filmov
tv
Yoshua Bengio – Cognitively-inspired inductive biases for higher-level cognition
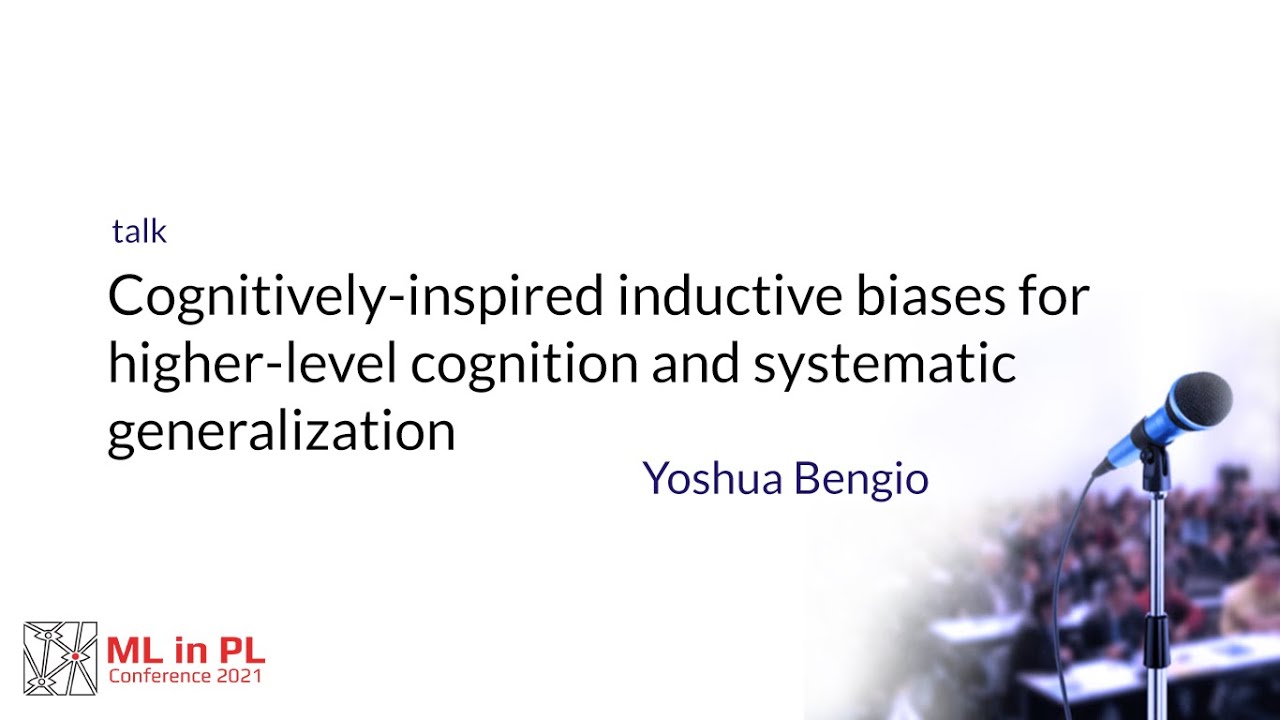
Показать описание
Yoshua Bengio – Cognitively-inspired inductive biases for higher-level cognition and systematic generalization
How can deep learning be extended to encompass the kind of high-level cognition and reasoning that humans enjoy and that seems to provide us with stronger out-of-distribution generalization than current state-of-the-art AI? Looking into neuroscience and cognitive science and translating these observations and theories into machine learning, we propose an initial set of inductive biases for representations, computations and probabilistic dependency structure. The Global Workspace Theory in particular suggests an important role for a communication bottleneck through a working memory, and this may impose a form of sparsity on the high-level dependencies. These inductive biases also strongly tie the notion of representation with that of actions, interventions and causality, possibly giving a key to stronger identifiability of latent causal structure and ensuing better sample complexity in and out of distribution, as well as meta-cognition abilities facilitating exploration that seeks to reduce epistemic uncertainty of the underlying causal understanding of the environment.
How can deep learning be extended to encompass the kind of high-level cognition and reasoning that humans enjoy and that seems to provide us with stronger out-of-distribution generalization than current state-of-the-art AI? Looking into neuroscience and cognitive science and translating these observations and theories into machine learning, we propose an initial set of inductive biases for representations, computations and probabilistic dependency structure. The Global Workspace Theory in particular suggests an important role for a communication bottleneck through a working memory, and this may impose a form of sparsity on the high-level dependencies. These inductive biases also strongly tie the notion of representation with that of actions, interventions and causality, possibly giving a key to stronger identifiability of latent causal structure and ensuing better sample complexity in and out of distribution, as well as meta-cognition abilities facilitating exploration that seeks to reduce epistemic uncertainty of the underlying causal understanding of the environment.
Yoshua Bengio – Cognitively-inspired inductive biases for higher-level cognition
Yoshua Bengio: System 2 GFlowNets: Induction Biases Towards Machines that Understand and Think
HLFF Shortcuts: Yoshua Bengio
[SAIF 2020] Day 1: Towards Discovering Casual Representations - Yoshua Bengio | Samsung
MoroccoAI Annual Conference 2021 - Honorary Keynote - Prof. Yoshua Bengio
Deep learning of representations for conscious cognition - Yoshua Bengio (Université de Montréal)
S3 E1 Turing Award Winner Yoshua Bengio: Equipping AI with Higher Level Cognition and Creativity
Séminaire DIC-ISC-CRIA du 17 février 2022 avec Yoshua Bengio
Priors for Semantic Variables - Yoshua Bengio
Deep Learning for AI, Yoshua Bengio
Deep Learning for Higher-Level Cognition
Yoshua Bengio - Towards Neural Nets for Conscious Processing and Causal Reasoning
AI for Ukraine - Talk from Professor Yoshua Bengio
TechAide Cafe with Yoshua Bengio on Role of Machine Learning to Understand Consciousness
Yoshua Bengio: Meta-learning (NeurIPS 2019)
#063 - Prof. YOSHUA BENGIO - GFlowNets, Consciousness & Causality
Rockwood Memorial Lecture 2021 - Yoshua Bengio, Ph.D., Mila, Université de Montréal (7/26/21)
Yoshua Bengio - How Could We Design Aligned & Provably Safe Al?
Yoshua Bengio: Symbolic AI in Contrast to Deep Learning (NeurIPS 2019)
Yoshua Bengio et al: What is out of distribution (OOD) generalization & why is it important? Neu...
Fireside Chat with Yoshua Bengio
Prof. Yoshua Bengio | From System 1 Deep Learning to System 2 Deep Learning | NeurIPS 2019
Séminaire DIC-ISC-CRIA - 17 février 2022 avec Yoshua BENGIO
MSR-IISc AI Seminar Series: GFlowNets and System 2 Deep Learning - Yoshua Bengio
Комментарии