filmov
tv
Building a RAG System With Google Gemma, Hugging Face and MongoDB
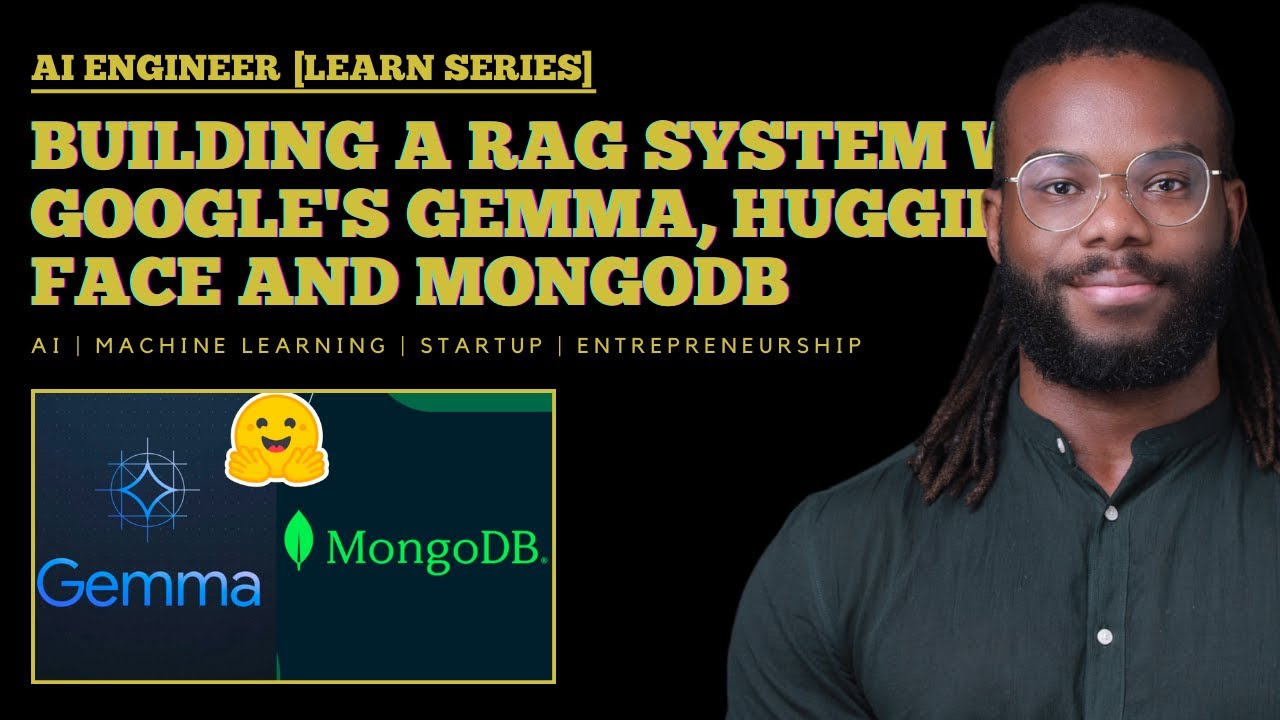
Показать описание
In this video, we will walk you through the process of building a RAG system using the Google's Gemma open model, GTE embedding models and MongoDB as the vector database.
We will be using Hugging Face as the model provider for this stack.
By the end of this video, you will have a clear understanding of how to build a RAG system using the latest Gemma model and MongoDB
⏱️ Timestamps
00:00 Introduction to the video topic and resources
01:06 Overview of Google's new open model - Gemma
01:35 Accessing Gemma models via Hugging Face
01:49 Setting up the development environment with necessary libraries
03:28 Loading and preparing the dataset for the recommender system
04:45 Exploring and selecting embedding models from Hugging Face
06:03 Encoding text to numerical representation with sentence transformers
07:00 Setting up and connecting to MongoDB database and collection
08:50 Creating a vector search index in MongoDB
10:50 Ingesting data into MongoDB and
13:05 Executing a vector search
14:55 Formatting and obtaining search results from the vector search
15:45 Crafting a user query for the recommender system
16:42 Utilizing Gemma for generating responses to user queries
19:00 Conclusion and invitation to subscribe to the channel
🧾 Article:
💻 Code:
📈 Hugging Face Dataset:
Thanks for Watching.
#artificialintelligence #machinelearning #aiengineer #openai #llamaindex
We will be using Hugging Face as the model provider for this stack.
By the end of this video, you will have a clear understanding of how to build a RAG system using the latest Gemma model and MongoDB
⏱️ Timestamps
00:00 Introduction to the video topic and resources
01:06 Overview of Google's new open model - Gemma
01:35 Accessing Gemma models via Hugging Face
01:49 Setting up the development environment with necessary libraries
03:28 Loading and preparing the dataset for the recommender system
04:45 Exploring and selecting embedding models from Hugging Face
06:03 Encoding text to numerical representation with sentence transformers
07:00 Setting up and connecting to MongoDB database and collection
08:50 Creating a vector search index in MongoDB
10:50 Ingesting data into MongoDB and
13:05 Executing a vector search
14:55 Formatting and obtaining search results from the vector search
15:45 Crafting a user query for the recommender system
16:42 Utilizing Gemma for generating responses to user queries
19:00 Conclusion and invitation to subscribe to the channel
🧾 Article:
💻 Code:
📈 Hugging Face Dataset:
Thanks for Watching.
#artificialintelligence #machinelearning #aiengineer #openai #llamaindex
Комментарии