filmov
tv
Feature Extraction of Hyperspectral Images With Image Fusion and Recursive Filtering
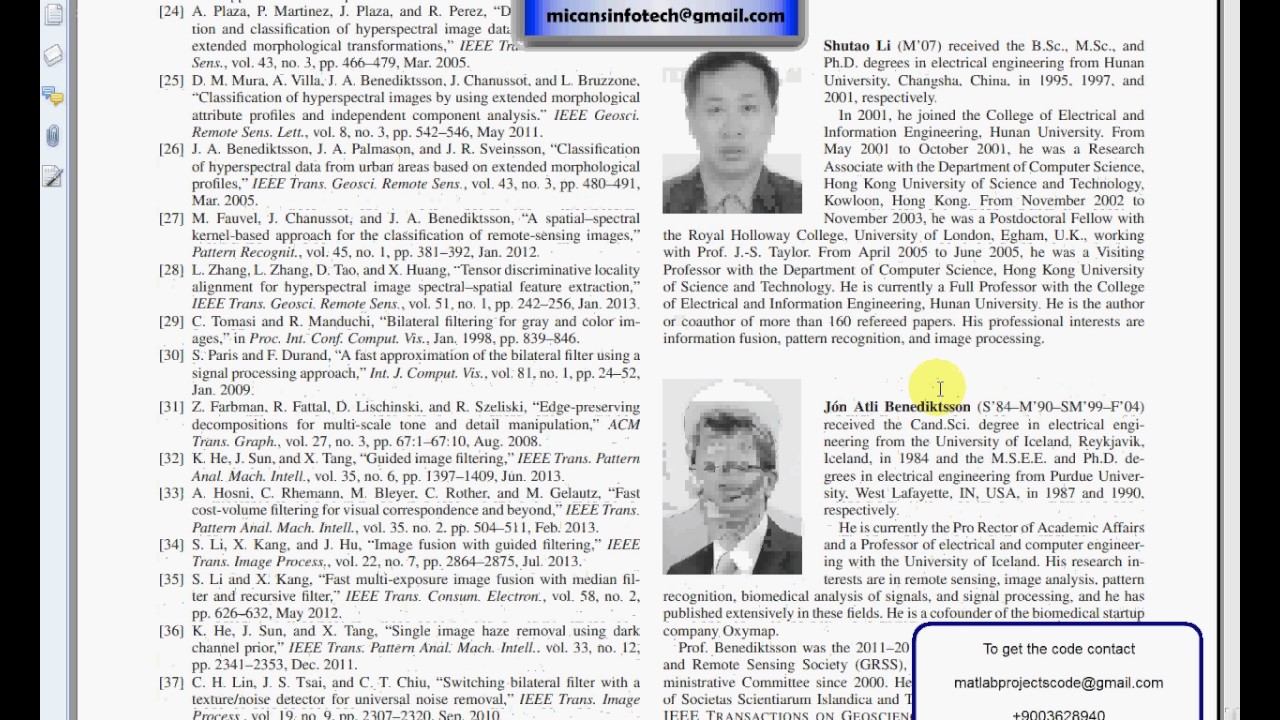
Показать описание
Abstract—Feature extraction is known to be an effective way in
both reducing computational complexity and increasing accuracy
of hyperspectral image classification. In this paper, a simple yet
quite powerful feature extraction method based on image fusion
and recursive filtering (IFRF) is proposed. First, the hyperspectral
image is partitioned into multiple subsets of adjacent hyperspectral
bands. Then, the bands in each subset are fused together by
averaging, which is one of the simplest image fusion methods.
Finally, the fused bands are processed with transform domain
recursive filtering to get the resulting features for classification.
Experiments are performed on different hyperspectral images,
with the support vector machines (SVMs) serving as the classifier.
By using the proposed method, the accuracy of the SVM classifier
can be improved significantly. Furthermore, compared with other
hyperspectral classification methods, the proposed IFRF method
shows outstanding performance in terms of classification accuracy and computational efficiency.
TO GET FULL SOURCE CODE
CONTACT
+91 90036 28940
both reducing computational complexity and increasing accuracy
of hyperspectral image classification. In this paper, a simple yet
quite powerful feature extraction method based on image fusion
and recursive filtering (IFRF) is proposed. First, the hyperspectral
image is partitioned into multiple subsets of adjacent hyperspectral
bands. Then, the bands in each subset are fused together by
averaging, which is one of the simplest image fusion methods.
Finally, the fused bands are processed with transform domain
recursive filtering to get the resulting features for classification.
Experiments are performed on different hyperspectral images,
with the support vector machines (SVMs) serving as the classifier.
By using the proposed method, the accuracy of the SVM classifier
can be improved significantly. Furthermore, compared with other
hyperspectral classification methods, the proposed IFRF method
shows outstanding performance in terms of classification accuracy and computational efficiency.
TO GET FULL SOURCE CODE
CONTACT
+91 90036 28940