filmov
tv
Accelerating the ML Lifecycle with an Enterprise-Grade Feature Store
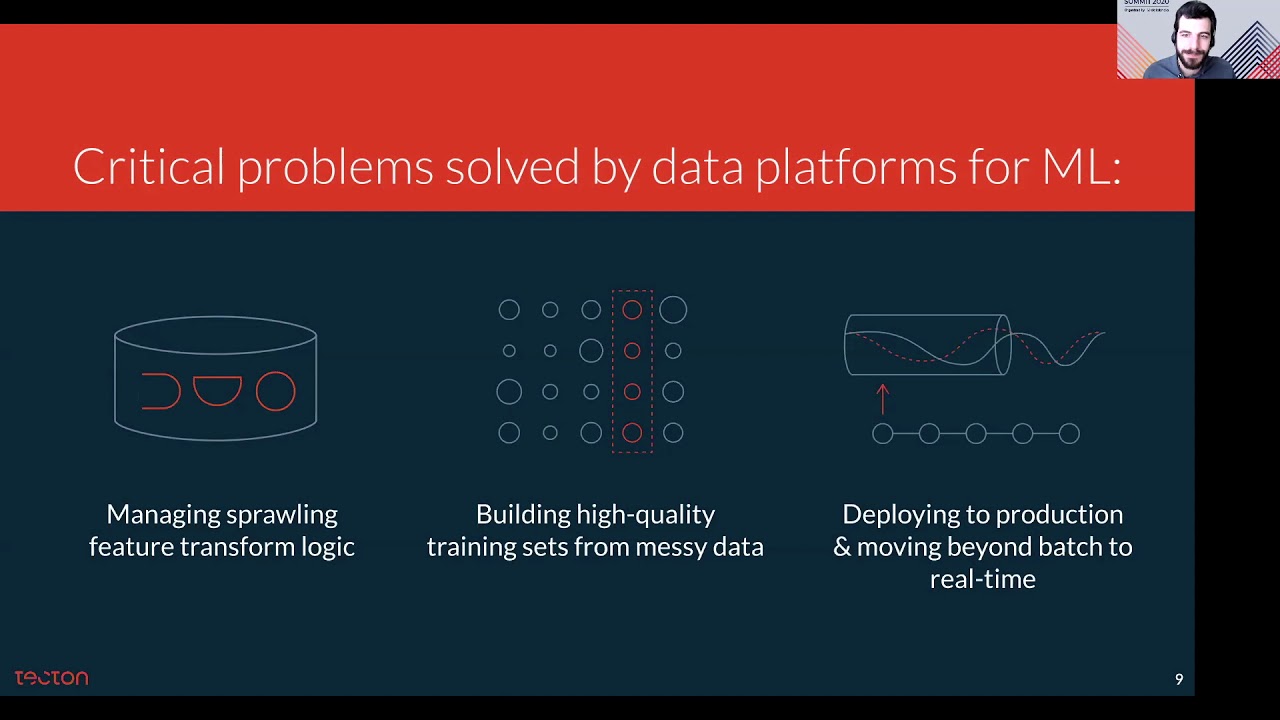
Показать описание
Productionizing real-time ML models poses unique data engineering challenges for enterprises that are coming from batch-oriented analytics. Enterprise data, which has traditionally been centralized in data warehouses and optimized for BI use cases, must now be transformed into features that provide meaningful predictive signals to our ML models. Enterprises face the operational challenges of deploying these features in production: building the data pipelines, then processing and serving the features to support production models. ML data engineering is a complex and brittle process that can consume upwards of 80% of our data science efforts, all too often grinding ML innovation to a crawl.
Atlassian will join us to provide first-hand perspective from an enterprise who has successfully deployed a feature platform in production. The platform powers real-time, ML-driven personalization and search services for a popular SaaS application.
Connect with us:
Atlassian will join us to provide first-hand perspective from an enterprise who has successfully deployed a feature platform in production. The platform powers real-time, ML-driven personalization and search services for a popular SaaS application.
Connect with us:
Accelerating the ML Lifecycle with an Enterprise-Grade Feature Store
Accelerate the ML Lifecycle with Unravel and Databricks
Accelerating the Machine Learning Lifecycle with MLflow 1.0 | M. Zaharia, A. Davidson, G. Buehrer
What's New in MLflow: a System to Accelerate the Machine Learning Lifecycle.
Managing your ML lifecycle with Azure Databricks and Azure ML - BRK3010
Learn to Use Databricks for the Full ML Lifecycle
Accelerating Production Machine Learning with MLflow - Matei Zaharia (Databricks)
Top Considerations for Accelerating AI/ML Lifecycle in the Cloud-Native Era
7.1 ML Lifecycle
How to Accelerate the End-to-End Machine Learning Lifecycle on Azure
Managing the ML Lifecycle with MLflow | On-Demand Webinar | Watch On-Demand
Managing the Complete Machine Learning Lifecycle with MLflow continues
Machine Learning Product Development LifeCycle
MLflow: Accelerating the Machine Learning Lifecycle by Matei Zaharia
End to End ML Lifecycle with Databricks
XLDB-2019: Accelerating the Machine Learning Lifecycle with MLflow
Managing the Machine Learning Lifecycle with MLflow and R with Kevin Kuo (RStudio)
MLOps: Accelerating Data Science with DevOps - Microsoft
MLflow Infrastructure for the Complete ML Lifecycle Matei Zaharia Databricks
AstraZeneca: Accelerating Drug Discovery with Machine Learning and AI on Cambridge-1
Managing your ML lifecycle with Azure Databricks and Azure Machine Learning | OD210
MLOps for managing the end to end life cycle with Azure Machine Learning service
DSPT Tutorial #2 - Managing the ML lifecycle with Mlflow
Machine Learning Lifecycle Made Easy with MLflow
Комментарии