filmov
tv
Dimensionality Reduction via Principal Component Analysis
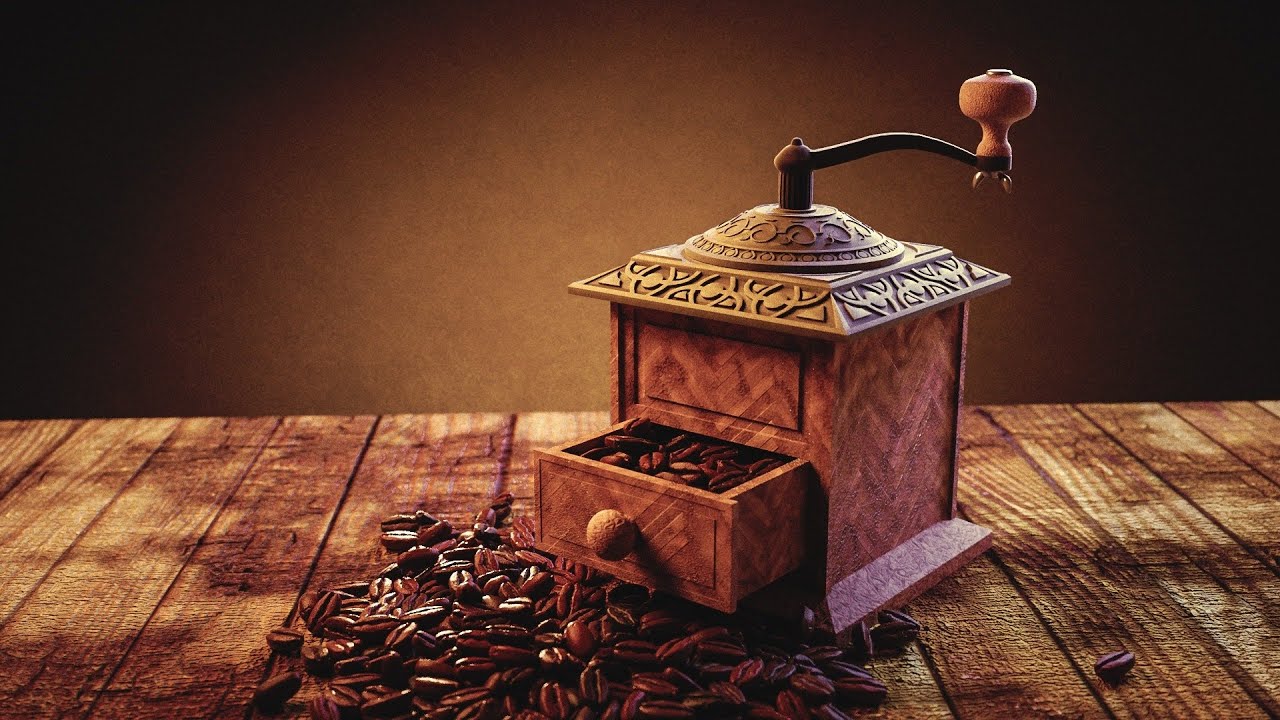
Показать описание
Datasets with high dimensionality, where each data point is associated to many features, can be difficult to process and analyze. The goal of dimensionality reduction is to embed such data in a lower-dimensional space, while preserving as much information as possible. In this video we explain how to use principal component analysis (PCA) for this purpose, illustrating the technique on two datasets. We then prove that PCA-based dimensionality reduction is optimal, in the sense that it is the linear approach that preserves the most variance.