filmov
tv
How to Check normality assumption in simple linear regression in SPSS
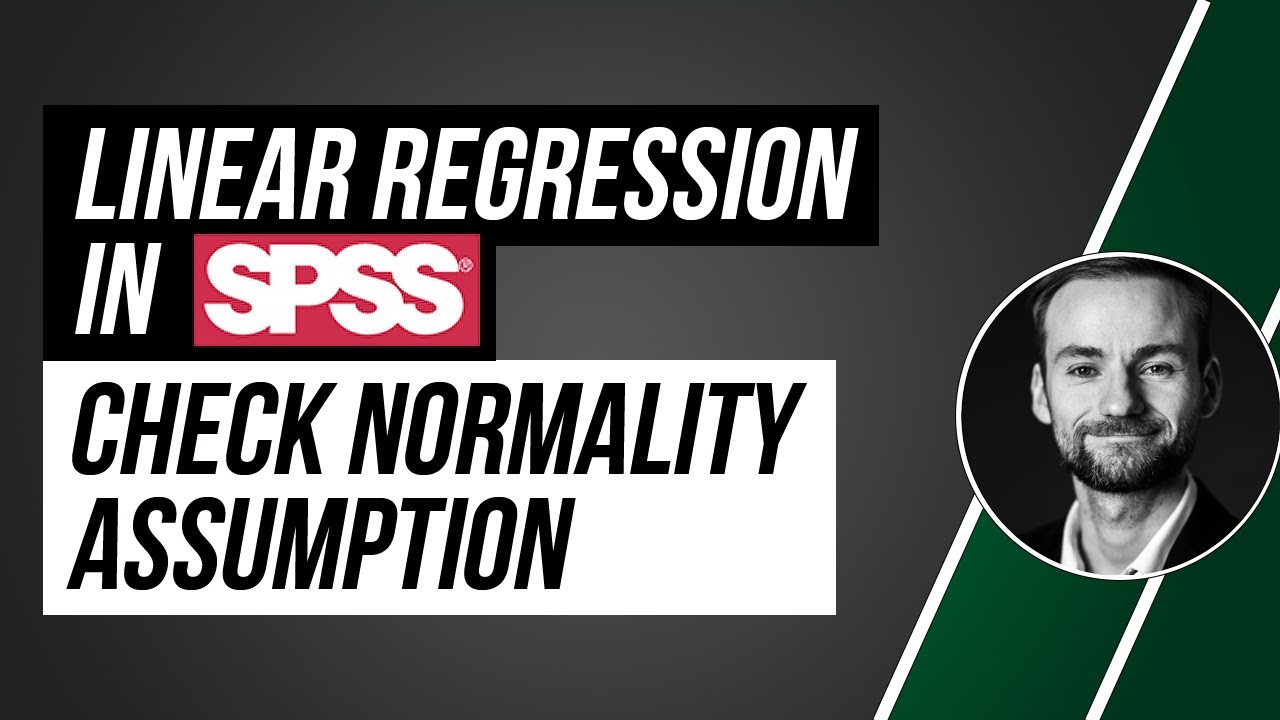
Показать описание
This tutorial shows how to test the residuals for normal distribution in a linear regression in SPSS.
Since residuals are the differences between the predicted and observed values of the dependent variable, the simple linear regression model within SPSS must be calculated first. I will use the standardized residuals. The show analyses work with undstandardized residuals as well.
Afterwards I will show three ways to check normality for the residuals in a simple linear regression in SPSS.
📋 3 ways to test for normality
=========================
1) Using the z-values of skewness and kurtosis (divide the respecive value by its standard error) and checking whether they exceed the critical values. Critical values are derived fomr the confidence levels (.05, .01 or .001) and range from 1.96, 2.58 to 3.29. Your z-values of skewness and kurtosis should be below the preselected confidence value and its critical value. Please be aware though that larger samples will automatically produce smaller standard errors and hence larger z-values, leading to unnecessary rejection of normal distribution of your residuals.
