filmov
tv
Episode 3: From PyTorch to PyTorch Lightning
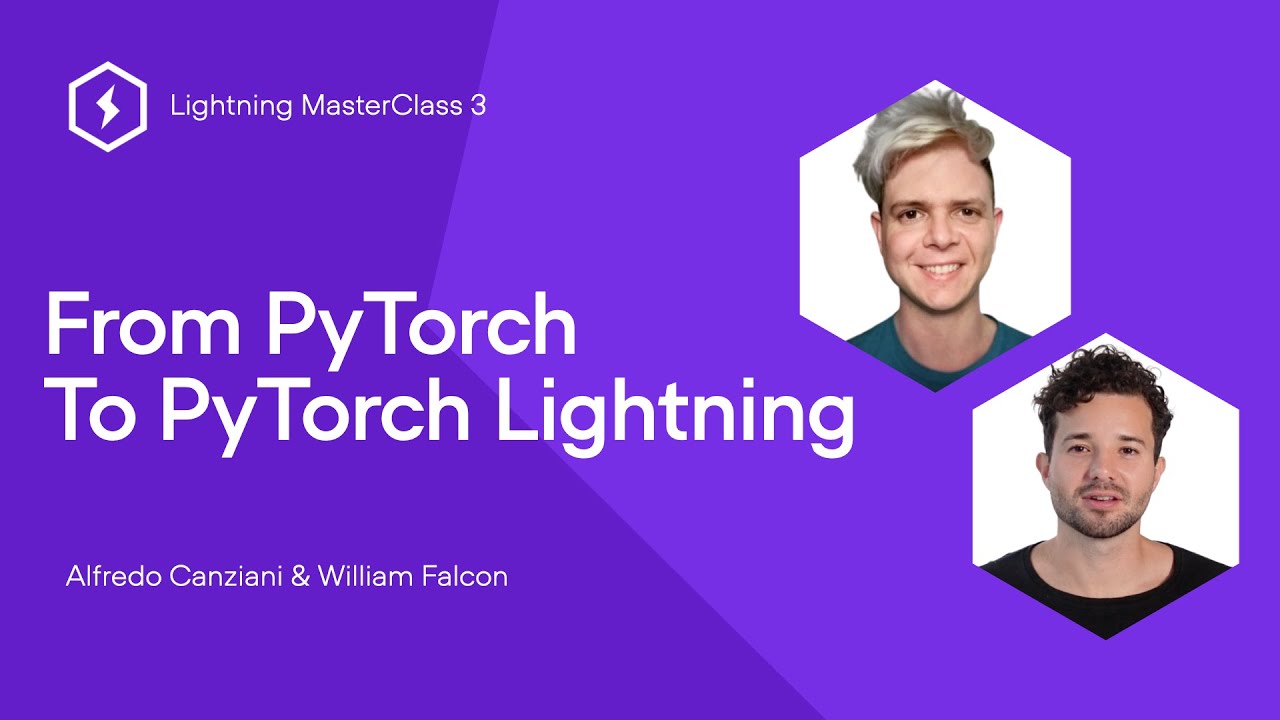
Показать описание
This video covers the magic of PyTorch Lightning! We convert the pure PyTorch classification model we created in the previous episode to PyTorch Lightning, which makes all the latest AI best practices trivial. We go over training on single and multi GPUs, logging and saving models, and many more!
Willam Falcon is an AI Ph.D. researcher at NYU, and creator and founder of PyTorch Lightning
Chapters:
00:00 Introduction to PyTorch Lightning
00:38 Install PyTorch Lightning
01:03 5 main components of a Lightning Module
01:47 Defining a model
04:05 Optimizer
05:20 The Training Loop
07:26 Loading and preparing data
09:10 Running training experiments
16:04 Training on a GPU
17:35 Logging and saving models
23:02 Validation loop
31:48 Multi GPU training
Episode 3: From PyTorch to PyTorch Lightning
What is backpropagation really doing? | Chapter 3, Deep learning
PyTorch Lightning Community Talks - Episode 3
PyTorch vs TensorFlow | Ishan Misra and Lex Fridman
G AI - Episode - 3 - Session - 1 - What is pytorch
G AI - Episode - 3 - Lesson - 2 - Demystifying torch.nn - The Backbone of Pytorch
Converting from pytorch to pytorch lightning in 4 minutes
Mr. Robot Sucks
The age old question: TensorFlow or PyTorch?
G - AI - Episode - 3 - Lesson - 7 - Lets Install Python and integrate Pytorch
11 years later ❤️ @shrads
What Is ChatGPT Doing? Episode 3: Neural Networks for Human-Like Tasks
Building a Neural Network with PyTorch in 15 Minutes | Coding Challenge
G AI - Episode - 3 - Introduction to developing a basic generative adversarial network
PyTorch Lightning - Speed up model training with benchmark
Getting Started with 3LC | Part 1: Creating a Table
Episode 2: PyTorch Dropout, Batch size and interactive debugging
Part 3: Multi-GPU training with DDP (code walkthrough)
Jeremy Howard: Deep Learning Frameworks - TensorFlow, PyTorch, fast.ai | AI Podcast Clips
Boltus: God of AI | Episode 3
Cats&Dogs PT 3: Pytorch Custom Dataset
PyTorch Lightning - Auto select GPUs
PyTorch on GCP
Pytorch - Lesson 3 - #AIScience
Комментарии