filmov
tv
Mengye Ren - Towards Continual and Compositional Few-shot Learning
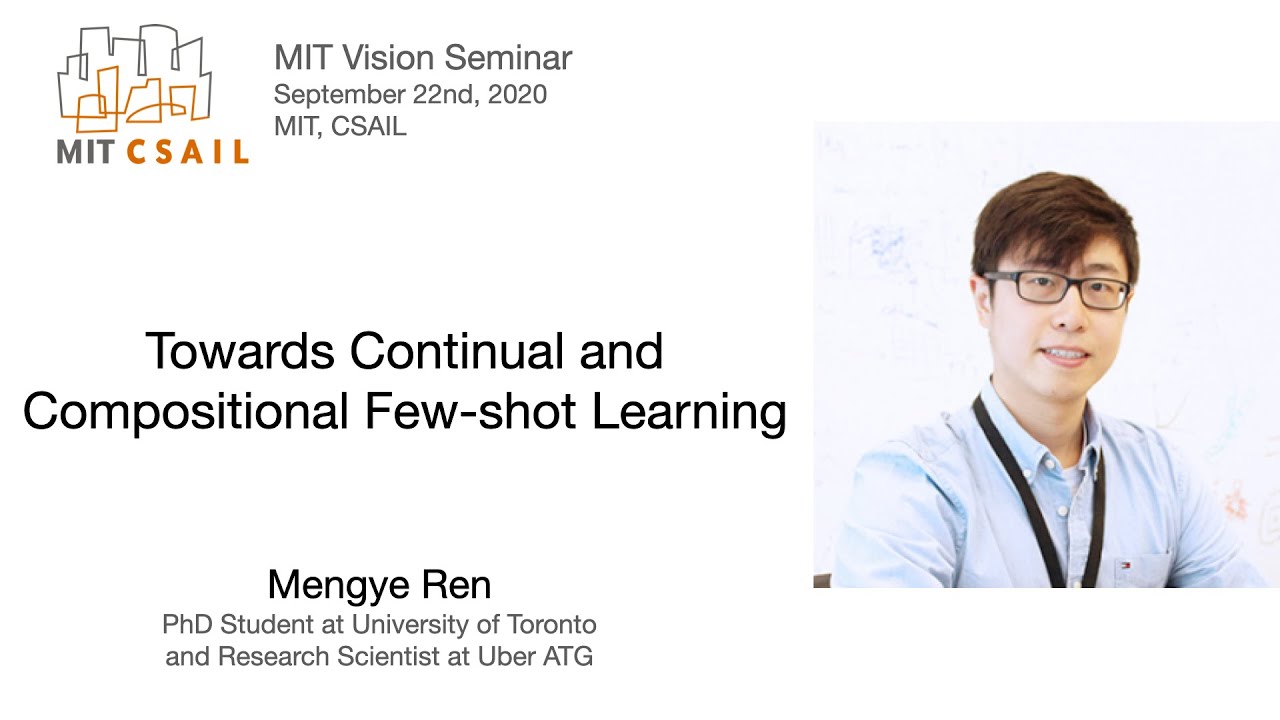
Показать описание
September 22, 2020. MIT CSAIL
Abstract: Few-shot learning has recently emerged as a popular area of research towards building more flexible machine learning programs that can adapt at test time. However, it now faces two major criticisms. First, the “k-shot n-way” episodic structure is still far from modelling the incremental knowledge acquisition procedure of an agent in a natural environment. Secondly, there has been limited improvement towards modelling compositional understanding of novel objects; on the other hand, features obtained from regular classification tasks can perform very well. In this talk I will introduce two recent advances that address each of the challenges above.
Bio: Mengye Ren is a PhD student in the machine learning group of the Department of Computer Science at the University of Toronto. His academic advisor is Prof. Richard Zemel. He also works as a research scientist at Uber Advanced Technologies Group (ATG) Toronto, directed by Prof. Raquel Urtasun, doing self-driving related research. His research interests are meta-learning, few-shot learning, and continual learning. He studied undergrad in Engineering Science at the University of Toronto.
Abstract: Few-shot learning has recently emerged as a popular area of research towards building more flexible machine learning programs that can adapt at test time. However, it now faces two major criticisms. First, the “k-shot n-way” episodic structure is still far from modelling the incremental knowledge acquisition procedure of an agent in a natural environment. Secondly, there has been limited improvement towards modelling compositional understanding of novel objects; on the other hand, features obtained from regular classification tasks can perform very well. In this talk I will introduce two recent advances that address each of the challenges above.
Bio: Mengye Ren is a PhD student in the machine learning group of the Department of Computer Science at the University of Toronto. His academic advisor is Prof. Richard Zemel. He also works as a research scientist at Uber Advanced Technologies Group (ATG) Toronto, directed by Prof. Raquel Urtasun, doing self-driving related research. His research interests are meta-learning, few-shot learning, and continual learning. He studied undergrad in Engineering Science at the University of Toronto.