filmov
tv
Finding scaling laws for Reinforcement Learning
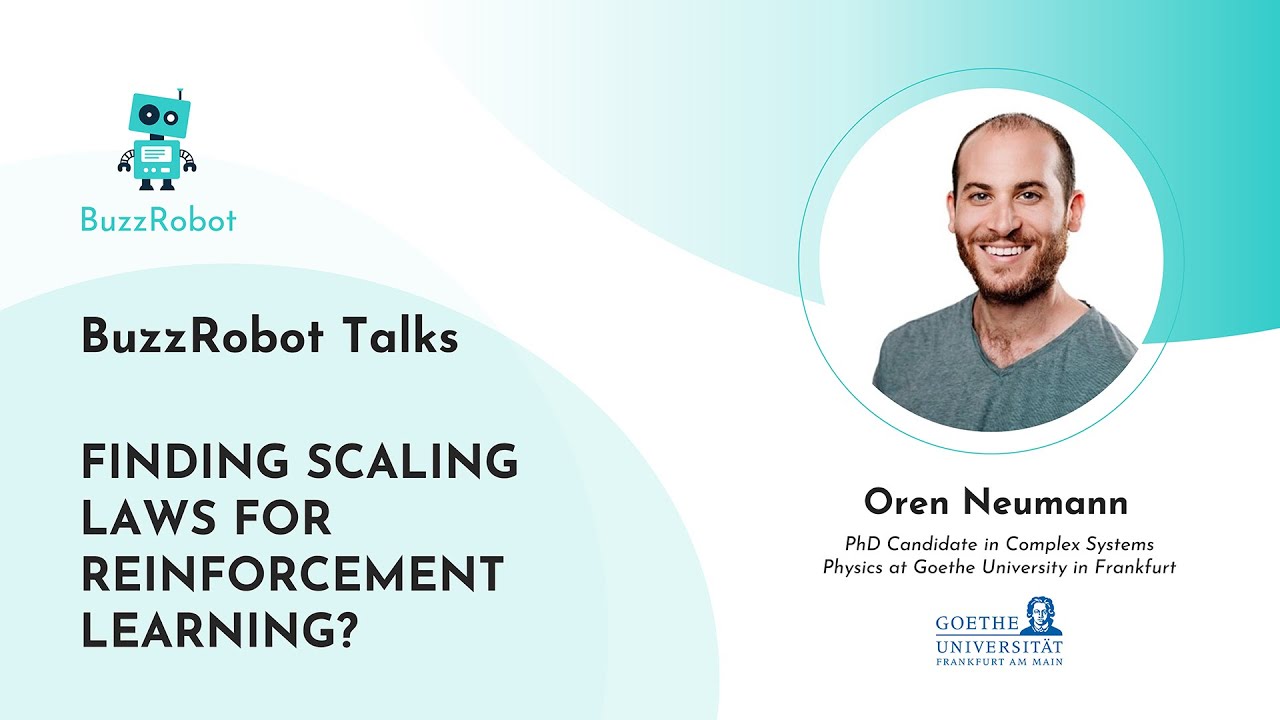
Показать описание
Neural scaling laws have received a lot of attention in recent ML research, ever since it was discovered that generative language models improve their performance as a power law of available resources. Since then, power-law scaling laws seem to pop up in every field and setting imaginable. These laws provide clear instructions on how to train large models on multi-million dollar budgets, and have directly guided the creation of SOTA models like GPT-3. Despite all this, Reinforcement Learning had until recently almost no record of power-law scaling.
In this talk, Oren Neumann shares how his team found power-law scaling laws for AlphaZero, why any previous attempt to find these laws in RL failed, and how to train a model efficiently when a single training attempt costs several million dollars.
Bio: Oren Neumann is PhD candidate in Complex Systems physics at Goethe University in Frankfurt. Coming from a background in Condensed Matter physics, where emergent power laws have been studied for decades, he focused his research on scaling phenomena in Reinforcement Learning.
In this talk, Oren Neumann shares how his team found power-law scaling laws for AlphaZero, why any previous attempt to find these laws in RL failed, and how to train a model efficiently when a single training attempt costs several million dollars.
Bio: Oren Neumann is PhD candidate in Complex Systems physics at Goethe University in Frankfurt. Coming from a background in Condensed Matter physics, where emergent power laws have been studied for decades, he focused his research on scaling phenomena in Reinforcement Learning.