filmov
tv
GPT-3: Language Models are Few-Shot Learners (Paper Explained)
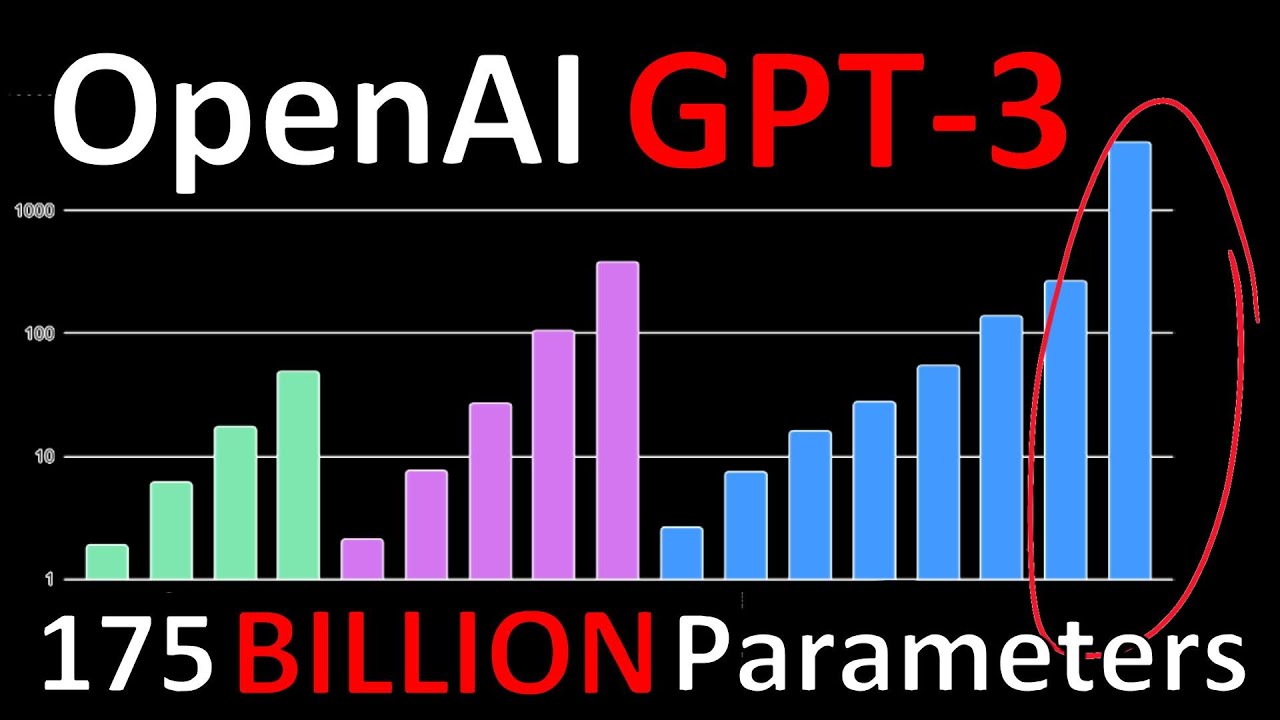
Показать описание
#gpt3 #openai #gpt-3
How far can you go with ONLY language modeling? Can a large enough language model perform NLP task out of the box? OpenAI take on these and other questions by training a transformer that is an order of magnitude larger than anything that has ever been built before and the results are astounding.
OUTLINE:
0:00 - Intro & Overview
1:20 - Language Models
2:45 - Language Modeling Datasets
3:20 - Model Size
5:35 - Transformer Models
7:25 - Fine Tuning
10:15 - In-Context Learning
17:15 - Start of Experimental Results
19:10 - Question Answering
23:10 - What I think is happening
28:50 - Translation
31:30 - Winograd Schemes
33:00 - Commonsense Reasoning
37:00 - Reading Comprehension
37:30 - SuperGLUE
40:40 - NLI
41:40 - Arithmetic Expressions
48:30 - Word Unscrambling
50:30 - SAT Analogies
52:10 - News Article Generation
58:10 - Made-up Words
1:01:10 - Training Set Contamination
1:03:10 - Task Examples
Abstract:
Recent work has demonstrated substantial gains on many NLP tasks and benchmarks by pre-training on a large corpus of text followed by fine-tuning on a specific task. While typically task-agnostic in architecture, this method still requires task-specific fine-tuning datasets of thousands or tens of thousands of examples. By contrast, humans can generally perform a new language task from only a few examples or from simple instructions - something which current NLP systems still largely struggle to do. Here we show that scaling up language models greatly improves task-agnostic, few-shot performance, sometimes even reaching competitiveness with prior state-of-the-art fine-tuning approaches. Specifically, we train GPT-3, an autoregressive language model with 175 billion parameters, 10x more than any previous non-sparse language model, and test its performance in the few-shot setting. For all tasks, GPT-3 is applied without any gradient updates or fine-tuning, with tasks and few-shot demonstrations specified purely via text interaction with the model. GPT-3 achieves strong performance on many NLP datasets, including translation, question-answering, and cloze tasks, as well as several tasks that require on-the-fly reasoning or domain adaptation, such as unscrambling words, using a novel word in a sentence, or performing 3-digit arithmetic. At the same time, we also identify some datasets where GPT-3's few-shot learning still struggles, as well as some datasets where GPT-3 faces methodological issues related to training on large web corpora. Finally, we find that GPT-3 can generate samples of news articles which human evaluators have difficulty distinguishing from articles written by humans. We discuss broader societal impacts of this finding and of GPT-3 in general.
