filmov
tv
Peter Dueben: Machine learning for weather predictions
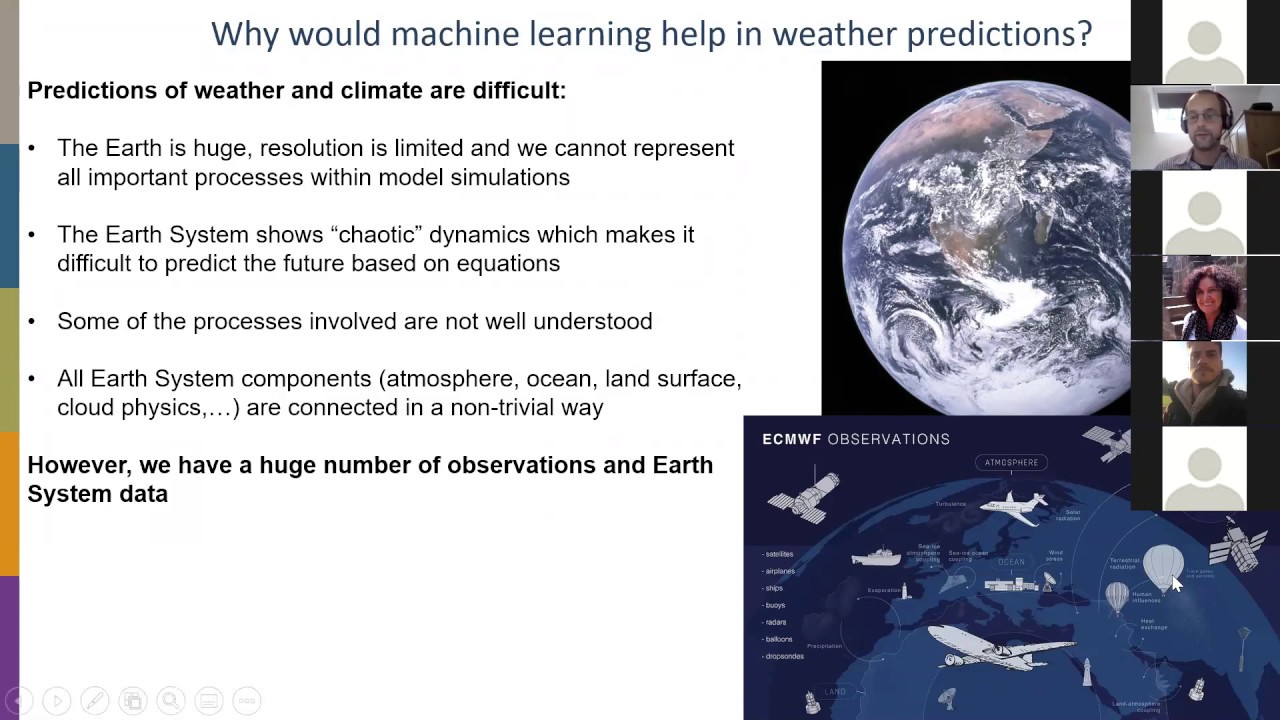
Показать описание
This talk will outline how machine learning, and in particular deep learning, could help to improve weather predictions in the coming years and present an overview on work on machine learning methods that is ongoing at the European Centre for Medium-Range Weather Forecasts. To predict the weather requires to model the Earth System -- a huge system that consists of many individual components and shows chaotic behaviour for which conventional tools are often struggling to provide satisfying results. On the other hand, a huge amount of data is available from both observations and modelling. Therefore, a large number of machine learning applications are currently tested in order to improve the different components across the workflow of numerical weather predictions. However, whether these approaches will succeed is still unclear as there are also a number of challenges for the application of machine learning tools in weather predictions that will be discussed in the talk.
Peter is the Coordinator of machine learning and AI activities at the European Centre for Medium-Range Weather Forecasts (ECMWF) and holds a University Research Fellowship of the Royal Society that allows him to follow his research interests in the area of numerical weather and climate modelling, machine learning, and high-performance computing. Before moving to ECMWF, he wrote his PhD thesis at the Max-Planck Institute for Meteorology in Hamburg, Germany, on the development of a finite element dynamical core for Earth System models. During the subsequent Postdoctoral Position with Professor Tim Palmer at the University of Oxford, he was focusing on the study of reduced numerical precision to speed- up simulations of Earth System models. During this time, he has written one of the first papers to test the use of Field Programmable Gate Arrays (FPGAs) in atmospheric applications, published the first paper on an approach to secure atmosphere models against hardware faults, and developed the first version of the weather forecast model IFS of ECMWF that was able to run in single precision instead of the double precision default. This change in precision is generating a reduction of runtime of forecast simulations by 40% and is currently prepared for operational weather forecasts at ECMWF. He is a work-package leader of the ESiWACE 2 Centre of Excellence, a co-author of the ESCAPE 2 project and Co-Pi on an INCITE grant that provides access to the fastest supercomputer in the world, Summit.
Peter is the Coordinator of machine learning and AI activities at the European Centre for Medium-Range Weather Forecasts (ECMWF) and holds a University Research Fellowship of the Royal Society that allows him to follow his research interests in the area of numerical weather and climate modelling, machine learning, and high-performance computing. Before moving to ECMWF, he wrote his PhD thesis at the Max-Planck Institute for Meteorology in Hamburg, Germany, on the development of a finite element dynamical core for Earth System models. During the subsequent Postdoctoral Position with Professor Tim Palmer at the University of Oxford, he was focusing on the study of reduced numerical precision to speed- up simulations of Earth System models. During this time, he has written one of the first papers to test the use of Field Programmable Gate Arrays (FPGAs) in atmospheric applications, published the first paper on an approach to secure atmosphere models against hardware faults, and developed the first version of the weather forecast model IFS of ECMWF that was able to run in single precision instead of the double precision default. This change in precision is generating a reduction of runtime of forecast simulations by 40% and is currently prepared for operational weather forecasts at ECMWF. He is a work-package leader of the ESiWACE 2 Centre of Excellence, a co-author of the ESCAPE 2 project and Co-Pi on an INCITE grant that provides access to the fastest supercomputer in the world, Summit.
Комментарии