filmov
tv
Curve Fitting for Understanding Michael Levitt 14May2020
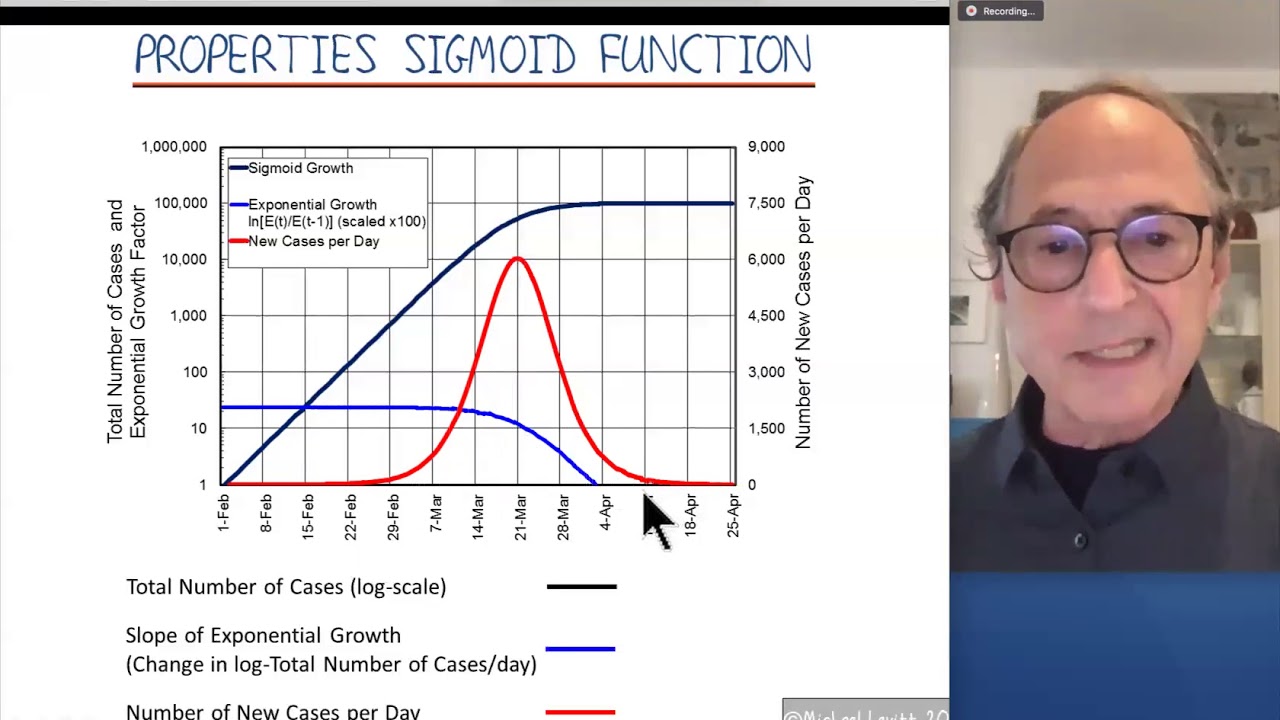
Показать описание
This is Part 2. Fitting viral growth data with simple mathematical functions can give important insights into how epidemics will grow. Here we illustrate two commonly used growth curves, the Sigmoid Function and the Gompertz Function. While superficially similar, they are really very different.
Curve Fitting for Understanding Michael Levitt 14May2020
M Levitt explains curve fitting using real data
Video Tutorial on Curve Fitting
Video Tutorial on Uncertainty of Parameters for Curve Fitting
Trig: 5.7: Curve Fitting
M Levitt #2 Concepts of curve fitting
Algebra II:1.4 Curve Fitting With Linear Models
Algebra II: 5.7 Curve Fitting With Quadratic Functions
Review of Curve Fitting in Python
Algebra II:2.8 Curve Fitting With Quadratic Models
curve fitting English
Excel - Fitting Data to Curve
Fitting Experimental Data and Linearization
Fitting Bell Curves to Data Distributions using Visualization - Fast Forward | VIS 2023
MTH 111 Linear Curve Fitting 4 3
Physics 111 CurveFitting with Google Sheets
Parabola of best fit using desmos
Estimating Partial Properties from Data & Ideal Gas Mixtures (Sept. 22, 2017)
116: Building entries without curvefitting [AUDIO ONLY]
Instantly Fix Lower Back Pain #Shorts
18th Jan reducing non-linear laws to linear form
Curve Fitting, Filleting
1.4: Curve fitting using pylab: Spring problem
Lesson 3.1, 2024 STEMNetX Data Science Workshop
Комментарии