2) Using analytical tests like the Kolmogorov-Smirnov test or the Shapiro-Wilk-tests which have the null hypothesis of normal distribution that should not be rejected. It’s also important to note that as the sample size increases, even small, insignificant deviations from normality can lead to rejecting the null hypothesis. Not only does this increased sensitivity in large samples lead to unnecessary rejection of the null hypothesis, but both tests also lack power in small samples. In summary: both tests are unreliable and should not be used, which is repeatedly emphasized in the literature, for instance Field p. 248.
3) Using graphical testing with histograms or Q-Q-plots. However, a histogram can be manipulated quite easily, by changing the number of intervals and make it look more favourable. Because of the ease of manipulation, some reviewers reject histograms and prefer Q-Q plots. In a Q-Q plot, the line represents the perfect normal distribution, and the points represent the residuals. For perfectly normally distributed residuals, all points lie exactly on the line. Of course, this is not expected in reality, and the points lie more or less on or very close to the line to indicate normal distribution.
Sources:
=======
📚 Field, A. (2018), Discovering Statistics Using SPSS, p. 246-256.
⏰ Timestamps:
==============
0:00 Introduction and acquiring residuals
0:50 Prepare three methods of testing
1:17 Skewness and Kurtosis
2:22 Kolmogorov-Smirnov and Shapiro-Wilk tests
3:15 Histogram and Q-Q-plot
If you have any questions or suggestions regarding testing normality for the simple linear regression in SPSS, please use the comment function. Thumbs up or down to decide if you found the video helpful.
#statorials
Support channel? 🙌🏼
===================
Since residuals are the differences between the predicted and observed values of the dependent variable, the simple linear regression model within SPSS must be calculated first. I will use the standardized residuals. The show analyses work with undstandardized residuals as well.
Afterwards I will show three ways to check normality for the residuals in a simple linear regression in SPSS.
📋 3 ways to test for normality
=========================
1) Using the z-values of skewness and kurtosis (divide the respecive value by its standard error) and checking whether they exceed the critical values. Critical values are derived fomr the confidence levels (.05, .01 or .001) and range from 1.96, 2.58 to 3.29. Your z-values of skewness and kurtosis should be below the preselected confidence value and its critical value. Please be aware though that larger samples will automatically produce smaller standard errors and hence larger z-values, leading to unnecessary rejection of normal distribution of your residuals.
2) Using analytical tests like the Kolmogorov-Smirnov test or the Shapiro-Wilk-tests which have the null hypothesis of normal distribution that should not be rejected. It’s also important to note that as the sample size increases, even small, insignificant deviations from normality can lead to rejecting the null hypothesis. Not only does this increased sensitivity in large samples lead to unnecessary rejection of the null hypothesis, but both tests also lack power in small samples. In summary: both tests are unreliable and should not be used, which is repeatedly emphasized in the literature, for instance Field p. 248.
3) Using graphical testing with histograms or Q-Q-plots. However, a histogram can be manipulated quite easily, by changing the number of intervals and make it look more favourable. Because of the ease of manipulation, some reviewers reject histograms and prefer Q-Q plots. In a Q-Q plot, the line represents the perfect normal distribution, and the points represent the residuals. For perfectly normally distributed residuals, all points lie exactly on the line. Of course, this is not expected in reality, and the points lie more or less on or very close to the line to indicate normal distribution.
Sources:
=======
📚 Field, A. (2018), Discovering Statistics Using SPSS, p. 246-256.
⏰ Timestamps:
==============
0:00 Introduction and acquiring residuals
0:50 Prepare three methods of testing
1:17 Skewness and Kurtosis
2:22 Kolmogorov-Smirnov and Shapiro-Wilk tests
3:15 Histogram and Q-Q-plot
If you have any questions or suggestions regarding testing normality for the simple linear regression in SPSS, please use the comment function. Thumbs up or down to decide if you found the video helpful.
#statorials
Support channel? 🙌🏼
===================