filmov
tv
Introduction to KL-Divergence | Simple Example | with usage in TensorFlow Probability
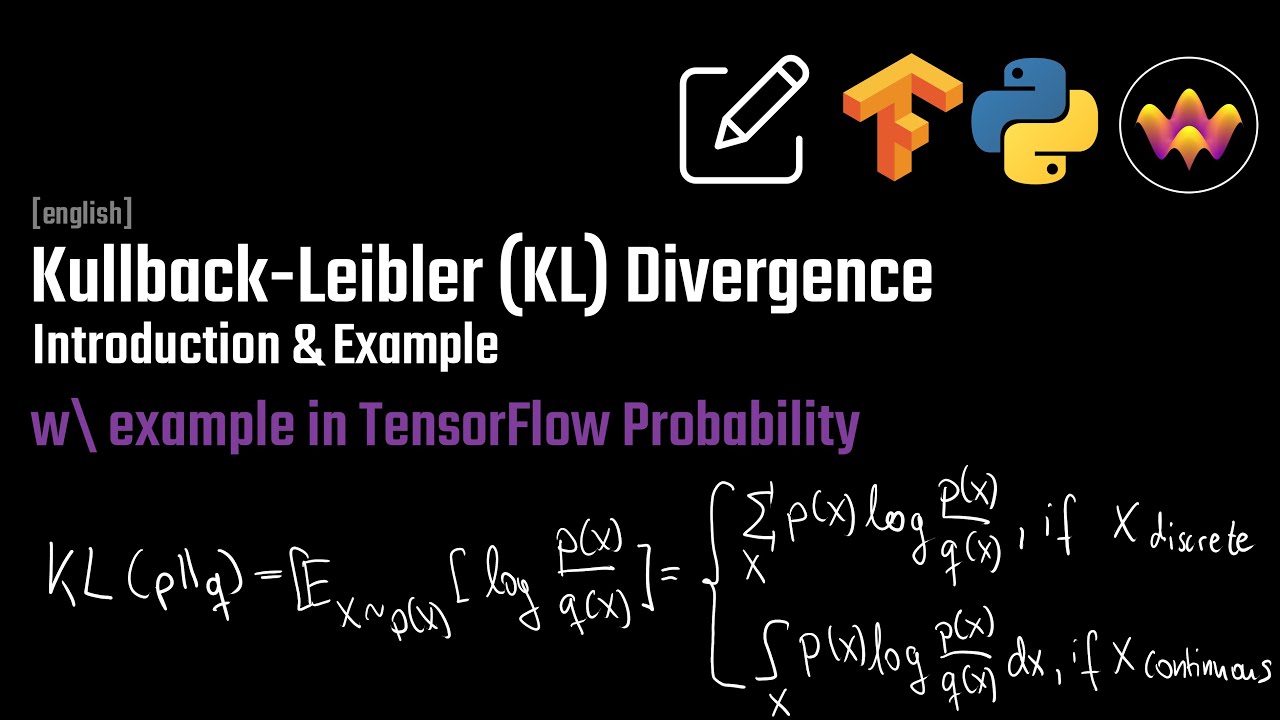
Показать описание
The KL-Divergence is especially relevant when we want to fit one distribution against another. It has multiple applications in Probabilistic Machine Learning and Statistics. In a later video, we will use it to derive Variational Inference, a powerful tool to fit surrogate posterior distributions.
-------
-------
Timestamps:
0:00 Opening
0:15 Intuition
03:21 Definition
05:28 Example
13:29 TensorFlow Probability
Intuitively Understanding the KL Divergence
Introduction to KL-Divergence | Simple Example | with usage in TensorFlow Probability
The KL Divergence : Data Science Basics
A Short Introduction to Entropy, Cross-Entropy and KL-Divergence
What is KL Divergence ?
Data Science Moments - Kullback-Leibler Divergence
Kullback-Leibler (KL) Divergence in Machine Learning | Data Science
KL Divergence - CLEARLY EXPLAINED!
#113 - KL Divergence
KL Divergence - Intuition and Math Clearly Explained
Kullback-Leibler (KL) Divergence Mathematics Explained
Kullback – Leibler divergence
20 - Properties of KL divergence
Kullback-Leibler Divergence (KL Divergence) Part-1
Entropy | Cross Entropy | KL Divergence | Quick Explained
5.7 The KL Divergence
KL (Kullback-Leibler) Divergence (Part 4/4): Jensen's Inequality and Why is KLD always positive...
Kullback Leibler Divergence || Machine Learning || Statistics
MaDL - Kullback-Leibler Divergence
Cross Entropy, Binary Cross Entropy and KL Divergence | Beginner Explanation
MLfAS - 08 Variational Autoencoder - 02 Kullback–Leibler Divergence
Kullback-Leibler (KL) Divergence | Data Science Interview Questions | Machine Learning
Approximating the KL-Divergence | Two Ways in TensorFlow Probability
Part 5: Kullback–Leibler discriminant analysis
Комментарии