filmov
tv
178 - An introduction to variational autoencoders (VAE)
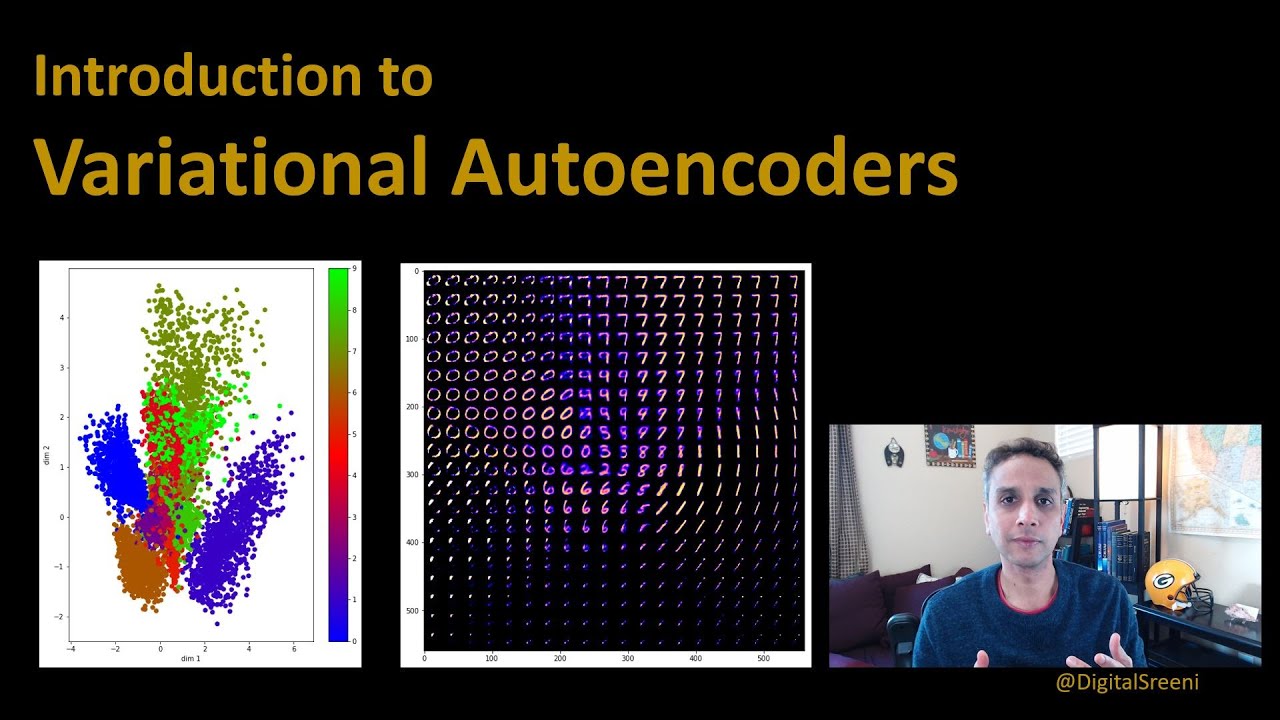
Показать описание
Code generated in the video can be downloaded from here:
178 - An introduction to variational autoencoders (VAE)
Episode 178: Introduction to the Income Statement
The Introduction of the Heinkel He 178: A Jet-Powered Marvel
Bruce Buffer Conor McGregor Introduction UFC 178 Vs Dustin Poirier
Có 178 cục bộ 1 kg #giabaojewelry #jewelry #trangsucbac #diamond
Introduction @178 // GABRIELZ
Introduction to Arithmetic Sequences
The Second Ring Beyond the ice walls: Map introduction and analysis (1)
178 Revelation, Introduction (2)
SCP Explained - A Modern Introduction to the SCP Foundation
CES: Introduction to Model Based Definition Using NX PMI
Virtual Geography 178 Introduction
autodesk revit 178 Introduction to Coordination
Sich VorstellenA1,A2#introduction#deutsch#learning#like#share#support#subscribe#video#yt#deutschland
CS101 slides #178|introduction to Computing | in Urdu/Hindi
Organ Sonata No. 8 in B Minor, Op. 178: III. Introduction - Passacaglia
Exploring the SCP Foundation: Introduction to the Foundation
A Brief Introduction to The Bahá’í Faith in Kikuyu By Thara wa Mundia Aka Mehraz Ehsani
:- Girls Introduction! #aesthetic #explore #shorts #viral
Long Division: A Step-By-Step Review | How to do Long Division | Math with Mr. J
Introduction to Selenium IDE interface
EASA Part 21 Subpart G Regulatory Framework Online Course Introduction - Sofema Online
Lec01 Introduction | CS178 SP23
Chemistry Lesson: Introduction to Measurements
Комментарии