filmov
tv
'Machine Learning for Proteins' by Lucy Colwell
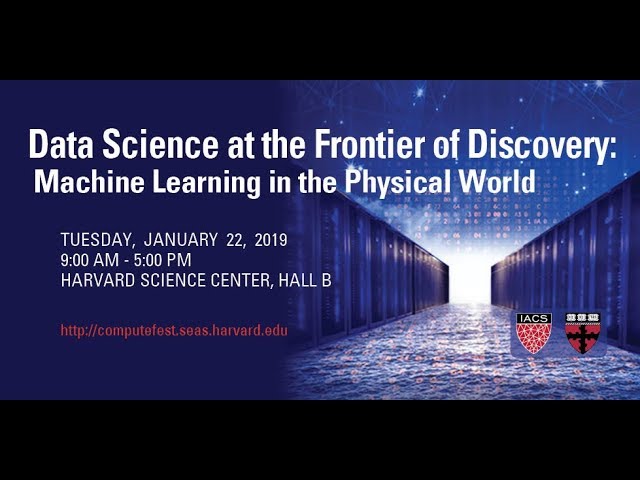
Показать описание
*** This talk is part of IACS's 2019 symposium on the Future of Computation: "Data Science at the Frontier of Discovery: Machine Learning in the Physical World" ***
Presenter: Lucy Colwell, Department of Chemistry, University of Cambridge
Presenter: Lucy Colwell, Department of Chemistry, University of Cambridge
'Machine Learning for Proteins' by Lucy Colwell
Machine learning methods for peptide, protein and antibody design
Machine Learning-Based Design Of Proteins
Andrew W. Ferguson, 'Machine learning-guided directed evolution of functional proteins'
Protein function prediction using neural networks | Google Developers ML Summit 2019
All-by-all learning of protein complexes from mass spectrometry data; SciPy 2013 Presentation
Nazim Bouatta | Machine learning for protein structure prediction, Part 1: Algorithm space
Robust deep learning based protein sequence design using ProteinMPNN
Python Machine Learning - Early Detection of Alzheimer’s Disease - ClickMyProject
Machine Learning-based Design of Proteins and Small Molecules
How to build a protein structure prediction app in Python using ESMFold and Streamlit
Machine Learning for Drug Discovery (Explained in 2 minutes)
Deep Neural Networks for Interpreting RNA-binding Protein Target... - Mahsa Ghanbari - ISCBacademy
Session 1: Algorithm development and machine learning approaches in genomics
Machine Learning-based Design of Proteins (Jennifer Listgarten, UC Berkeley)
Deploying protein machine learning models on the web
CSC2547 Fast end-to-end learning on protein surfaces
Machine learning methods for protein sorting prediction | Henrik Nielsen | Лекториум
PROTEIN_GENERATOR: Unleashing AI for Protein Design
Deep Learning for Protein Engineering Primer (AlphaFold 2, ProteinMPNN, RFDiffusion)
Hands-on on Protein Function Prediction with Machine Learning and Interactive Analytics
Proteins as language: NLP, Machine Learning & Protein... - Dan Ofer - MLCSB - Poster - ISMB 2022
Deep Learning in Structural Biology and Protein Design: How, Where, and Why
Machine Learning-Assisted Protein Engineering with ftMLDE and evSeq
Комментарии