filmov
tv
The Unexpected Power of Orthogonal Matrices
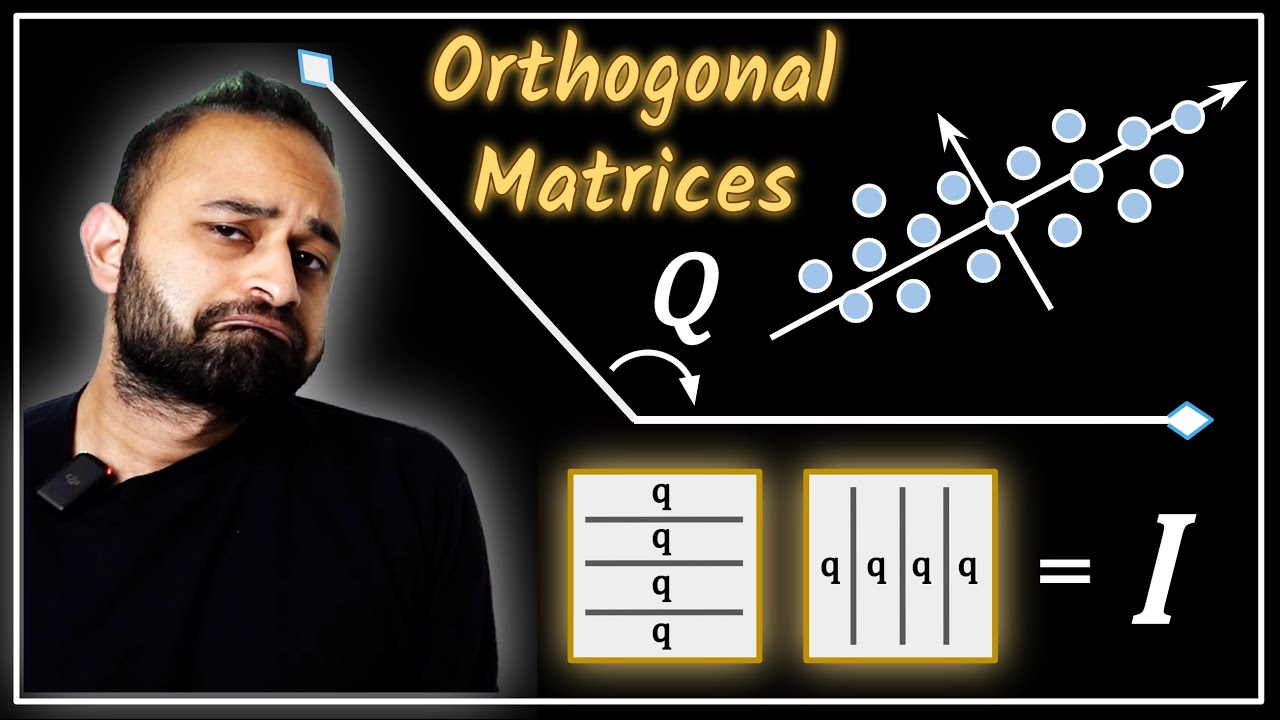
Показать описание
0:00 Intro
1:17 Definition
3:28 Inverse Convenience
6:31 Types of Orthogonal Transformations
10:11 Application to PCA
NOTE: I meant to say "orthogonal" instead of "linearly independent" in the verbal definition. Orthogonal means that the dot product between two vectors is 0 but linear independence means that one vector cannot be expressed as a linear combination of another vector. Thanks to some viewers for pointing this out.
The Unexpected Power of Orthogonal Matrices
Proof: Orthogonal Matrices Satisfy A^TA=I
Orthogonal matrices preserve norm
Salsa Night in IIT Bombay #shorts #salsa #dance #iit #iitbombay #motivation #trending #viral #jee
This chapter closes now, for the next one to begin. 🥂✨.#iitbombay #convocation
IIT Bombay CSE 😍 #shorts #iit #iitbombay
Orthogonal Matrices - Geometric Interpretation
Orthogonal Matrices — Topic 24 of Machine Learning Foundations
SET OF ALL ORTHOGONAL MATRICES FORM A GROUP?
Visualize Different Matrices part2 | SEE Matrix, Chapter 1
IIT Bombay Lecture Hall | IIT Bombay Motivation | #shorts #ytshorts #iit
Before JEE vs After JEE 😍 | My Transformation💔 | IIT Motivation|Jee 2023 #transformation #iit #viral...
Mrsir in pw patna vidyapeeth ||Pw vidyapeeth | #mrsir #mrsirphysics#pwvidyapeeth #pw
CRISPR's Next Advance Is Bigger Than You Think | Jennifer Doudna | TED
@ALLENCareerInstituteofficial reply to @PhysicsWallah #shorts #jee2023 #iitjeemotivation
Linear Algebra 7.1 Orthogonal Matrices
How (and why) to raise e to the power of a matrix | DE6
unexpected neet result #physicswallah #yakeen2.0 #competitionwallah
Dear linear algebra students, This is what matrices (and matrix manipulation) really look like
Shradha didi at lpu 🤩 #apna college #viralshorts
Francesco Mezzadri: Moments of Random Matrices and Hypergeometric Orthogonal Polynomials
JEE Aspirants ka Sach 💔 #JEE #JEEMain #Shorts
Three-dimensional linear transformations | Chapter 5, Essence of linear algebra
Orthogonal Frequency division Multiplexing
Комментарии