filmov
tv
How Do Neural Networks Grow Smarter? - with Robin Hiesinger
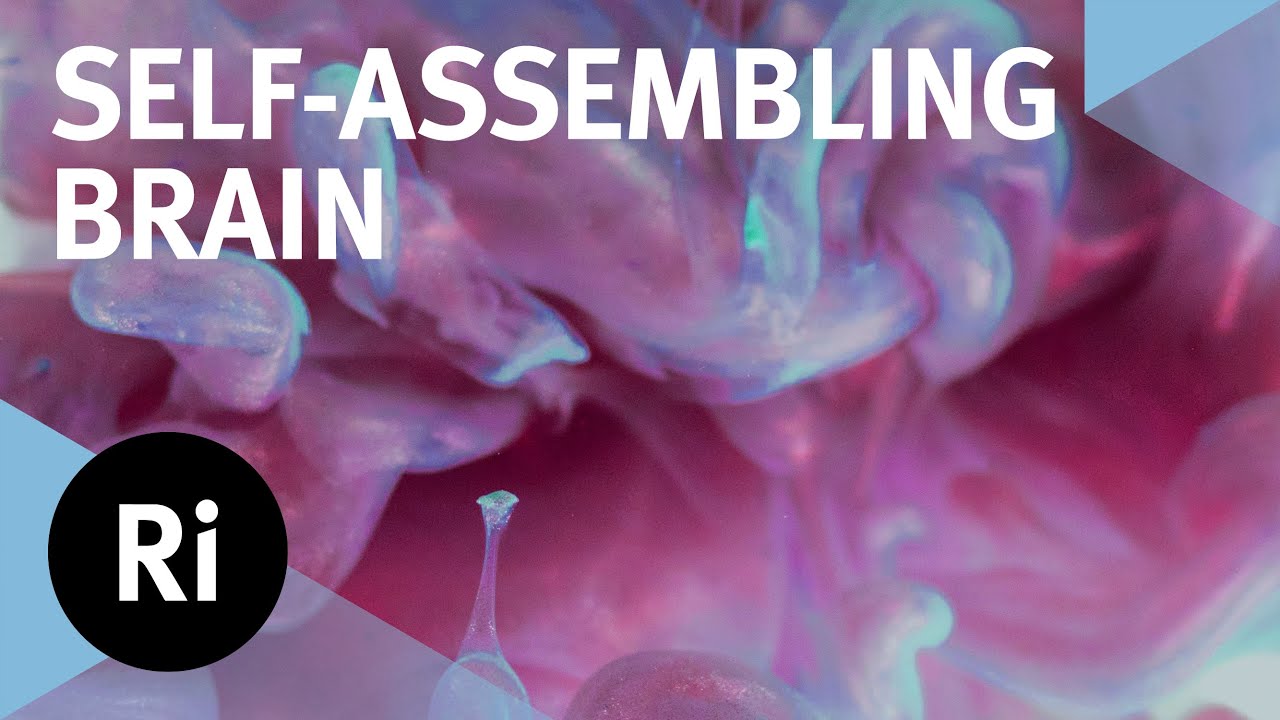
Показать описание
Neurobiologists and computer scientists are trying to discover how neural networks become a brain. Will nature give us the answer, or is it all up to an artificial intelligence to work it out?
Join Peter Robin Hiesinger as he explores if the biological brain is just messy hardware which scientists can improve upon by running learning algorithms on computers.
In this talk, Robin will discuss these intertwining topics from both perspectives, including the shared history of neurobiology and Artificial Intelligence.
Peter Robin Hiesinger is professor of neurobiology at the Institute for Biology, Freie Universität Berlin.
Robin did his undergraduate and graduate studies in genetics, computational biology and philosophy at the University of Freiburg in Germany. He then did his postdoc at Baylor College of Medicine in Houston and was Assistant Professor and Associate Professor with tenure for more than 8 years at UT Southwestern Medical Center in Dallas. After 15 years in Texas and a life with no fast food, no TV, no gun and no right to vote, he is currently bewildered by his new home, Berlin, Germany.
This talk was recorded on 20th April 2021
---
A very special thank you to our Patreon supporters who help make these videos happen, especially:
---
Product links on this page may be affiliate links which means it won't cost you any extra but we may earn a small commission if you decide to purchase through the link.
Join Peter Robin Hiesinger as he explores if the biological brain is just messy hardware which scientists can improve upon by running learning algorithms on computers.
In this talk, Robin will discuss these intertwining topics from both perspectives, including the shared history of neurobiology and Artificial Intelligence.
Peter Robin Hiesinger is professor of neurobiology at the Institute for Biology, Freie Universität Berlin.
Robin did his undergraduate and graduate studies in genetics, computational biology and philosophy at the University of Freiburg in Germany. He then did his postdoc at Baylor College of Medicine in Houston and was Assistant Professor and Associate Professor with tenure for more than 8 years at UT Southwestern Medical Center in Dallas. After 15 years in Texas and a life with no fast food, no TV, no gun and no right to vote, he is currently bewildered by his new home, Berlin, Germany.
This talk was recorded on 20th April 2021
---
A very special thank you to our Patreon supporters who help make these videos happen, especially:
---
Product links on this page may be affiliate links which means it won't cost you any extra but we may earn a small commission if you decide to purchase through the link.
Комментарии