filmov
tv
Rethinking Pre-training and Self-Training
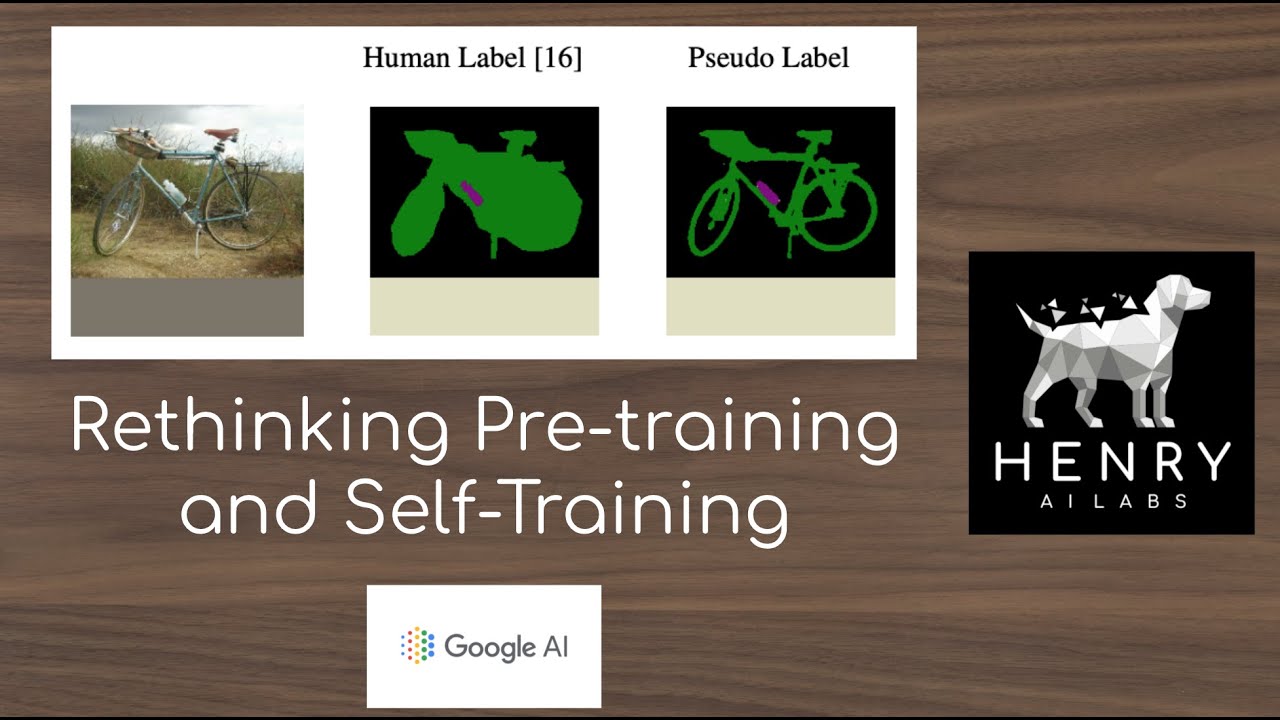
Показать описание
**ERRATA** at 9:31 I called the large scale jittering "color jittering", this isn't an operation specifically on colors.
This video explores an interesting paper from researchers at Google AI. They show that self-training outperforms supervised or self-supervised (SimCLR) pre-training. The video explains what self-training is and how all these methods attempt to utilize extra data (labeled or not) for better performance on downstream tasks.
Thanks for watching! Please Subscribe!
Paper Links:
This video explores an interesting paper from researchers at Google AI. They show that self-training outperforms supervised or self-supervised (SimCLR) pre-training. The video explains what self-training is and how all these methods attempt to utilize extra data (labeled or not) for better performance on downstream tasks.
Thanks for watching! Please Subscribe!
Paper Links:
Rethinking Pre-training and Self-Training
Harvard Medical AI: Arvind Saligrama on 'Rethinking Pre-training and Self-training'
Rethinking Pre training and Self training
Self-Training improves Pre-Training for Natural Language Understanding
[Paper review]Rethinking Pre training and Self training
Self-Training in Computer Vision
ImageGPT (Generative Pre-training from Pixels)
How Useful Is Self-Supervised Pretraining for Visual Tasks?
AdKDD 2020 On the Effectiveness of Self-supervised Pre-training for Modeling User Behavior Sequences
What is Pre-training a model?
Rethinking ImageNet Pretraining
Magical Way of Self-Training and Task Augmentation for NLP Models
Enhanced Direct Speech to Speech Translation with Self supervised Pre-training and Data Augmentation
[NeurIPS 2020] Rethinking the Value of Labels for Improving Class-Imbalanced Learning
STEAM plus Hybrid Training 1. Rethinking Lifelong Learning
Meetup #1 Rethinking ImageNet Pre-Training
Revisiting Self-Training for Neural Sequence Generation | NLP Journal Club
Rethinking ImageNet Pre-training @ TWiML Online Meetup
Research talk: Large-scale, self-supervised pretraining: From language to vision
Self-training Improves Pre-training for Natural Language Understanding
Rethinking Semantic Segmentation: A Prototype View | CVPR 2022
Full Stack Speech Processing with Wav LM: a Large-Scale Self-Supervised Pre-Training (Paper Summary)
[NeurIPS2022]10,000 times faster! (Rethinking and scaling up Graph contrastive learning)
Self-Supervised Learning for Object Detection in Autonomous Driving
Комментарии