filmov
tv
Seminar: Physics informed neural networks for modelling respiratory system
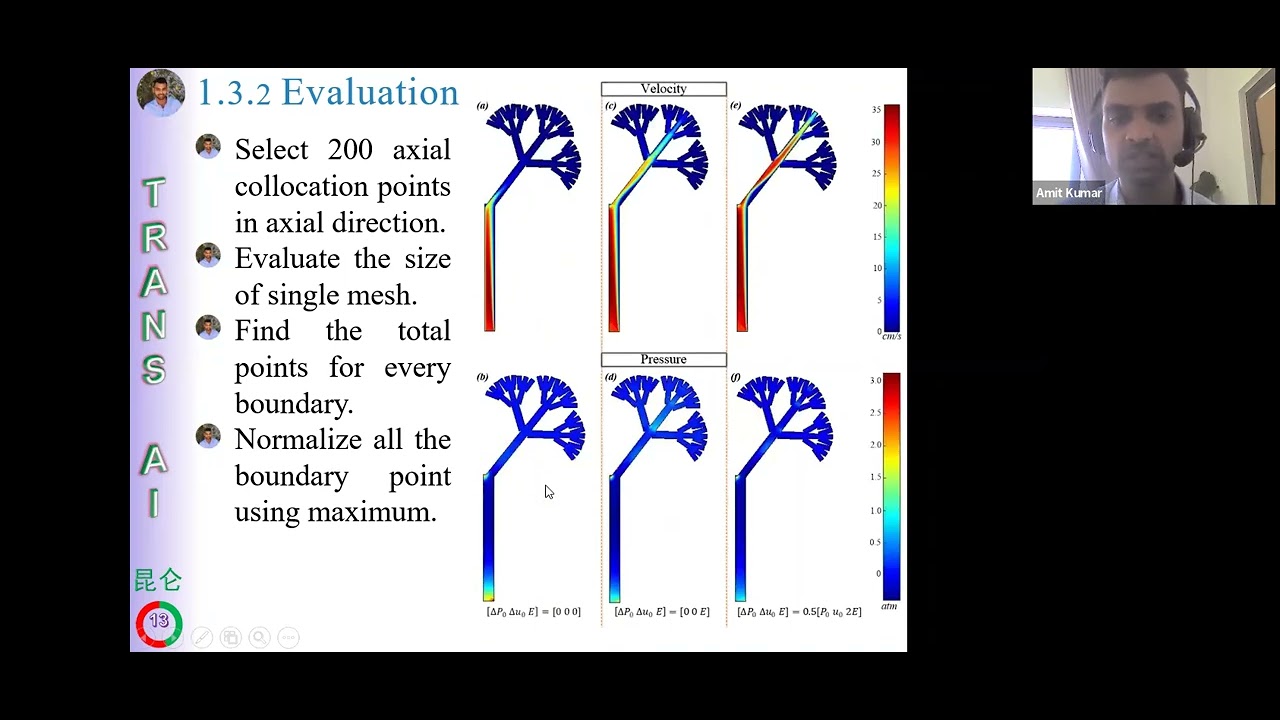
Показать описание
Abstract:
Clinical decision support systems play a vital role in providing health care to respiratory patients. Respiratory impedance is one of the decisive health indicators. A respiratory impedance model provides relevant information to support the CDSS. The respiratory systems are governed by complex laws of physics. Physics-informed neural networks (PINNs) can embed the knowledge of partial differential equations of physical laws that govern a system during the model training process. PINNs are faster than the traditionally used numerical methods. PINNs is integrated with an entangled ladder network for modelling the inhalation impedance of the conduction zone of the pulmonary airways. Entanglement is inherited to simplify the complex structure of airways. The entangled ladder network approximates the overall impedance of the conduction zone with application of a finite series continued fractions. The impedance is evaluated for 9 sets of initial conditions with varying pressure, velocity, and elasticity. The results are compared with the Forced oscillation test and it is found that the model closely resembles the FOT values for restrictive conditions. It also provides the experimental threshold of flow parameters that reduce the impedance within the lungs without exceeding the fatigue condition. Gradually decreasing the initial velocity by 20% yields low impedance for adults with 75% total lung capacity. The impedance can be further lowered progressive increase in the pressure by 20%. The increased respiratory flow reduced the dead-space in the lungs .
Presenter Biography:
Time 5pm (Sydney time), Friday 17th March 2023
Location: Red Centre Building, Rm 4082
Group: Transitional Artificial Intelligence Research Group, School of Mathematics and Statistics, UNSW Sydney
Clinical decision support systems play a vital role in providing health care to respiratory patients. Respiratory impedance is one of the decisive health indicators. A respiratory impedance model provides relevant information to support the CDSS. The respiratory systems are governed by complex laws of physics. Physics-informed neural networks (PINNs) can embed the knowledge of partial differential equations of physical laws that govern a system during the model training process. PINNs are faster than the traditionally used numerical methods. PINNs is integrated with an entangled ladder network for modelling the inhalation impedance of the conduction zone of the pulmonary airways. Entanglement is inherited to simplify the complex structure of airways. The entangled ladder network approximates the overall impedance of the conduction zone with application of a finite series continued fractions. The impedance is evaluated for 9 sets of initial conditions with varying pressure, velocity, and elasticity. The results are compared with the Forced oscillation test and it is found that the model closely resembles the FOT values for restrictive conditions. It also provides the experimental threshold of flow parameters that reduce the impedance within the lungs without exceeding the fatigue condition. Gradually decreasing the initial velocity by 20% yields low impedance for adults with 75% total lung capacity. The impedance can be further lowered progressive increase in the pressure by 20%. The increased respiratory flow reduced the dead-space in the lungs .
Presenter Biography:
Time 5pm (Sydney time), Friday 17th March 2023
Location: Red Centre Building, Rm 4082
Group: Transitional Artificial Intelligence Research Group, School of Mathematics and Statistics, UNSW Sydney