filmov
tv
Learning Machines Seminar: Yoshua Bengio (Université de Montreal) / November 4, 2022
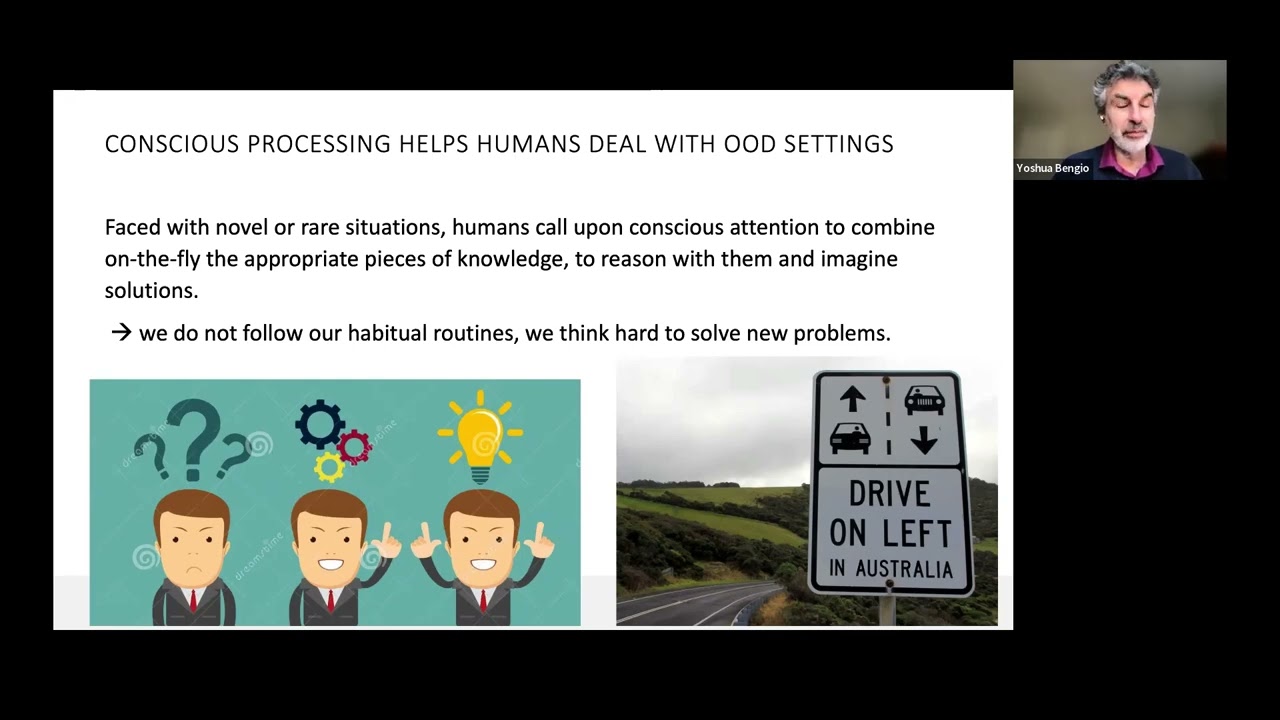
Показать описание
TITLE
Reusable Modular and Causal Knowledge Representation for Lifelong Learning
ABSTRACT
How can what has been learned on previous tasks generalize quickly to new tasks or changes in distribution? The study of conscious processing in human brains (and the window into it given by natural language) suggests that we are able to decompose high-level verbalizable knowledge into reusable components (roughly corresponding to words and phrases). This has stimulated research in modular neural networks where attention mechanisms can be used to dynamically select which modules should be brought to bear in a given new context. Another source of inspiration for tackling this challenge is the body of research into causality, where changes in tasks and distributions are viewed as interventions. The crucial insight is that we need to learn to separate (somewhat like in meta-learning) what is stable across changes in distribution, environments or tasks and what may be separate to each of them or changing in non-stationary ways in time. From a causal perspective what is stable are the reusable causal mechanisms, along with the inference machinery to make probabilistic guesses about the appropriate combination of mechanisms (maybe seen as a graph) in a particular new context. What may change with time are the interventions and other random variables which are those that yield more directly to observations. If interventions are not observed (we do not have labels for fully explaining the changes in tasks in terms of the underlying modules and causal variables) we would ideally like to estimate the Bayesian posterior over the interventions, given whatever is observed. This research approach raises many interesting research questions ranging from Bayesian inference and identifiability to causal discovery, representation learning and out-of-distribution generalization and adaptation, which will be discussed in the presentation.
BIO
Yoshua Bengio is a Full Professor in the Department of Computer Science and Operations Research at Université de Montreal, as well as the Founder and Scientific Director of Mila and the Scientific Director of IVADO. Considered one of the world’s leaders in artificial intelligence and deep learning, he is the recipient of the 2018 A.M. Turing Award with Geoff Hinton and Yann LeCun, known as the Nobel prize of computing. He is a Fellow of both the Royal Society of London and Canada, an Officer of the Order of Canada, Knight of the Legion of Honor of France, and a Canada CIFAR AI Chair.
Reusable Modular and Causal Knowledge Representation for Lifelong Learning
ABSTRACT
How can what has been learned on previous tasks generalize quickly to new tasks or changes in distribution? The study of conscious processing in human brains (and the window into it given by natural language) suggests that we are able to decompose high-level verbalizable knowledge into reusable components (roughly corresponding to words and phrases). This has stimulated research in modular neural networks where attention mechanisms can be used to dynamically select which modules should be brought to bear in a given new context. Another source of inspiration for tackling this challenge is the body of research into causality, where changes in tasks and distributions are viewed as interventions. The crucial insight is that we need to learn to separate (somewhat like in meta-learning) what is stable across changes in distribution, environments or tasks and what may be separate to each of them or changing in non-stationary ways in time. From a causal perspective what is stable are the reusable causal mechanisms, along with the inference machinery to make probabilistic guesses about the appropriate combination of mechanisms (maybe seen as a graph) in a particular new context. What may change with time are the interventions and other random variables which are those that yield more directly to observations. If interventions are not observed (we do not have labels for fully explaining the changes in tasks in terms of the underlying modules and causal variables) we would ideally like to estimate the Bayesian posterior over the interventions, given whatever is observed. This research approach raises many interesting research questions ranging from Bayesian inference and identifiability to causal discovery, representation learning and out-of-distribution generalization and adaptation, which will be discussed in the presentation.
BIO
Yoshua Bengio is a Full Professor in the Department of Computer Science and Operations Research at Université de Montreal, as well as the Founder and Scientific Director of Mila and the Scientific Director of IVADO. Considered one of the world’s leaders in artificial intelligence and deep learning, he is the recipient of the 2018 A.M. Turing Award with Geoff Hinton and Yann LeCun, known as the Nobel prize of computing. He is a Fellow of both the Royal Society of London and Canada, an Officer of the Order of Canada, Knight of the Legion of Honor of France, and a Canada CIFAR AI Chair.