filmov
tv
Maximum Likelihood Estimator of The Mean of The Normal Distribution
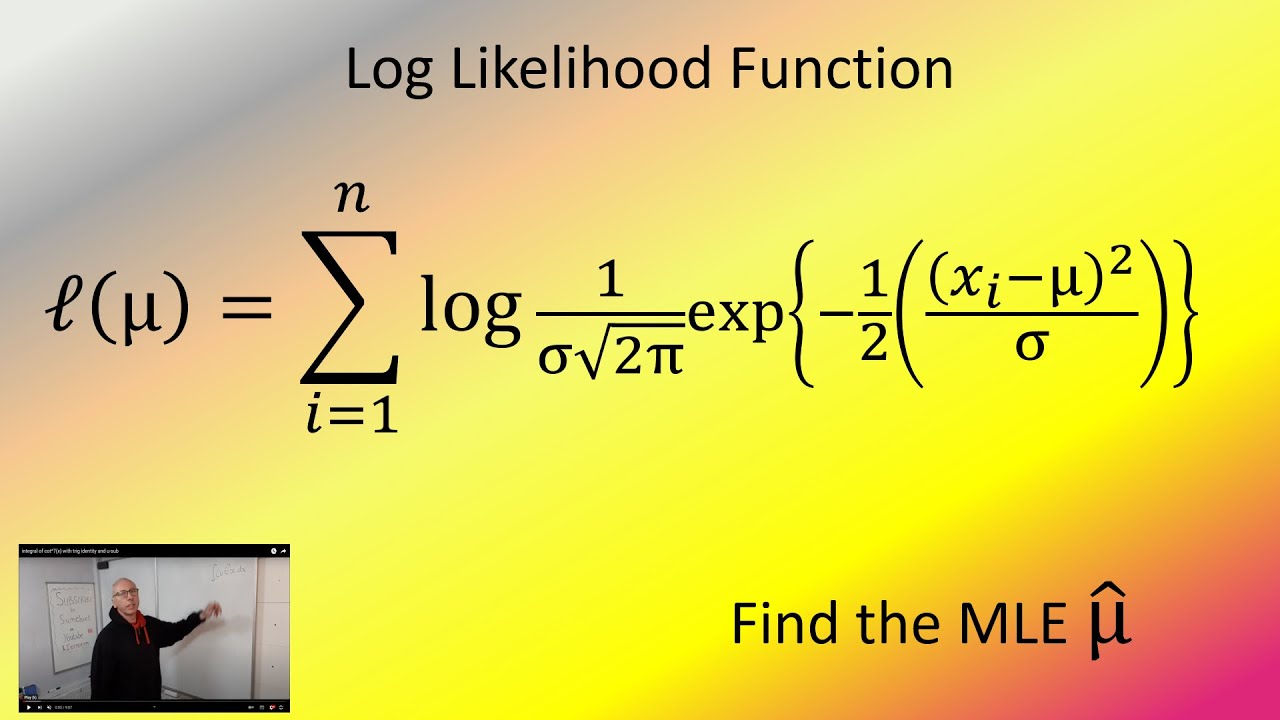
Показать описание
Statistics and Bayesian Statistics and Point Estimation.
This video shows how to find the candidate for the Maximum Likelihood Estimate of mu from the Normal Distribution otherwise known as the mean.
Next, we find the log likelihood function with respect to mu. This will be easier to differentiate in the next few stages.
The log likelihood is then differentiated with respect to mu, and we set l'(mu)=0.
This is for x greater than 0 and mu is greater than 0
This gives us our candidate for the MLE of mu. also, known as hat mu.
To check if this is a suitable candidate, we take the second derivative of the log likelihood function and see that it is less than zero always. If so, it is a suitable candidate.
#statistics
#logarithm
#discretemaths
#probabilityandstatistics
#stats
#probability_distribution
#bernoulli
#binomial_distribution
#poissondistribution
#exponential
there is an excellent article on the Normal Distribution here
The simplest case of a normal distribution is known as the standard normal distribution or unit normal distribution. This is a special case when mu =0 and sigma =1, and it is described by this probability density function (or density):
The variable z has a mean of 0 and a variance and standard deviation of 1.
Although the density above is most commonly known as the standard normal, a few authors have used that term to describe other versions of the normal distribution. Carl Friedrich Gauss, for example, the probability density of the standard Gaussian distribution (standard normal distribution, with zero mean and unit variance) is often denoted with the Greek letter phi. The alternative form of the Greek letter phi is also used quite often.
About 68% of values drawn from a normal distribution are within one standard deviation σ away from the mean; about 95% of the values lie within two standard deviations; and about 99.7% are within three standard deviations. This fact is known as the 68-95-99.7 (empirical) rule, or the 3-sigma rule.
This video shows how to find the candidate for the Maximum Likelihood Estimate of mu from the Normal Distribution otherwise known as the mean.
Next, we find the log likelihood function with respect to mu. This will be easier to differentiate in the next few stages.
The log likelihood is then differentiated with respect to mu, and we set l'(mu)=0.
This is for x greater than 0 and mu is greater than 0
This gives us our candidate for the MLE of mu. also, known as hat mu.
To check if this is a suitable candidate, we take the second derivative of the log likelihood function and see that it is less than zero always. If so, it is a suitable candidate.
#statistics
#logarithm
#discretemaths
#probabilityandstatistics
#stats
#probability_distribution
#bernoulli
#binomial_distribution
#poissondistribution
#exponential
there is an excellent article on the Normal Distribution here
The simplest case of a normal distribution is known as the standard normal distribution or unit normal distribution. This is a special case when mu =0 and sigma =1, and it is described by this probability density function (or density):
The variable z has a mean of 0 and a variance and standard deviation of 1.
Although the density above is most commonly known as the standard normal, a few authors have used that term to describe other versions of the normal distribution. Carl Friedrich Gauss, for example, the probability density of the standard Gaussian distribution (standard normal distribution, with zero mean and unit variance) is often denoted with the Greek letter phi. The alternative form of the Greek letter phi is also used quite often.
About 68% of values drawn from a normal distribution are within one standard deviation σ away from the mean; about 95% of the values lie within two standard deviations; and about 99.7% are within three standard deviations. This fact is known as the 68-95-99.7 (empirical) rule, or the 3-sigma rule.
Комментарии