filmov
tv
Vision Transformer explained in detail | ViTs
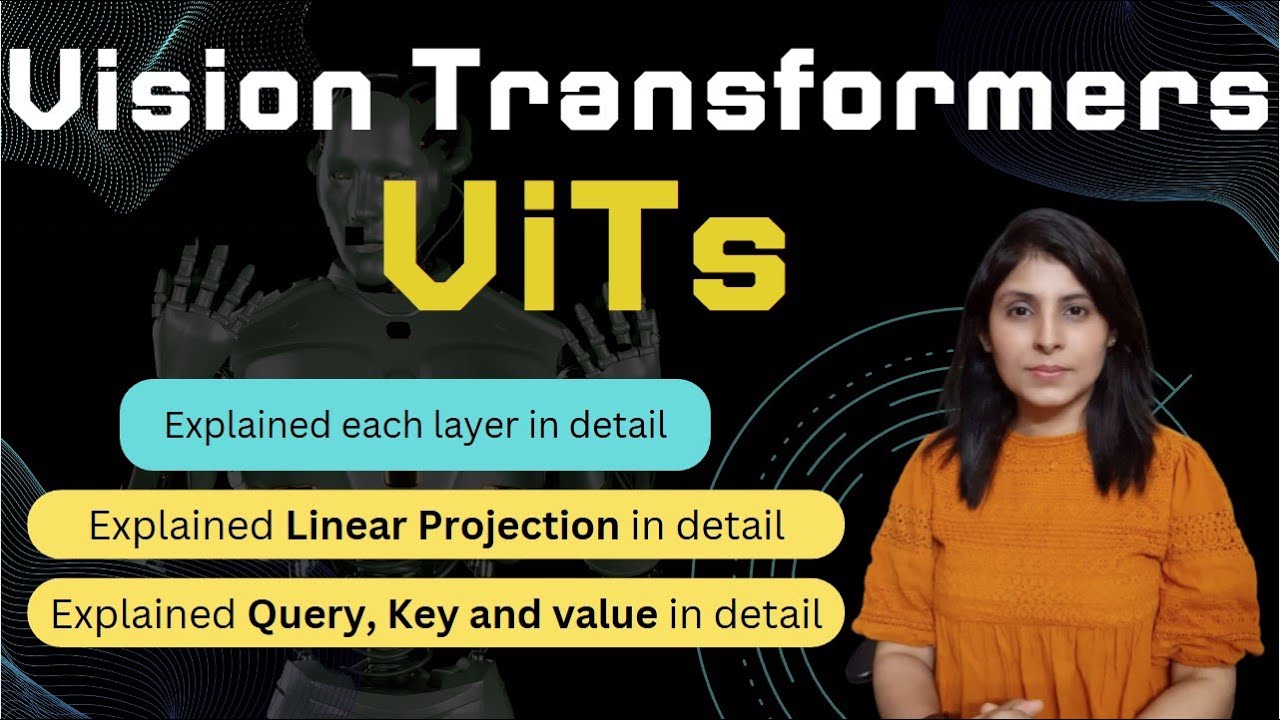
Показать описание
Understanding Vision Transformers: A Beginner-Friendly Guide:
In this video, I dive into Vision Transformers (ViTs) and break down the core concepts in a simple and easy-to-follow way. You’ll learn about:
Linear Projection: What it is and how it plays a role in transforming image patches.
Multihead Attention Layer: An explanation of query, key, and value, and how these components help the model focus on important information.
Key Concepts of Vision Transformers: From patch embedding to self-attention, you'll understand the basics and gain insight into how Vision Transformers work.
Whether you're new to transformers or looking to build a stronger foundation, this video is for you.
Make sure to like, subscribe, and comment if you found this helpful!
In this video, I dive into Vision Transformers (ViTs) and break down the core concepts in a simple and easy-to-follow way. You’ll learn about:
Linear Projection: What it is and how it plays a role in transforming image patches.
Multihead Attention Layer: An explanation of query, key, and value, and how these components help the model focus on important information.
Key Concepts of Vision Transformers: From patch embedding to self-attention, you'll understand the basics and gain insight into how Vision Transformers work.
Whether you're new to transformers or looking to build a stronger foundation, this video is for you.
Make sure to like, subscribe, and comment if you found this helpful!
Vision Transformer Quick Guide - Theory and Code in (almost) 15 min
An Image is Worth 16x16 Words: Transformers for Image Recognition at Scale (Paper Explained)
Vision Transformer explained in detail | ViTs
Vision Transformers explained
Vision Transformer Basics
Vision Transformer for Image Classification
An image is worth 16x16 words: ViT | Vision Transformer explained
Vision Transformers (ViT) Explained + Fine-tuning in Python
Vision Transformer and its Applications
Vision Transformer (ViT) - An image is worth 16x16 words | Paper Explained
The Vision Transformer Model (ViT)
PATCH EMBEDDING | Vision Transformers explained
Attention in transformers, visually explained | DL6
Illustrated Guide to Transformers Neural Network: A step by step explanation
DINO: Emerging Properties in Self-Supervised Vision Transformers (Facebook AI Research Explained)
Vision Transformer Explained
Transformers in Vision: From Zero to Hero
What are Transformers (Machine Learning Model)?
Transformer Neural Networks, ChatGPT's foundation, Clearly Explained!!!
CS 198-126: Lecture 15 - Vision Transformers
Vision Transformer Attention
ATTENTION | An Image is Worth 16x16 Words | Vision Transformers (ViT) Explanation and Implementation
EfficientML.ai Lecture 14 - Vision Transformer (MIT 6.5940, Fall 2023)
Transformers, explained: Understand the model behind GPT, BERT, and T5
Комментарии