filmov
tv
[MERL Seminar Series Spring 2022] Hybrid robotics and implicit learning
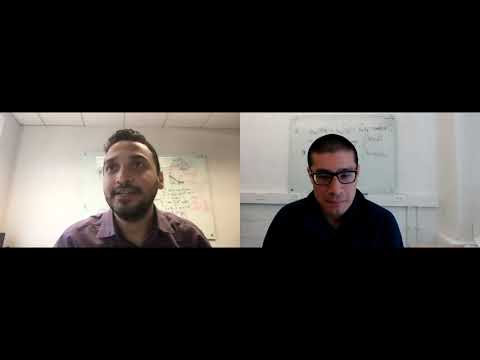
Показать описание
Michael Posa from U Pennsylvania, presented a talk in the MERL Seminar Series on May 3, 2022.
Abstract:
Machine learning has shown incredible promise in robotics, with some notable recent demonstrations in manipulation and sim2real transfer. These results, however, require either an accurate a priori model (for simulation) or a large amount of data. In contrast, my lab is focused on enabling robots to enter novel environments and then, with minimal time to gather information, accomplish complex tasks. In this talk, I will argue that the hybrid or contact-driven nature of real-world robotics, where a robot must safely and quickly interact with objects, drives this high data requirement. In particular, the inductive biases inherent in standard learning methods fundamentally clash with the non-differentiable physics of contact-rich robotics. Focusing on model learning, or system identification, I will show both empirical and theoretical results which demonstrate that contact stiffness leads to poor training and generalization, leading to some healthy skepticism of simulation experiments trained on artificially soft environments. Fortunately, implicit learning formulations, which embed convex optimization problems, can dramatically reshape the optimization landscape for these stiff problems. By carefully reasoning about the roles of stiffness and discontinuity, and integrating non-smooth structures, we demonstrate dramatically improved learning performance. Within this family of approaches, ContactNets accurately identifies the geometry and dynamics of a six-sided cube bouncing, sliding, and rolling across a surface from only a handful of sample trajectories. Similarly, a piecewise-affine hybrid system with thousands of modes can be identified purely from state transitions. Time permitting, I'll discuss how these learned models can be deployed for control via recent results in real-time, multi-contact MPC.
Abstract:
Machine learning has shown incredible promise in robotics, with some notable recent demonstrations in manipulation and sim2real transfer. These results, however, require either an accurate a priori model (for simulation) or a large amount of data. In contrast, my lab is focused on enabling robots to enter novel environments and then, with minimal time to gather information, accomplish complex tasks. In this talk, I will argue that the hybrid or contact-driven nature of real-world robotics, where a robot must safely and quickly interact with objects, drives this high data requirement. In particular, the inductive biases inherent in standard learning methods fundamentally clash with the non-differentiable physics of contact-rich robotics. Focusing on model learning, or system identification, I will show both empirical and theoretical results which demonstrate that contact stiffness leads to poor training and generalization, leading to some healthy skepticism of simulation experiments trained on artificially soft environments. Fortunately, implicit learning formulations, which embed convex optimization problems, can dramatically reshape the optimization landscape for these stiff problems. By carefully reasoning about the roles of stiffness and discontinuity, and integrating non-smooth structures, we demonstrate dramatically improved learning performance. Within this family of approaches, ContactNets accurately identifies the geometry and dynamics of a six-sided cube bouncing, sliding, and rolling across a surface from only a handful of sample trajectories. Similarly, a piecewise-affine hybrid system with thousands of modes can be identified purely from state transitions. Time permitting, I'll discuss how these learned models can be deployed for control via recent results in real-time, multi-contact MPC.