Authors: Tom B. Brown, Benjamin Mann, Nick Ryder, Melanie Subbiah, Jared Kaplan, Prafulla Dhariwal, Arvind Neelakantan, Pranav Shyam, Girish Sastry, Amanda Askell, Sandhini Agarwal, Ariel Herbert-Voss, Gretchen Krueger, Tom Henighan, Rewon Child, Aditya Ramesh, Daniel M. Ziegler, Jeffrey Wu, Clemens Winter, Christopher Hesse, Mark Chen, Eric Sigler, Mateusz Litwin, Scott Gray, Benjamin Chess, Jack Clark, Christopher Berner, Sam McCandlish, Alec Radford, Ilya Sutskever, Dario Amodei
Links:
How far can you go with ONLY language modeling? Can a large enough language model perform NLP task out of the box? OpenAI take on these and other questions by training a transformer that is an order of magnitude larger than anything that has ever been built before and the results are astounding.
OUTLINE:
0:00 - Intro & Overview
1:20 - Language Models
2:45 - Language Modeling Datasets
3:20 - Model Size
5:35 - Transformer Models
7:25 - Fine Tuning
10:15 - In-Context Learning
17:15 - Start of Experimental Results
19:10 - Question Answering
23:10 - What I think is happening
28:50 - Translation
31:30 - Winograd Schemes
33:00 - Commonsense Reasoning
37:00 - Reading Comprehension
37:30 - SuperGLUE
40:40 - NLI
41:40 - Arithmetic Expressions
48:30 - Word Unscrambling
50:30 - SAT Analogies
52:10 - News Article Generation
58:10 - Made-up Words
1:01:10 - Training Set Contamination
1:03:10 - Task Examples
Abstract:
Recent work has demonstrated substantial gains on many NLP tasks and benchmarks by pre-training on a large corpus of text followed by fine-tuning on a specific task. While typically task-agnostic in architecture, this method still requires task-specific fine-tuning datasets of thousands or tens of thousands of examples. By contrast, humans can generally perform a new language task from only a few examples or from simple instructions - something which current NLP systems still largely struggle to do. Here we show that scaling up language models greatly improves task-agnostic, few-shot performance, sometimes even reaching competitiveness with prior state-of-the-art fine-tuning approaches. Specifically, we train GPT-3, an autoregressive language model with 175 billion parameters, 10x more than any previous non-sparse language model, and test its performance in the few-shot setting. For all tasks, GPT-3 is applied without any gradient updates or fine-tuning, with tasks and few-shot demonstrations specified purely via text interaction with the model. GPT-3 achieves strong performance on many NLP datasets, including translation, question-answering, and cloze tasks, as well as several tasks that require on-the-fly reasoning or domain adaptation, such as unscrambling words, using a novel word in a sentence, or performing 3-digit arithmetic. At the same time, we also identify some datasets where GPT-3's few-shot learning still struggles, as well as some datasets where GPT-3 faces methodological issues related to training on large web corpora. Finally, we find that GPT-3 can generate samples of news articles which human evaluators have difficulty distinguishing from articles written by humans. We discuss broader societal impacts of this finding and of GPT-3 in general.
Authors: Tom B. Brown, Benjamin Mann, Nick Ryder, Melanie Subbiah, Jared Kaplan, Prafulla Dhariwal, Arvind Neelakantan, Pranav Shyam, Girish Sastry, Amanda Askell, Sandhini Agarwal, Ariel Herbert-Voss, Gretchen Krueger, Tom Henighan, Rewon Child, Aditya Ramesh, Daniel M. Ziegler, Jeffrey Wu, Clemens Winter, Christopher Hesse, Mark Chen, Eric Sigler, Mateusz Litwin, Scott Gray, Benjamin Chess, Jack Clark, Christopher Berner, Sam McCandlish, Alec Radford, Ilya Sutskever, Dario Amodei
Links:
Комментарии