filmov
tv
Why 'Grokking' AI Would Be A Key To AGI
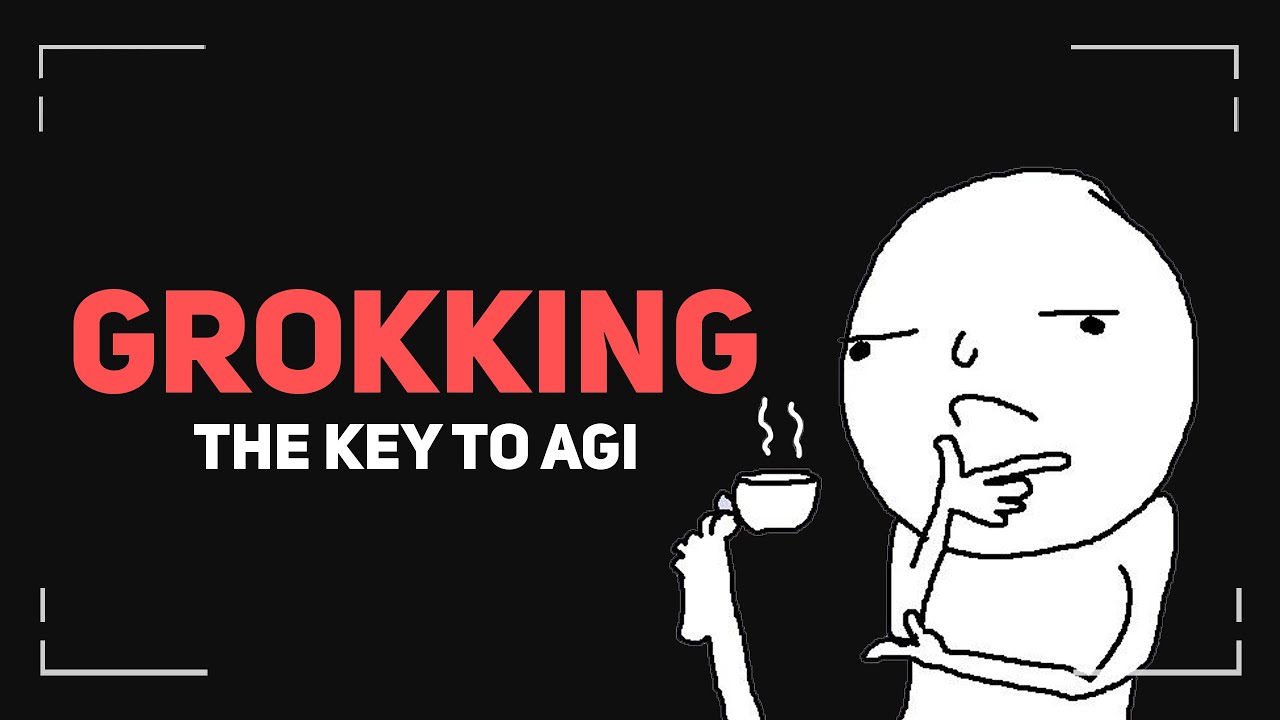
Показать описание
Check out my newsletter:
Are We Done With MMLU?
Alice in Wonderland: Simple Tasks Showing Complete Reasoning Breakdown in State-Of-the-Art Large Language Models
Grokked Transformers are Implicit Reasoners: A Mechanistic Journey to the Edge of Generalization
Grokfast: Accelerated Grokking by Amplifying Slow Gradients
This video is supported by the kind Patrons & YouTube Members:
🙏Andrew Lescelius, alex j, Chris LeDoux, Alex Maurice, Miguilim, Deagan, FiFaŁ, Robert Zawiasa, Daddy Wen, Tony Jimenez, Panther Modern, Jake Disco, Demilson Quintao, Shuhong Chen, Hongbo Men, happi nyuu nyaa, Carol Lo, Mose Sakashita, Miguel, Bandera, Gennaro Schiano, gunwoo, Ravid Freedman, Mert Seftali, Mrityunjay, Richárd Nagyfi, Timo Steiner, Henrik G Sundt, projectAnthony, Brigham Hall, Kyle Hudson, Kalila, Jef Come, Jvari Williams, Tien Tien, BIll Mangrum, owned, Janne Kytölä, SO, Richárd Nagyfi, Hector, Drexon, Claxvii 177th, Inferencer, Michael Brenner, Akkusativ, Oleg Wock, FantomBloth
[Music] massobeats - noon
[Video Editor] Silas
Комментарии