Fractals and Chaos Theory in Biological Systems
11:30 - Feedback Loops and Adaptability in Biology
14:50 - Ethical Considerations in Complex Systems
17:30 - The Future of Complex Systems and Interdisciplinary Approaches
19:30 - Conclusion: Embracing Complexity in Science
This video offers a comprehensive exploration of reductionism’s limitations, emphasizing the need to embrace randomness and complexity at the nano scale. It highlights how new scientific approaches must account for emergent behaviors and dynamic systems to achieve breakthroughs in nanotechnology and synthetic biology.
Комментарии