filmov
tv
Probabilistic Machine Learning for Decision-making with 3D Geological Models - Florian Wellmann
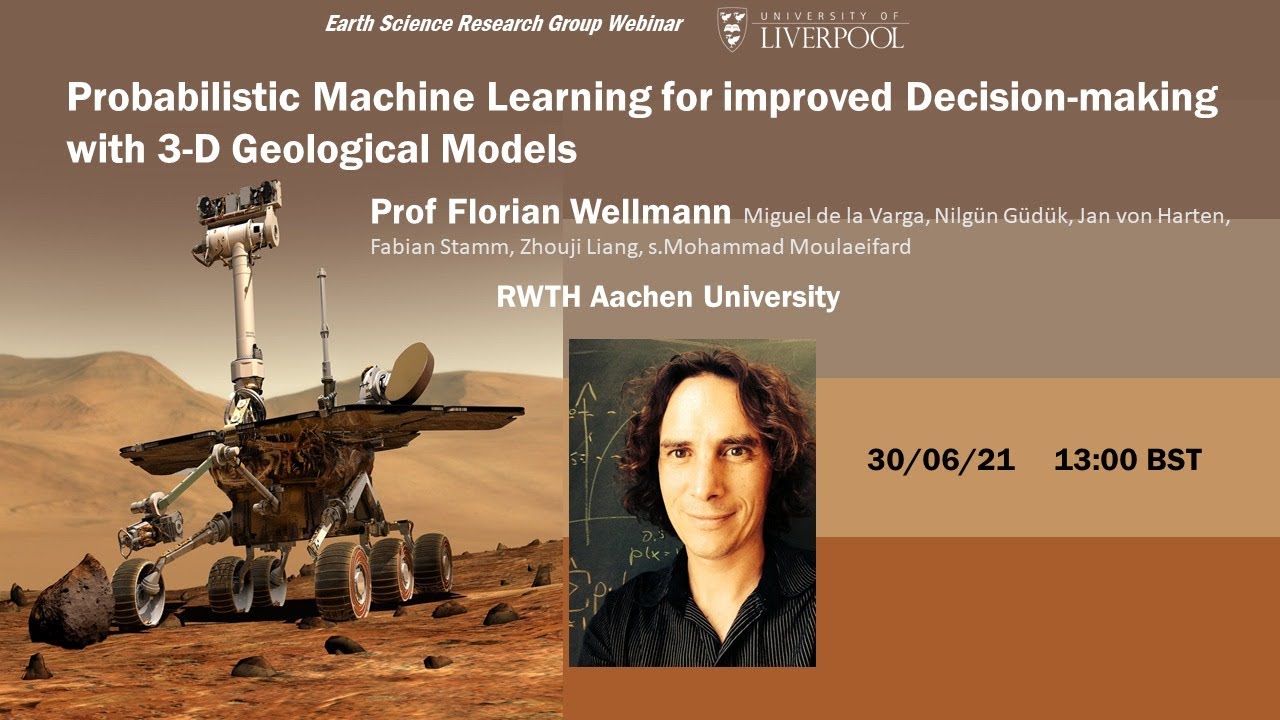
Показать описание
Geological models, as 3-D representations of subsurface structures, can be combined with gravity inversions to obtain geometric representations of geological objects with similar porperty distributions. These models are built on prior assumptions and imperfect information, and they often result from an integration of geological and geophysical data types with varying quality. These aspects result in uncertainties about the predicted subsurface structures and property distributions, which will affect the subsequent decision process.
We discuss approaches to evaluate uncertainties in geological models and to integrate geological and geophysical potential-field information in combined workflows. A first step is the consideration of uncertainties in prior model parameters on the basis of uncertainty propagation (forward uncertainty quantification). When applied to structural geological models with discrete classes, these methods result in a class probability for each point in space, often represented in tessellated grid cells.
A logical extension is the integration of geological forward operators into geophysical inverse frameworks, to enable a full flow of inference for a wider range of relevant parameters. We investigate here specifically the use of probabilistic machine learning tools in combination with geological and geophysical gravity and magnetic modeling. Challenges exist due to the hierarchical nature of the probabilistic models, but modern sampling strategies allow for efficient sampling in these complex settings. We showcase the application with examples combining geological modeling and geophysical potential field measurements in an integrated model for improved decision making.
We discuss approaches to evaluate uncertainties in geological models and to integrate geological and geophysical potential-field information in combined workflows. A first step is the consideration of uncertainties in prior model parameters on the basis of uncertainty propagation (forward uncertainty quantification). When applied to structural geological models with discrete classes, these methods result in a class probability for each point in space, often represented in tessellated grid cells.
A logical extension is the integration of geological forward operators into geophysical inverse frameworks, to enable a full flow of inference for a wider range of relevant parameters. We investigate here specifically the use of probabilistic machine learning tools in combination with geological and geophysical gravity and magnetic modeling. Challenges exist due to the hierarchical nature of the probabilistic models, but modern sampling strategies allow for efficient sampling in these complex settings. We showcase the application with examples combining geological modeling and geophysical potential field measurements in an integrated model for improved decision making.
Комментарии