filmov
tv
An Introduction to the ImageNet Dataset
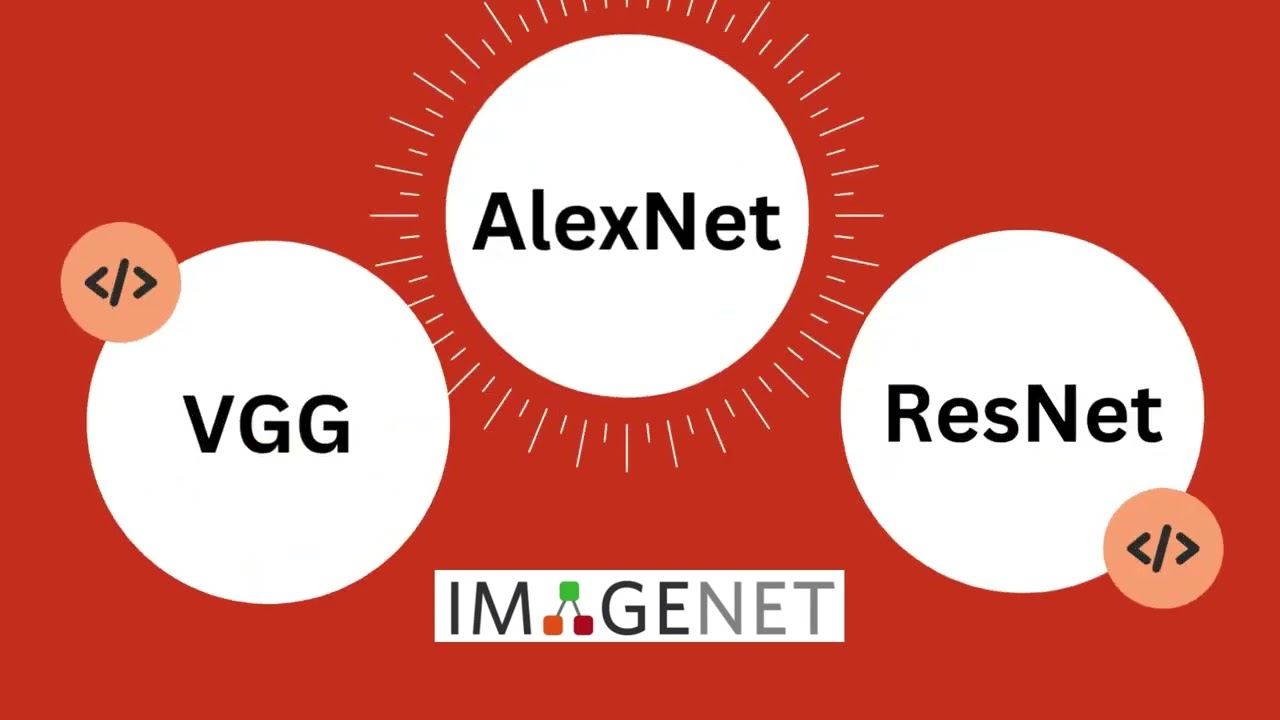
Показать описание
In this OER, we introduce the significance of ImageNet in the field of computer vision.
We start by explaining how the massive dataset is structured, discussing its hierarchical
organization and how the images are being visualized. We also explore the advantages
that such a massive dataset provides and how it has played a pivotal role in advancing the
capabilities of image classification models, particularly Convolutional Neural Networks
(CNNs). Moreover, we also discuss the challenges and limitations that it poses, including
their bias, diversity and semantic gap. Finally, we also discuss the concept of transfer
learning and how ImageNet is used for pre-training models that can be adapted to perform
different downstream tasks with minimal modifications.
ImageNet references for the video:
Deng, J. et al. (2009) ‘ImageNet: A large-scale hierarchical image database’, 2009 IEEE
Conference on Computer Vision and Pattern Recognition [Preprint].
doi:10.1109/cvpr.2009.5206848.
Iofinova, E. et al. (2022) ‘How well do sparse ImageNet models transfer?’, 2022
IEEE/CVF Conference on Computer Vision and Pattern Recognition (CVPR)
[Preprint]. doi:10.1109/cvpr52688.2022.01195.
Luccioni, A.S. and Rolnick, D. (2023) ‘Bugs in the data: How imagenet misrepresents
biodiversity’, Proceedings of the AAAI Conference on Artificial Intelligence,
37(12), pp. 14382–14390. doi:10.1609/aaai.v37i12.26682.
Nielsen, F.Å. (2018) ‘Linking ImageNet WordNet synsets with Wikidata’, Companion
of the The Web Conference 2018 on The Web Conference 2018 - WWW ’18
[Preprint]. doi:10.1145/3184558.3191645.
Russakovsky, O. et al. (2015) ‘Imagenet Large Scale Visual Recognition Challenge’,
International Journal of Computer Vision, 115(3), pp. 211–252.
doi:10.1007/s11263-015-0816-y.
Yang, K. et al. (2021) A Study of Face Obfuscation in ImageNet [Preprint].
We start by explaining how the massive dataset is structured, discussing its hierarchical
organization and how the images are being visualized. We also explore the advantages
that such a massive dataset provides and how it has played a pivotal role in advancing the
capabilities of image classification models, particularly Convolutional Neural Networks
(CNNs). Moreover, we also discuss the challenges and limitations that it poses, including
their bias, diversity and semantic gap. Finally, we also discuss the concept of transfer
learning and how ImageNet is used for pre-training models that can be adapted to perform
different downstream tasks with minimal modifications.
ImageNet references for the video:
Deng, J. et al. (2009) ‘ImageNet: A large-scale hierarchical image database’, 2009 IEEE
Conference on Computer Vision and Pattern Recognition [Preprint].
doi:10.1109/cvpr.2009.5206848.
Iofinova, E. et al. (2022) ‘How well do sparse ImageNet models transfer?’, 2022
IEEE/CVF Conference on Computer Vision and Pattern Recognition (CVPR)
[Preprint]. doi:10.1109/cvpr52688.2022.01195.
Luccioni, A.S. and Rolnick, D. (2023) ‘Bugs in the data: How imagenet misrepresents
biodiversity’, Proceedings of the AAAI Conference on Artificial Intelligence,
37(12), pp. 14382–14390. doi:10.1609/aaai.v37i12.26682.
Nielsen, F.Å. (2018) ‘Linking ImageNet WordNet synsets with Wikidata’, Companion
of the The Web Conference 2018 on The Web Conference 2018 - WWW ’18
[Preprint]. doi:10.1145/3184558.3191645.
Russakovsky, O. et al. (2015) ‘Imagenet Large Scale Visual Recognition Challenge’,
International Journal of Computer Vision, 115(3), pp. 211–252.
doi:10.1007/s11263-015-0816-y.
Yang, K. et al. (2021) A Study of Face Obfuscation in ImageNet [Preprint].