filmov
tv
Evidence Lower Bound (ELBO) - CLEARLY EXPLAINED!
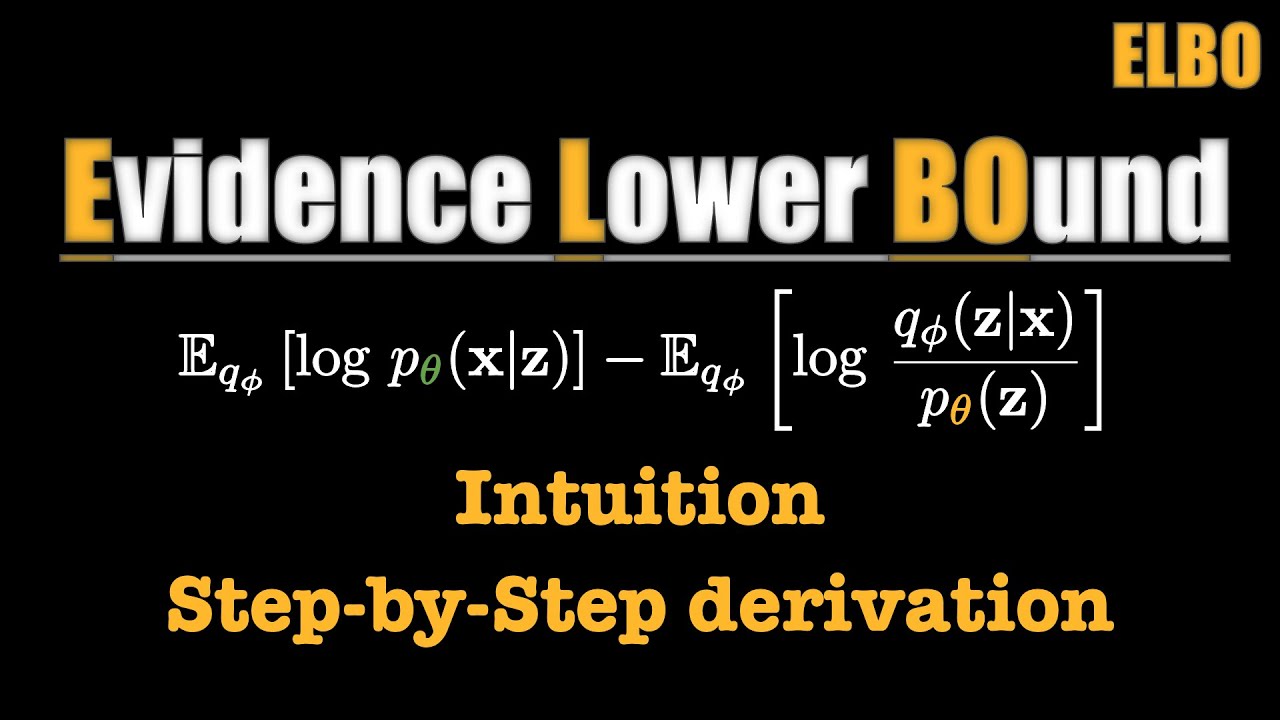
Показать описание
This tutorial explains what ELBO is and shows its derivation step by step.
#variationalinference
#kldivergence
#bayesianstatistics
#variationalinference
#kldivergence
#bayesianstatistics
Evidence Lower Bound (ELBO) - CLEARLY EXPLAINED!
Variational Inference | Evidence Lower Bound (ELBO) | Intuition & Visualization
Variational Autoencoder - Model, ELBO, loss function and maths explained easily!
VI - 4 - ELBO - Evidence Lower BOund
ELBO
Understanding Variational Autoencoders (VAEs) | Deep Learning
What is Evidence Lower Bound (ELBO) ?
Deep Learning Lecture 11.2 - Variational Inference
ELBO | Variational Inference
Variational Autoencoders
What is Evidence Lower Bound (ELBO) ?
Evidence Lower Bound (ELBO)
CS 285: Lecture 18, Variational Inference, Part 1
Deformed Evidence Lower Bound (λ-ELBO) using Rényi and Tsallis Divergence
The challenges in Variational Inference (+ visualization)
Variational Autoencoder - VISUALLY EXPLAINED!
Don't Blame The Elbo! 3 Minute Paper Summary (Neurips 2019)
Variational Inference by Automatic Differentiation in TensorFlow Probability
Variational Methods: How to Derive Inference for New Models (with Xanda Schofield)
CS 477 AI: Variational Autoencoders And ELBO: A Rigorous Derivation
Ali Ghodsi, Deep Learning, Variational Autoencoder, VAE, Performer, Fall 2023, Lecture 15
ELBO - The North star of AI Precision
The ELBO of Variational Autoencoders Converges to a Sum of Entropies (AISTATS 2023)
AI4OPT Seminar Series: Improving Variational Inference for Complex Probabilistic Modeling
Комментарии