filmov
tv
Few Shot Learning - EXPLAINED!
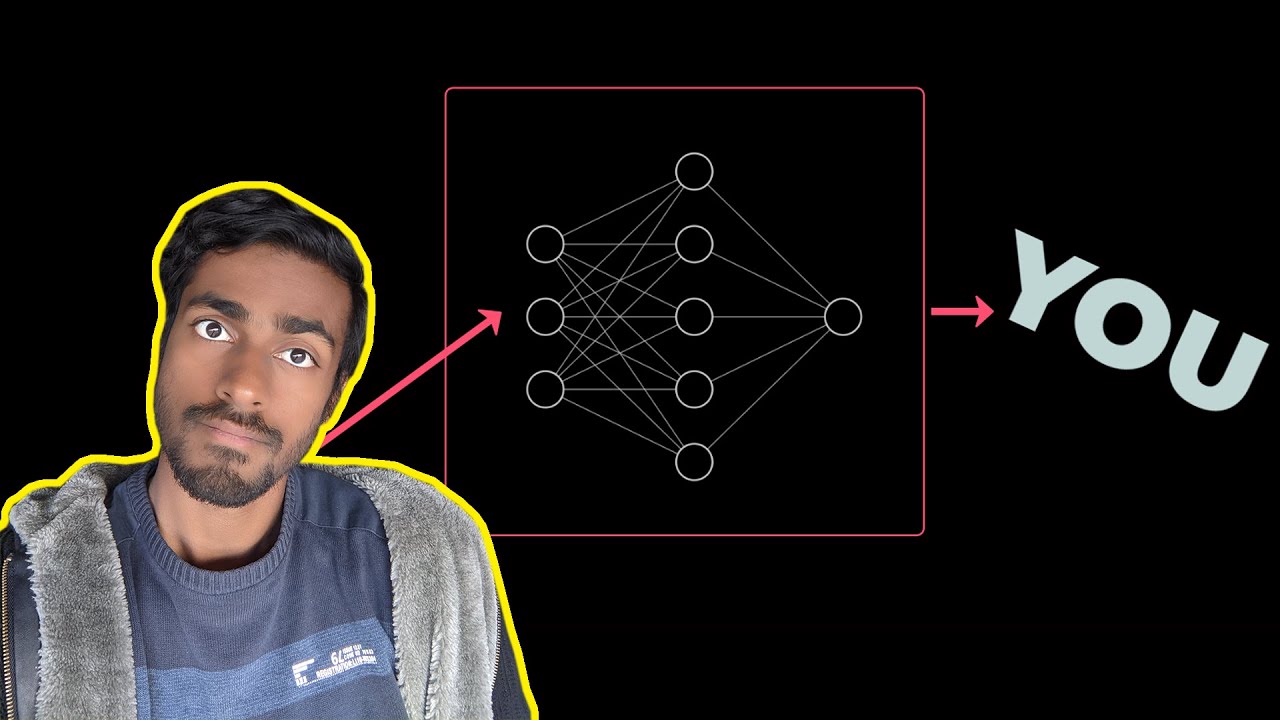
Показать описание
TIMESTAMPS
0:00 Case Study
1:37 Prior Knowledge
3:07 Disadvantage of Traditional Models
4:57 The new Model – explained
6:40 Math
9:04 Code
REFERENCES
Few Shot Learning - EXPLAINED!
Few-Shot Learning (1/3): Basic Concepts
What is Zero-Shot Learning?
Zero-shot, One-shot and Few-shot Prompting Explained | Prompt Engineering 101
Episode 57: Few-Shot Learning Explained
Few Shot Learning with Code - Meta Learning - Prototypical Networks
[Few-shot learning][2.2] Prototypical Networks: intuition, algorithm, pytorch code
C4W4L02 One Shot Learning
AI First Principles: Effective Communication & Collaboration with AI tools
Multimodal Few-Shot Learning with Frozen Language Models | Paper Explained
Few-shot and Zero-shot Learning - Part 01
All Machine Learning algorithms explained in 17 min
Few-Shot Prompting Explained
Few-shot Learning Explained (FSL): AI with Minimal Data
few shot, zero shot learning explained #deeplearning #artificialintelligence #shorts
Few-Shot Learning Through an Information Retrieval Lens | TDLS
Few-shot Learning | Lecture 72 (Part 2) | Applied Deep Learning (Supplementary)
Corporate-Talk Chatbot: Few-Shot Learning, Large Language Models Explained Prompt Engineering Course
Mengye Ren - Towards Continual and Compositional Few-shot Learning
Zero-Shot Learning - Dr. Timothy Hospedales
FEW-SHOT LEARNING | Basic concepts | Introduction to Natural Language Processing (NLP) | 02
Master the Perfect ChatGPT Prompt Formula (in just 8 minutes)!
Learning What Not To Segment: A New Perspective on Few Shot Segmentation | CVPR 2022
Meta-Learning of Neural Architectures for Few-Shot Learning
Комментарии